How to Deploy Your Data Science Team Across the Business
The crux of the problem in deploying a data science team is aligning the technical knowledge of a team to the needs of the business and choosing an alignment that offers the greatest impact.
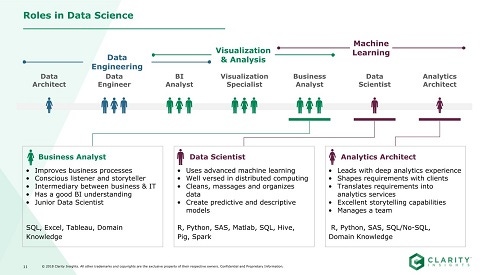
In the magical land of Silicon Valley, there is a legend about successful data science teams: They are made up of mythical creatures called “unicorns.” These data science unicorns hold advanced degrees in math and science, program in R/Python/Scala/Spark, deploy code on cloud platforms, put together compelling PowerPoints, and command rooms of executives.
There’s no denying there are exceptional data scientists, with both technical acumen and business expertise. In fact, some of us in this industry have worked with them. But the reality is no matter how good the data scientists are, their interaction with the business and IT sides of the company are critical. How can you get a team of qualified data scientists to understand the business and work with other teams effectively to focus on business outcomes, test hypothesis, etc.?
Breaking down the team
First, let’s quickly review the critical components that make up a best-in-class data science team. There are three components of successful analytics implementation: data, analysis, and production. Tied to these, there are three major elements for executing effective data science for the benefit of the business: data engineering, visualization, and analytics/machine learning.
Each of these capabilities needs a resource dedicated to those individual tasks:
● Machine Learning Specialist: expert grasp of machine learning and artificial intelligence algorithms, modeling process and distributed computing
● Business/Data Analyst: gathers requirements and creates business-friendly data visualizations that tell the data story
● Data Engineer: acquires and prepares data for the business analyst and ML specialist, deploys machine learning models on cloud platforms
To complete the team, you need a leader who leads with business strategy in mind: The Analytics Architect. He or she is someone who understands how the teams interact together and focuses on creating cohesive work, stakeholder communication and driving data-informed decisions. The architect should be able to “think like a data scientist” and know how best to collaborate with data engineers and analysts to uncover the customer, product, service, and operational insights that will drive business success.
Full team integration and deployment
Once the data science team is assembled, the business will thrive if the team is integrated into and across other departments. There are several ways to deploy your data experts in ML, analysis, engineering, and architecture:
● Offer a central team: In this deployment method, the data science team works together in one physical office, fielding requests that come in from the different business units. This approach works well for large companies that want to control the quality and priority of tickets. It also allows data scientists to have consistent interaction with each other, sharing methodologies and information. The downside to this method is that certain business units’ requests might get deprioritized over others, causing internal company resentment.
● Spread out analysts within each business team: This method allows each facet of the business to find and hire their own data scientists. This ensures that the data scientists learn that business area more deeply, truly understanding its needs and pain points. However, he/she might not be working with others who understand the purpose of the data scientist or the technologies used. He/she might be misunderstood at times and feel at loss without the benefit of talking regularly to other technical-minded people.
● Borrowed resources: Allow the data science team members to be “checked out” as a resource. They are on one central team, but business units can borrow them together or individually, as needed, to help right set the business goals. Policies should be put in place regarding need definition and how long the data scientist’s “tour of duty” can be.
Data science is a discipline that is constantly evolving. Data scientists today not only require skills in math, science and programming, but Cloud, containerization, serverless architectures, and microservices. The crux to the data science problem is aligning the technical knowledge of a team to the needs of the business and choosing an alignment that will offer the greatest impact. Ensure that your data science teams, or an experienced consultancy, recognize these needs and lay out a detailed plan to achieve your business goals.
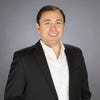
Gabriel Mohanna is director of data science and Mike Shaw is managing director of data engineering at Clarity Insights.
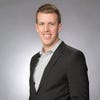
Clarity Insights is a strategic partner for the nation's leading organizations, providing data and analytics consulting services and enabling data-driven culture changes. With a team steeped in technical expertise and business acumen, Clarity Insights co-creates self-learning, sustainable cultures with clients. Working across the full ecosystem of data, from data science to strategy, they ensure data is a powerful competitive edge across industries, including healthcare, financial services, insurance and others.
About the Author(s)
You May Also Like