Data and Analytics: For and of the People
Organizations today generate massive amounts of data, but unlocking the power of data and analytics begins with democratization.
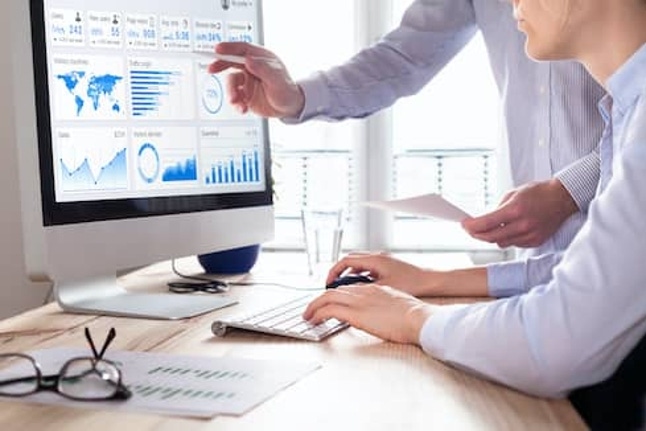
In the last decade, data has earned its rightful place as an asset of the business after decades of languishing as the exhaust from business processes. A 2020 study found that data-driven organizations were 162% more likely to exceed revenue targets than others. Other estimates may be more, or less, optimistic, but all agree that data-native organizations outperform those that are not, by a long margin.
Successful data-native organizations foster a culture of data to compete, differentiate and responsibly leverage the innate power of data and analytics. With modern design patterns for managing and utilizing data in cloud ecosystems, experts predict that, in the coming months, 75% of centralized analytics initiatives will make way for a federated model where local business-domain analytics units will come to share authority and decision-making with the central team.
This implies not just the devolution of power, but also the evolution of analytics from a business support activity into a core business function.
Data Literacy
For the most part in a data economy, data is a loss-leading asset, meaning while it is invaluable to conducting and competing in business, the upkeep and value of it is not directly realizable in a balance sheet, unless the business itself is selling data. To optimize a loss-leader asset like data, a data-native enterprise should embed data literacy in every section of its business model.
Thus, forward thinking organizations have developed governance models that encourage citizen data scientists to innovate with data and analytics. This essentially translates into effecting a cultural transformation, so D&A is seen as a valuable resource owned and nurtured by the enterprise, and thus become the DNA of the business.
Democratic Data
A culture of data is institutionalized by developing a democracy of data. A change of this scale, which often is like a constitutional amendment for most organizations, needs to be driven from the top, with leadership responsibility extending all the way to tackling the challenges of operationalization.
Extensive collaboration between diverse/cross-functional teams -- both within the enterprise and in tandem with external ecosystems -- is critical for ensuring the right resources are available for the program.
This calls for bringing experts, such as data scientists, out of their ivory towers. Some organizations are blurring the boundaries by deploying D&A staff in line roles, by rotation, while others are adding analytical roles -- reporting into the data organization -- to their business functions. Evolved organizations are going further, but in the opposite direction, to train business employees in coding basics and quantitative concepts.
It is also crucial to balance IT and business roles, so that in allowing business teams greater autonomy, the expertise of the central IT team does not go unutilized -- for example, knowing how and where to deploy influential technologies like AI, IoT, mobility, cloud computing, robotic automation and of course, various types of analytics.
Data Translator
There is competitive advantage in developing the best data-estates using the most advanced engineering techniques and crafting innovative mathematical models to deliver differentiated insights for business users. However, unless these are aligned to a business context and interpreted for a business goal, most of these become much ado about nothing.
Increasingly, organizations are developing a practice of ‘data translators’ who have a business empathy, as well as a strong foundation in data and mathematical engineering and who become the pivot for maximizing investments in data assets. Neither a PhD in mathematics nor an MBA from a reputed business school, is a pre-requisite for these data translator.
A business user with a penchant for being data-driven, can easily be trained to leverage data and mathematics to develop insights that are pertinent to the opportunity. On the other hand, a data engineer or a data scientist, who has deep domain knowledge through experience, can utilize the data and analytics solution to drive a purposeful business narrative.
The ‘data translator’ practice can be embedded within the frontlines of the business or created as a horizontal and fungible pool to maximize cross-pollination and re-use.
Low-Code and No-Code
Enabling sophisticated data and mathematical coding using drag-and-drop interfaces has now become a key-enabler to amplify citizen data-scientists and data translators. With easy interfaces and rich graphics, modern cloud-enabled platforms can develop data solutions, such as visual analytical dashboards, trends analysis, predictive insights, and prescriptive narratives, with some of them being auto-generated. These can empower real-time decision making for business users at every level and in every role, from a warehouse manager who needs to take inventory decisions to a chief marketing officer who is trying to assess how well a new campaign will be received by its target audience.
Summing Up
The value of data and analytics increases with its adoption across the enterprise. But even among the world’s top companies, only 24% have managed to create a data-native organization. In a difficult environment riddled with uncertainty, disruption and competitive pressure, enterprises cannot afford to forgo the considerable value they can derive from data.
About the Author(s)
You May Also Like