Best Practices for AI Training Data Protection
As artificial intelligence becomes prevalent, protecting AI training data is more important. Here’s how companies can enhance security to safeguard AI data.
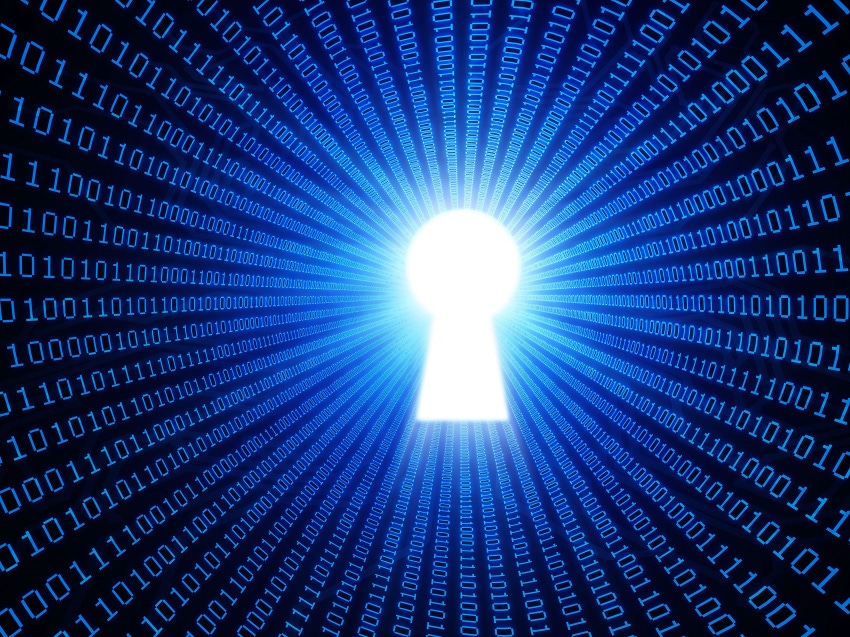
With the rise of AI, data protection challenges are evolving in parallel with the emerging technologies to both threaten and protect an enterprise’s data assets. When training AI, the massive quantities of data utilized for AI models pose new and unique data protection challenges that require innovative solutions.
To update enterprise data protection strategies to accommodate the needs of the AI training data you must first understand the specific challenges and solutions involved in AI model training.
What is AI Training Data?
AI training data refers to the data used to train generative AI models. These models typically analyze vast amounts of information to recognize patterns or trends, which they use to create new content. The performance of a model often improves with the addition of more relevant data that is accurate and managed in easily identifiable standards.
Challenges of AI Data Protection
AI training data is often repurposed from existing data sources. For instance, businesses may train models using data originally generated for other purposes, such as emails, IT tickets, customer support conversations, or even legacy data like weather reports or historical supply chain distribution timelines. This approach helps the models better understand the context and optimize various business processes.
That said, data that organizations use for AI training purposes is different from data that exists in other contexts, which creates unique challenges:
Data volume: AI training data is typically massive, often requiring millions or even hundreds of millions of records, including images, videos, audio files, or unstructured data like documents. Securing and protecting such a vast amount of data is a significant task.
Disparate data types: AI training data can encompass diverse types of information, making it challenging to assume uniformity across all data records or to support specific technologies without adaptation.
Non-continuous use: AI training data isn’t continuously used unlike operational data. It’s only needed during active model training, with intermittent retraining using the same data at later points. Cost-effectively storing this data for future use is paramount.
Sensitive information: AI training data often includes sensitive information, such as personally identifiable information (PII) related to customers, vendors, or employees. Proper security and compliance measures must be in place to protect this data from unauthorized access or misuse.
How to Effectively Protect AI Training Data
To create a data protection strategy for AI data, begin by implementing fundamental data protection practices crucial for any type of data, such as:
Encrypt data end-to-end: Encrypting data at rest and in transit is a fundamental data protection measure. Even if your data is expected to remain within your organization during training, encryption adds an extra layer of security in case of unauthorized access.
Log and monitor data access: Tracking and monitoring data access helps detect unauthorized activities and potential security threats.
Comprehensively back up your data: A robust backup strategy ensures the recovery of training data in case of accidental or deliberate loss, crucial for ongoing retraining.
Manage third-party data access: When external vendors engage in AI training or model management, ensuring compliance and auditing data access becomes more complex.
In addition to these basic measures, several additional data protection strategies can help safeguard AI training data.
Data minimization: This involves collecting and utilizing only the necessary data for a particular AI application. For instance, if you’re training using emails but only certain emails are relevant, filter out the remainder as irrelevant. This approach can accelerate training operations (due to less data to process), reduce the data volume for backup, and minimize data loss in case of a breach.
Data compliance strategy: Identifying the compliance and regulatory requirements necessary training data must adhere to is essential. Apart from the usual standards for sensitive information, rapidly changing AI regulations may specify ways to manage or store training data.
Secure data storage: Due to the high volume of AI training data, businesses often opt for cost-effective solutions, commonly found in Cloud storage services. However, it's crucial to select a Cloud storage provider that offers strong security features, including encryption, network security measures, and compliance with industry standards and certifications (like ISO 27001 or SOC 2). Prioritize data security over choosing the cheapest storage option to avoid putting your data at risk.
Managing third-party vendor risk: If your AI strategy involves granting external vendors access to training data, establish clear policies regarding the permissible uses of your data. Additionally, assess their internal security controls, policies, and incident response capabilities. Remember, you could be held accountable for compliance or security incidents resulting from inappropriate use of your data, even if it’s by a third party. It’s crucial to prioritize data protection even when your training data is managed by an external organization.
As AI becomes more prevalent, the need to manage and protect AI training data is increasingly necessary. The unique challenges posed by AI data protection are evident. A sensible first step for businesses is to devise strategies that enhance security to safeguard this critical AI data, like how businesses protect their other internal data. Subsequently, enterprises should consider how AI can enhance existing security strategies, via tools or through precise and efficient threat detection capabilities. With this assessment, businesses will be well prepared to engage AI and rest assured that their AI training data is well protected.
About the Author(s)
You May Also Like