3 Things Execs Should Know About Machine Learning
Implementing machine learning isn't just about the technology; it requires organizational changes too.
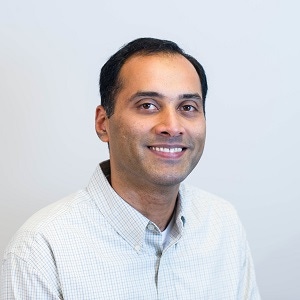
Machine learning and artificial intelligence are technologies that have evolved rapidly in the last decade. Most people today are familiar with intelligent voice assistants, streaming video platforms that recommend personalized content, and vehicle navigation systems that suggest best routes in real time to avoid traffic, all examples of artificial intelligence and machine learning in the consumer world.
However, the path to machine learning isn’t quite so clear on the enterprise side. While consumer-facing machine learning often has a narrow focus, enterprise machine learning solutions must cater to many different types of businesses, all of which measure success differently. A consumer can expect Netflix to learn her movie preferences by tracking what she and people similar to her click on, a solution that can be built to be fairly generic. However, enterprise machine learning solutions rarely work as seamlessly right out of the box. A leader looking to implement a machine learning solution must devote serious thought to how it can provide value to their business.
Here are a few business issues that leaders should be aware of when overseeing a machine learning implementation:
1. Your data may not be ready for machine learning. Business leaders have heard about the power of machine learning and they want to take advantage of it. However, their underlying data may not lend itself to machine learning immediately. While most organizations today collect enormous amounts of data, very little of it is organized in a way that lets it be immediately ingested by a machine learning algorithm. One late 2017 survey found that 51% of CIOs cited data quality as a barrier to machine learning adoption at their companies. Data may also be siloed within different departments, which is an organizational issue too. Per McKinsey, departments can often “hoard information and politicize access to it,” creating another hurdle to a successful organization-wide machine learning implementation.
For these reasons, it often makes the most sense to pilot a company’s first machine learning implementation in one or two business areas where data practices are robust. This focused approach avoids the challenges mentioned above and makes it easier to show strong results. After generating buy-in based on that initial success, business leaders can thenbegin the hard work of rolling out a machine learning implementation organization-wide.
2. No one size fits all. There has been a slew of companies lately claiming to provide out-of-the-box machine learning solutions that solve specific use cases. Some analysts believe such solutions will soon make machine learning so easy that most data scientist jobs will soon be obsolete. For instance, Gartner predicts that 40% of data science tasks will be automated by 2020. But while it’s true that technological refinements are making machine learning easier to implement, we’re still very far from the day when it’s as easy as clicking a button. Today, no machine learning solutions are truly “one size fits all,” as many software applications would be. To be really impactful, they must be tailored to each organization’s business needs, taking into account differences in business processes, workflows, enterprise systems, and company priorities.
That’s because the key to a successful machine learning implementation isn’t just adopting new technology, it’s changing the way the organization does business. It’s worth the time, expense, and effort required to build a custom solution that will surface machine learning-driven insights in existing workflows, putting the power of predictive analytics at managers’ and employees’ fingertips.
3. Engaging stakeholders is key to successful implementation. Everyone at the business ought to be involved in a machine learning implementation from the ground up. Effective communication is key: Employees might not be aware of what machine learning can and can’t do. Since machine learning informs human judgement, often improving employees’ work experiences, it’s important for leaders to help everyone at the company understand how to best leverage its capabilities.
Leaders need to convey how machine learning implementation doesn’t only help them, but everyone at the company. They need to be able to express that machine learning will take over many repetitive, mindless tasks at the company, allowing employees to do more value added work. For example, automated document extraction via machine learning can help employees spend more time on analyzing and taking action instead of manually entering data. It could also make customer support more painless, by allowing a machine learning program to serve as a chatbot that can respond to common questions, freeing up support agents to focus on more complex customer questions.
Though out-of-the-box solutions might seem tempting, it’s worth investing time and effort to understand what areas of the business are good candidates for machine learning and then outline a proper implementation plan that covers data, technology, and people. A focused pilot that solves a specific pain point or provides immediate ROI is a great starting point. By engaging all stakeholders through this process, a business leader can lay the groundwork for a successful rollout—and begin to harness the power of predictive analytics for their entire organization.
Girish Reddy is co-founder and CTO of SpringML, a predictive analytics provider focused on data-intensive industries. He was previously director of data integrations at Workday.
About the Author
You May Also Like