Enterprise AI is Hard. These 3 Guidelines Fuel Success
Fundamentals of operationalizing artificial intelligence help companies chart a course for early wins.
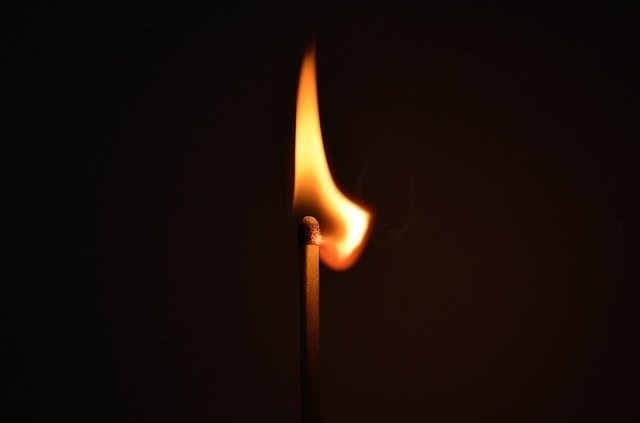
AI is becoming increasingly ubiquitous -- from enterprises to the edge. It’s a movement accelerated by the pandemic, which sped up many companies’ planning and implementation of AI projects. Some 86% of respondents surveyed by consulting firm PwC reported that AI is becoming a mainstream technology at their companies.
The reason? Companies had to adapt quickly to a whole new business landscape, faster than ever.
Yet, while AI is making rapid inroads as a tool to solve complex business challenges, many enterprises still struggle with the move from testing to deployment. In fact, a 2022 O’Reilly survey found that just 26% of respondents report having AI currently in production. This can be caused by anything from a lack of skilled staff to unrealistic expectations for an initial AI project.
Enterprises can plan for success by focusing on three areas for operationalizing AI: understanding the AI lifecycle; building skills and expertise; and leveraging MLOps to harden AI for production.
1. Understand the AI lifecycle
Understanding the complete AI lifecycle is crucial to preparing for successful deployments. Teams need to collect and prepare data, build a model, train the model, deploy the model, run inference, and then monitor it to determine if the model is delivering accurate results.
Few IT teams expect traditional enterprise applications like databases, spreadsheets, and email to evolve much once deployed. Their AI counterparts, however, typically require frequent monitoring and updates to keep the application relevant to the business and aligned with market changes.
For example, a recommender system requires seasonal updates to make sure it’s able to suggest movies, music or products tied to a specific holiday or event. It also needs to evolve as consumer tastes and trends change.
Having a broad view across the full AI development lifecycle also helps enterprises ensure they have the right people to support AI, from development to production deployment. Companies may need data scientists, AI developers, machine learning engineers and IT experts to build out a comprehensive team.
2. Build foundational AI skills with learning labs and pretrained models
Smart companies are building their AI teams by hiring AI experts and upskilling current employees for new roles. This provides unexpected benefits: both groups can learn from each other as they work to integrate new AI capabilities into the company’s operations and culture.
Hands-on labs also serve as a launchpad to accelerate the journey to successful AI deployments. Labs can teach teams a broad range of key AI use cases, from developing intelligent chatbots for customer service, to employing image classification for an online service, to boosting safety and efficiency on a manufacturing line, to training a large-scale natural language processing model.
In addition to labs, third-party enterprise AI software helps enterprises quickly train, adapt, and optimize their models. Libraries of pretrained models are also available to give enterprises a head start that speeds time to AI. These can quickly adapt to a unique application and integrated with customized models for testing and deployment.
3. Support enterprise-grade AI with MLOps
Once an AI model is ready to deploy, companies need to operationalize it before it can run in production with enterprise-grade reliability. Machine learning operations, better known as MLOps, builds on the well-known principles of DevOps to establish best practices in enterprise-grade AI deployments.
Part process, part technology, MLOps enables enterprises to ensure that AI applications are as dependable as traditional business applications. MLOps software platforms help enterprises operationalize the AI development lifecycle, with testing and hardening at each stage.
Unlike most developer software, enterprise ready MLOps solutions feature 24/7 support to ensure that experts are always ready to address any issues. And just like any other enterprise application being evaluated for adoption, it’s key to read software licensing agreements before adopting AI software or systems. No company wants to learn that a key platform isn’t supported by its provider at the moment help is needed.
Planning, Training and Process Lead to Early Wins
Every major computing paradigm shift brought challenges before becoming the de-facto standard of operations. AI is no different.
Understanding the AI lifecycle and knowing where to look for support and shortcuts -- enterprise AI labs and pretrained models -- creates a foundation for delivering enterprise-grade AI.
About the Author
You May Also Like