5 Ways to Embrace Next-Generation AI
Artificial intelligence represents an exciting new frontier in technology. How do companies decide how to begin leveraging next-generation solutions that will result in tangible value?
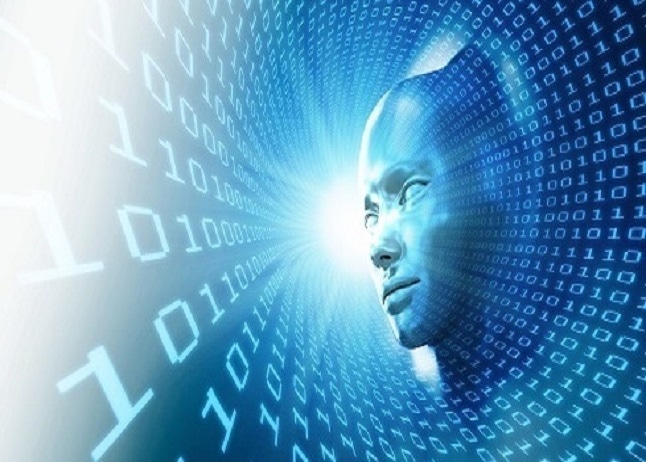
Experts weighed in on leading with next-generation AI during a keynote panel at research and advisory company Forrester’s Data & Strategy event this week.
How is AI at work today? Michael Finnerty SVP, marketing solutions global services of identity resolution company Neustar, A TransUnion Company, talked about how companies are using AI to better understand and meet the needs of their customers. Chris Twogood, senior vice president, global marketing at software company Teradata Corporation, offered an example of how a large North American healthcare company is using AI to predict renal failure.
AI has myriad use cases, but many companies are still in the early stages of adopting this technology. What does it take to embrace AI?
Make an Argument for a Business Problem
Twogood suggested getting started by identifying how AI can solve a challenge. “What is your biggest business problem? Then, apply AI to it,” he said.
A large financial institution in the AMEA region was struggling with remote access takeover fraud. It was using a model that took 45 minutes to run, much slower than the fraudsters, according to Twogood. “We got that model running down to 12 minutes. It was able to take care of $100 million worth of preventable fraud,” he shared.
While AI can solve big problems, it doesn’t have to do so all at once. “In the projects I’ve seen successful from our customers or internally, it is just getting moving with the tools you have or small investments and then growing from there,” Mark Maughan, chief analytics officer at cloud business intelligence platform Domo, asserted. “Just get moving. Get started. Test, learn, grow, and iterate.”
Get Stakeholders Onboard
Once you have an argument for the value of AI, you need to get all the stakeholders onboard. “You need, ideally, senior C-suite alignment and support to drive that through because this is a team sport,” Finnerty said. “You need data. You need IT infrastructure. You essentially touch almost every hand in the customer experience lifecycle to get this fully implemented.”
A significant part of gaining that buy-in is having the talent that can communicate effectively with the various stakeholders. Leveraging storytelling to illustrate the problem and how AI can solve it is a powerful tool. The earlier organizations engage relevant stakeholders, the more likely the project is to be successful.
That ability to communicate may or may not come naturally, but it can be learned. “One thing that we've always found very helpful is either rotations or shadowing for anyone in the data science analytic organization around the different business and operational stakeholder groups to get a better understanding of what is actually going on,” Finnerty said. “How is this data going to help impact the processes and the people that you are interacting with?”
Be Transparent
Many organizations, particularly at the executive level, are risk averse. Adopting a new technology, like AI, can certainly drive value, but it involves a level of uncertainty. Implementing a transparent approach helps to address that uncertainty and demonstrate value.
“Create dashboards and metrics, and show folks what's happening, what the plan is and when that plan is successful or not successful, share it,” Maughan suggested.
Look for Opportunities to Operationalize and Scale
AI and data are inextricably linked. “As much as we are excited about AI, we need data for AI,” Srividya Sridharan VP, senior research director at Forrester, emphasized. Data governance and strategy lay the groundwork for effective use of AI. If you don’t have that foundation, operationalization becomes difficult.
“It's really important that when you build the foundation for what you need out of your AI environment to solve your business problems that you think about it end to end and you understand what the handoffs are,” Twogood said. Who is responsible for operationalization? How will insights translate into value?
Once a company has adopted AI, how can it scale its use of the technology? Twogood offered two paths to scaling AI models: vertical and horizontal. In vertical scaling, companies can use fewer models with more data. In horizontal scaling, companies can build more models that run on smaller data sets. He gave the example of a European automobile manufacturer that is running millions of models per day at every point in its car construction to drive higher quality.
Prepare for Disruption
As the adoption of next-generation AI continues, disruptive forces are to be expected. Twogood spoke about automation as one of the biggest disruptors ahead. As the technology evolves, autonomy could be introduced into data prep, data governance and in building out and defining models. Automation could lead to using AI on a massive scale, but it doesn’t mean the end of human intervention.
“Even as automation takes [off], make sure you're looking for events that enable you to do checkpoints,” Twogood urged. “Make sure you are consistently measuring it, consistently monitoring it and understand the value that is coming out of it.”
What to Read Next:
Special Report: Privacy in the Data-Driven Enterprise
How Can Emerging Technology Actually Drive Value for Companies?
Data Strategy: Synthetic Data and Other Tech for AI's Next Phase
About the Author(s)
You May Also Like