Conversational AI: How It Works and Where It's Headed
The next person you speak with may not be a person at all. Welcome to the uncanny valley of conversational AI.
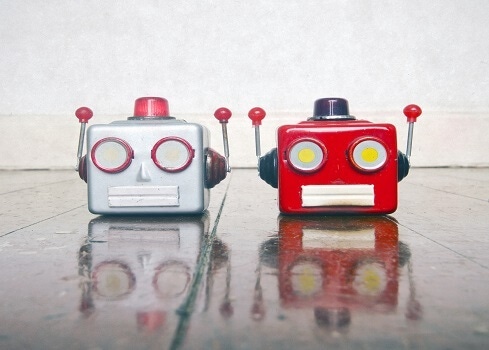
Current chatbots tend to be awkward and even agonizing to use, limited to answering a set of simple queries -- often incorrectly. But what if a chatbot could be designed to support more complex and multistep tasks, such as organizing a daily schedule or pinpointing a fault lurking inside a complex mechanical device? Conversational AI technology promises all of this and more.
Conversational AI is built on natural language processing (NLP) and other machine learning (ML) technologies, with the goal of enabling human-like interactions between machines and people. So far, conversational AI has mostly been used to create sophisticated chatbots -- as opposed to scripted, rules-based chatbots. “It's widely used in marketing and customer service contexts because it can significantly improve reach, responsiveness, efficiency, and personalization and, at the same time, reduce cost,” says Yan Huang, an associate professor of business technologies at the Carnegie Mellon University Tepper School of Business.
Early attempts at conversational AI targeted simple customer service applications. “However, we're now seeing an expansion of conversational AI applications in other areas, from internal IT support to maintenance and manufacturing,” says Dan Simion, vice president of AI and analytics at IT and business advisory firm Capgemini Americas.
A telecommunications company, for example, may have a technician who's trying to fix a technical issue inside a customer’s home. As the technician works to troubleshoot the problem, conversational AI can guide the tech through the task. “Instead of going through a manual to fix the modem in the house -- or in the case of manufacturing, fix a piece of machinery back at a factory or plant -- technicians can ask questions through a chatbot-type of solution that gives them answers on the spot,” Simion explains.
AI Concierge Service
In its current nascent stage, conversational AI is mostly used in customer call interactions. “It's often the first interaction that you might have with a company to direct your call, respond to simple requests, or to locate a particular person,” says Theresa Kushner, data and analytics senior director at global IT services provider, NTT Data Services. “In this way, it acts like a company concierge, providing service at a fraction of the cost of a human interaction.”
Existing technology allows a machine learning model to be trained to recognize words or phrases that indicate a specific user intent, such as checking a bank balance, scheduling meetings, asking HR questions, and opening customer or employee support tickets. “Known as natural language understanding (NLU), the model is able to generalize and recognize the intent of what the user is asking,” explains Gillian McCann, CTO at Workgrid Software, a company owned by Liberty Mutual Insurance.
Once user intent has been recognized, and the dialogue continues, named entity recognition (NER) technology steps in to extract any additional information that may be needed to fulfill a required task. “When all the information has been gathered, standard application integration approaches are used to perform the actual task or retrieve the required information,” McCann says.
Kushner notes that conversational AI is now approaching the point where it's able to handle complex interactions with customers, learn from those interactions, and provide a level of customer support that never tires, never gets angry, and always provides answers.
Simion agrees. “We’re seeing these applications getting more intelligent,” he says. “Once a question is asked by the user, the conversational AI tool provides an answer [and] then, when a follow-up question is asked, it continues to pinpoint the right information based on the entire context and history of the conversation.”
Room for Improvement in Conversational AI
Despite recent progress, conversational AI technology is still relatively limited. User experiences vary widely, even across different channels within the same organization.
“This leads to customer frustration, and everybody has a bad tale to tell,” says Wayne Butterfield, director of ISG Automation, a unit of technology research and advisory firm ISG. “The good news is that when conversational AI is done well, it’s available, helpful, and immediate.”
At the end of the day, companies need to make sure conversational AI is helpful and isn’t frustrating users. “We’ve all had experiences with customer service chatbots that may not understand what we're asking or are routing us to the wrong decision-maker,” Simion says. “Making sure conversational AI tools are useful and not frustrating is crucial to further adoption.” There's still plenty of room for improvement to be made, he added. “The entire experience is a decision tree based on the user’s inputs, and the conversational AI tool must make sure the user gets onto the right branch to gain the information and answers they seek.”
Security and privacy are also potential stumbling blocks. “Users may be concerned about the security of conversational AI applications because the technology requires constantly collecting data about users and their interactions with the applications and, thus, are vulnerable to data breaches,” Huang explains. To address this concern, security and privacy protection should be built directly into conversational applications, she notes. “Some end users may not trust machines in general, hence it is also important to educate users on the safety and benefits of conversational AI to improve users' trust toward the technology.”
What to Read Next
What Some Customers and Employees Hate About Chatbots
About the Author(s)
You May Also Like