AI’s Bumpy Road to Fruition
Almost every organization has engaged in preliminary artificial intelligence modeling and experimentation. But there is still much to be done before most can take full advantage of AI.
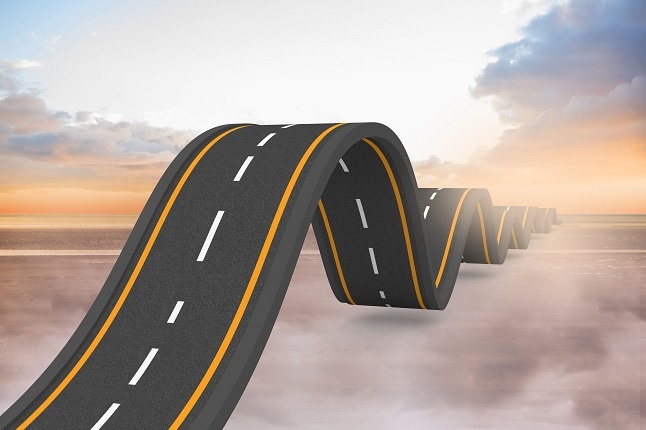
Areas where artificial intelligence has begun to show returns are industrial automation (smart factories), decision support in medical diagnosis, new drug formulations (e.g., COVID medications), and automating business processes such as fraud detection and intervention in financial transactions.
In each of these cases, business value was easily demonstrated in operational savings, loss prevention, and speed to decision.
It’s success stories like these that make AI such a compelling goal for business leaders. Unfortunately, at ground level, there is still much to be done before most companies can take full advantage of AI.
Where Most Companies Stand With AI
In March 2021, a BCG survey revealed that less than half of businesses queried had a mature (AI) program that was installed in production.
In some instances, business use cases weren’t fully defined, and organizations still viewed AI as an experimental technology. In other cases, the use cases were there, but the organization wasn't ready to develop and install them. Barriers to implementation readiness included lack of quality data for AI use, as well as lack of overall readiness of IT, data scientists, and users throughout the organization for full AI deployment.
These were (and still are) the hurdles to overcome:
1. Data silos
Companies are still contending with data silos across their organizations that aren't integrated with other sources and types of data in the enterprise. It will require the development of a comprehensive data fabric that can connect all data and weave it into universally accessible data that everyone can access to eliminate the silos.
Most companies don’t have these overarching data fabrics in place, so the isolated data silos continue to exist, and no one has access to all of the data that could make an AI application truly rich with information. These limitations constrain AI’s ability to produce quality insights that are fully trusted and immediately actionable.
2. Lack of AI tools
Data preparation, extract-transform-load (ETL) tools, business automation and intelligence software, and security governance tools are all needed to fully develop, deploy and support an AI system in production. Many IT and data science departments are still defining these toolsets.
A majority have not yet thought out what their software maintenance methods will be for AI, either. Until toolsets and procedures for maintaining the health of deployed AI systems are defined and implemented, AI will remain in a developmental stage.
3. Lack of personnel with skills in AI tools
IT needs to upskill staff so staff can effectively develop, deploy and support AI. The AI life cycle is iterative. The test for AI is coming within a certain percent (e.g., 95%) of accuracy of what subject matter experts in each discipline would conclude, so designing tests for AI is considerably different than designing a QA script for a DevOps or a traditional software application.
AI also runs on different operating systems and hardware than traditional software. AI’s storage architecture, which may need to store large volumes of data, must be structured between on premises and in-cloud data repositories.
IT leaders will need to focus staff upskilling in these and other areas of AI.
The Road to AI Success
In 2022, organizational readiness will be the prime focal area for companies working with AI, with one key caveat: In 2022, businesses will be expecting AI, analytics, and big data to deliver real-world results.
To deliver real-world results for the business with AI, IT must minimally be able to check the following boxes:
Develop and deploy at least one business use case that “pays off” for the company by delivering faster and more reliable business processes which either drive down costs or enhance revenue.
Deliver data and outcomes that management trusts.
Develop AI methodologies and skillsets in IT so IT can develop, deploy, and support AI successfully.
Ensure robust AI security and governance.
Can IT Do This?
In a November 2021 report, Gartner opined that organizations were still experimenting with AI and struggling to incorporate AI into their standard operations. Gartner’s prediction was that it might take until 2025 for half of organizations worldwide to reach what the Gartner’s AI maturity model described as the “stabilization stage” of AI maturity.
If this prediction holds true, the key for IT leaders in 2022 will be to shepherd AI into smaller use cases that they know will succeed in demonstrating the value of AI to the CEO and other C-level executives. At the same time, CIOs must take steps to acquire tools, build data architectures, and develop staff skillsets that can support an imminent future of more widespread AI deployment.
What to Read Next:
About the Author
You May Also Like