Talent Alert: Data Science Help is Coming
But will the flood of candidates solve your data problems? Here are a few issues to mull and tips on how to attract/retain data scientists in this hot market.
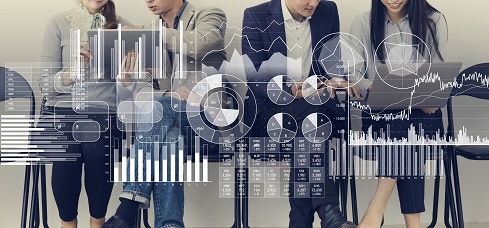
No matter which industry, just about every company has more data than ever before. And almost universally, these companies either want to use that data to make their businesses smarter and more competitive, or they want to find a way to turn it into new revenue. Either way, there’s one thing every one of these data-enriched firms need: more good data scientists.
I live in San Francisco, where the free agency wars over data science talents can run incredibly intense. You’d be hard-pressed to find a chief information officer or business leader who hasn’t had to battle over hiring a data scientist or three.
But even outside of the best and brightest engineers in Silicon Valley, the collection of skilled professionals who are deeply versed in the data needs of an industry (for example, marketing or travel) is even smaller -- and thus the talent battles are that much fiercer.
That’s why you’re naturally seeing tons of talent flood into this sector. If you have a college-aged son or daughter looking for a viable profession, chances are someone has nudged them towards becoming a software engineer.
According to a recent report from Bain & Company, the global supply of IT professionals with advanced analytics skills is set to double between late 2018 and late 2020 to one million workers.
So, there is a clear need for a “flood” of college graduates with degrees in subjects like computer science and data science in our country. This should all be good news.
Yet, we all need to view this trend with open eyes and temper our expectations. A cadre of newly-minted data scientists won’t solve all our needs overnight.
Unfortunately, simply bringing on more analytics talent won’t solve our data challenges for these five reasons:
1. Much of academia teaches data processing as if it takes place in a perfect world: Most data scientists find themselves thrust into messy projects at imperfect or technologically-delayed companies. Data science is not as exact a science as we’d like and, unfortunately, too many graduates lack the real-world experience to understand this. Certainly, we can push the education system to better prepare students to enter a job market that necessitates a more realistic and practical approach to data science.
2. Hiring is expensive: Given how in-demand sharp analytics talent is, the going rate for hires is pricey and keeps getting higher. It’s very difficult for less glamorous industries to compete for this talent with the leading tech companies of the world.
3. New graduates need real-life skills and experience: To be useful to most big companies, data scientists need to become well versed in the unique nuances of an industry or company. This can take three to five years and most companies are looking for impactful hires right away.
4. Retention is hard: Once your new hires get the experience they need, they become poaching targets. Given the robust market, these people are going to get offers from competitors.
5. Corporate data challenges today are very different than 10 years ago: Today, data is big and many of the newfangled algorithms are resource hogs. Data scientists often need to use computing power in spurts. They may need a vast amount of computing for a few hours and much less in between. Cloud computing is the solution, but unlike in the past, they can’t simply lean on the IT department when they need help managing all that computer processing power. Instead, today’s data talent needs to support itself.
In a sense, you need to be your own IT department during those heavy usage spikes. Unfortunately, most new hires have not been trained to work this way. They ideally need a deep understanding of statistics and machine learning and cloud computing administration -- yet those skills are typically taught by different departments.
Ok, so it won’t be easy. What should companies do to ensure talent success? Here are a few ideas:
Provide training: Companies need to get their organization set up to train new talent right way. This means having the right mentors in place from the get-go.
Hire strategically: Companies shouldn’t just go out and hire as many great data science professionals as they can find. Instead, they should work backward from their needs. It’s important to figure out where you need support and which aspects of the business will benefit the most -- and hire accordingly.
Be aware of your data science opportunities and limitations: The adage -- from the prehistoric times of data mining -- of “no data no mining” is still valid, but in the era of big data everywhere the less snappy “no ore, no profit” is also true. Just because you are collecting terabytes of data doesn't mean that data is complete and clean enough to be useful or even contains the information you are looking for.
Provide incentives: Not every company can compete with the tech titans on massive salaries or stock options. So, it’s necessary to find ways to get new hires to buy into the company culture. You need other ways to entice employees to sign on and stick around.
Regardless of the challenges that a tidal wave of raw talent presents, there’s little doubt that more skilled professionals entering a crucial field is largely a good thing. It’s just important to recognize that amidst the hype and hiring rush, there are some stark realities to face.
In the end, the most successful data-driven companies will be the ones that have a clear plan on how building out a larger data science team will help their business for years to come.
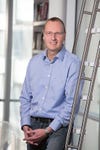
Dirk Beyer is Neustar’s SVP of Data Science Research and the General Manager of OneID, Neustar’s identity data platform. Before that, he worked as MarketShare's Chief Science Officer, leading both analytics delivery and research teams in Marketing Analytics. Beyer has more than 20 years of professional experience in the design and development of analytical solutions. He holds a master’s degree in education and a Ph.D. in operations research from Leipzig University.
About the Author(s)
You May Also Like