4 Principles of Developing an Ethical AI Strategy
How prioritizing responsible artificial intelligence will set your company up for success -- and instill trust in your customers
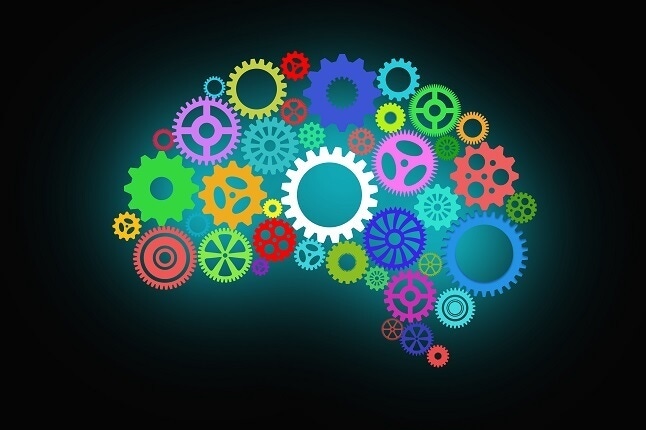
Artificial intelligence is the future, but it already has a prominent standing in the present. As data science gets more sophisticated and consumers continue to demand a more personalized customer experience, AI is the tool that will help enterprises better understand their customers and audiences. But even though AI has all the potential in the world, if we cannot figure out how to address the ethical challenges that remain, this full potential may never be reached.
As this technology evolves, one question should remain in the minds of all leaders seeking to implement an AI strategy: How can I ethically and responsibly make the most of AI within my organization?
In order to implement and scale AI capabilities that result in a positive return on investment (ROI) while minimizing risk, mitigating biases, and driving speed to value with your AI, enterprises should follow these four principles:
1. Understand goals, objectives, and risks
About seven years ago, Gartner released what they referred to as the “Hype Cycle for Emerging Technologies,” which highlighted the technologies it predicted would change society and business over the next decade. Among these technologies was AI.
The release of this report sent companies into a scramble to prove to analysts and investors that they were AI savvy -- and many began to implement AI strategies into their business models. However, at times, these strategies proved to be poorly executed and tacked on as an afterthought on top of existing analytics or digital objectives. This is because organizations did not have a clear understanding of the business problem that they were looking for AI to solve.
Only 10% of AI and ML models developed by enterprises are implemented. The historic disconnect between organizations with a problem and the data scientists who can use AI to solve that problem has left AI lagging. However, as data maturity has increased, organizations have begun to integrate data translators into different value chains -- such as marketing -- to uncover and translate business desires for outcomes.
This is why the first principle of developing an ethical AI strategy is to understand all goals, objectives, and risks, and then to create a decentralized approach to AI within your organization.
2. Do no harm
There have been many public examples of harmful AI. Organizations large and small have been left with damaged reputations and distrusting customers because they never properly developed their AI solutions to address issues of bias.
Organizations looking to create AI models must take preemptive measures to ensure their solutions do no harm. The way to do this: have a framework in place to prevent any negative impacts on algorithm predictions.
For example, if a company was looking to better understand the sentiment from customers through surveys, such as how underrepresented communities view their services, they may use data science to analyze these customer surveys and recognize that a percentage of surveys issued were being returned with responses in a non-English language, the only language the AI algorithm might understand.
To solve this issue, data scientists could go beyond modifying the algorithm to incorporate the intricate nuances of language. If these linguistic nuances were interpreted, and the AI was combined with a stronger fluency of language to make those conclusions more viable, the organization would be able to understand the needs of underrepresented communities to improve their customer experience.
3. Develop underlying data that is all-encompassing
AI algorithms are capable of analyzing vast datasets -- and enterprises should prioritize the development of a framework for the standards of data being used and ingested by their AI models. In order to successfully implement AI, a holistic, transparent, and traceable data set is essential.
Oftentimes, AI must account for human interference. Consider slang, abbreviations, code words, and more that humans develop on an evolving basis -- each of which has the ability to trip up a highly technical AI algorithm. AI models that are not equipped to process these human nuances ultimately lack a holistic dataset. Much like trying to drive with no mirrors, you have some of the information you need, but are missing key blind spots.
Organizations must find the balance between historical data and human interference to allow their AI models to learn these complex distinctions. By combining the structured data with unstructured data and training your AI to recognize both, a more holistic dataset can be generated and increase the accuracy of predictions.
To take it one step further, third-party audits of datasets can be an added bonus free of bias and discrepancies.
4. Avoid a black-box approach to algorithm development
For AI to be ethical, full transparency is required. In order to develop an AI strategy that is simultaneously transparent, interpretable, and explainable, enterprises must open the ‘black box’ of code to understand how each node or gate in the algorithm draws conclusions and interprets results.
While this may sound straightforward, to deliver on this requires a robust technical framework that can explain model and algorithm behaviors by reviewing the underlying code to show the different sub predictions being generated.
Enterprises can rely on open-sourced frameworks to assess AI and ML models across a variety of dimensions, including:
Feature analysis to assess the impact of applying new features to an existing model
Node analysis to explain a subset of predictions
Local analysis to explain individual predictions and matching features that can enhance results
Global analysis to provide a top-down review of the overall model behaviors and top features
Artificial intelligence is a complex technology -- with many potential pitfalls if organizations are not careful. A successful AI model is one that prioritizes ethics from day one, not as an afterthought. Across industries and organizations, AI is not a one-size fits all, but one common denominator that should break through is a commitment to transparent and unbiased predictions.
About the Author
You May Also Like