Structure Your Analytics Team for Success in the Age of AI
The data team structure that your company has used for a few years needs to turn its focus to results and putting analytics tools in the hands of business units.
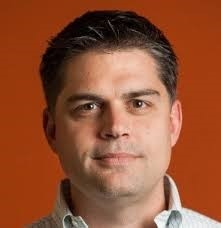
Despite major advances in data technologies in recent years, access to data remains a constant source of tension among IT teams, knowledge workers, and the C-suite. Everyone wants access to more and better data to improve their line of business, but visibility into critical business insights is still primarily limited to senior executives and the data experts delivering them.
What’s going on here? In my experience, it comes down to the steep technical requirements that still exist in many analytics platforms today. The complexity of the tools and datasets makes them inaccessible to everyday users, which in turn leads to massive bottlenecks in the data department.
That's all set to change with the rise of AI. According to the latest research from McKinsey, two-thirds of the opportunities in AI revolve around improving the performance of existing analytics techniques. What’s more, the researchers estimate that applying AI techniques to data analytics has the potential to create $3.5 to $5.8 trillion in value annually.
But to deliver on the promise of emerging applications of AI in data analytics, a fundamental shift needs to happen in the way data teams are structured. After more than 20 years leading the data initiatives at businesses like Disney and AOL, I’ve learned a thing or two about turning the corner.
Focus on the business – not the tech
Today, most data teams are organized around the specific capabilities (and limitations) of data technology and the resulting workflow. It’s often a combination of:
Data engineers who build and maintain a data warehouse
Business analysts who translate business questions to data-specific questions
BI analysts who run queries and build data models
Administrators who oversee permissions and access to the data warehouse.
But with AI changing traditional data workflows by expanding access to all knowledge workers, the duties of data teams need to evolve as well. No longer gatekeepers, they must focus on helping other teams use new data technologies to drive change across lines of business. For example, business analysts should focus on helping non-technical knowledge workers use technology to answer their own questions, rather than turning their questions into queries themselves.
New roles will also emerge. For instance, the best teams have at least one data evangelist in their ranks, someone who lives and breathes data, can help accelerate the journey to becoming more data-driven by answering questions and guiding teams and individuals as needed.
Rethink goals and metrics
As traditional data roles change and new ones emerge, businesses need to rethink how they evaluate the effectiveness of their data team’s initiatives. Legacy metrics – such as the average time it takes to generate a report, the number of reports being created, and backlog volume – won’t cut it. Looking ahead, data teams need metrics that map to goals and objectives of analytics in the Age of AI, first and foremost, tangible business results.
At the highest level, establishing a culture of data literacy should be the primary goal. That is, creating an environment where everyone in the organization – from top to bottom – prioritizes insights based on empirical data over intuition and feels empowered to answer questions with data on their own.
The first step for most organizations is conditioning teams to ask for data. Once people start asking for data, others will pick up on it quickly and follow suit. To drive this in an organic way, data teams should work with departments to revamp metrics around internal workflows and processes. For example, updating proposals, sales decks, project goal setting, and even performance reviews to make them more data-oriented.
Data teams also need to ensure that data is universally accessible and focus on getting clean, safe, and timely data into everyone’s hands. In terms of metrics, that translates to declines in the number of reports requested and generated, declines in traditional tool use, and so on.
Pick the right leader
In recent years, discussion has intensified around who exactly is responsible for driving digital transformation through analytics. Many assume it’s the CIO because they have "information" in their title. But the reality is that CIOs already have a job, and it’s related to the management of data (e.g. security, privacy, and scalability) — not the actual use of it.
What’s needed is a Chief Data Officer (CDO), a leader who can balance the demands of new and existing analytics requirements, champion the use of data across the entire organization, and foster a culture of data literacy. Specifically, you want someone with a strong understanding of legacy data models and workflows. That’s because large-scale cultural change comes from within – and it comes from the top – which means CDOs need a deep understanding of how it works today, especially from the leadership team’s point of view.
What’s more, you want a leader who can balance the needs of IT and the business, and who has an eye for detail while also being able to stay focused on the big picture. They must be able to educate teams and drive adoption throughout the organization by teaching people not only about the tools and technology available today but also why adopting them is mission-critical for the business.
Doug Bordonaro is chief data evangelist at ThoughtSpot.
About the Author
You May Also Like