Top Trends in Data Lakes
Does it seem too early for data lakes to have trends? The reality is data lakes are on the very edge of business transformation efforts and dramatic change.
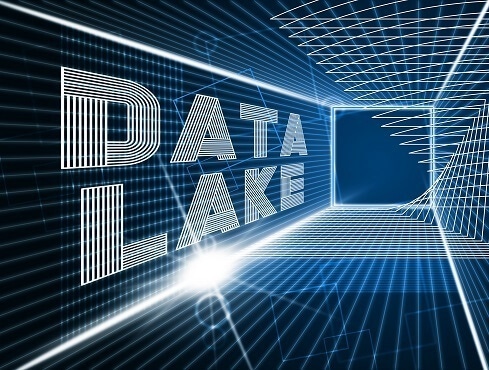
Data lake platforms load, store, and analyze volumes of data at scale, providing timely insights into business. Data-driven organizations leverage this data in many ways -- advanced analysis to market new promotions, operational analytics to drive efficiency, predictive analytics to evaluate credit risk and detect fraud and many other uses.
While it may seem like early days for the data lake idea to have trends, the reality is that data lakes are on the very edge of business transformation efforts and therefore there are some dramatic changes happening to them now. Some lakes have even failed, but most of those organizations have retrenched and are coming back for its value proposition.
These are trends that will be tied not only to the data lake, but also to data maturity, and company maturity.
The rise of the lakehouse
The most glaring trend is the merger of the data lake and the data warehouse. The effective “lakehouses” combine a data warehouse on an analytic database that meets enterprise SLAs for performance at scale with a cloud-storage based data lake. The combination is primarily the ability of the data warehouse to reach into the cloud storage as necessary. These structures also live on a pipeline with the cloud storage serving as staging for the data warehouse, which will contain a subset of the data (though as much as is needed for high-fidelity analysis), and the data lake, which data scientists will primarily use.
Explosion in sensor-based time-series data and edge AI
Data volumes are expanding for many organizations as many are now leveraging 5G and IoT data. The number of sensor-driven sources has grown tremendously, and the data being generated is largely time-series data. This data is generated for every point in a small measure of time and collectively represents how a system/process/behavior changes over time.
Embedded databases are built into software, transparent to the application’s end user and require little or no ongoing maintenance. Embedded databases are growing in ubiquity with the rise of mobile applications and internet of things (IoT), giving innumerable devices robust capabilities via their own local database management system (DBMS). Developers can create sophisticated applications right on the remote device. Today, to fully harness data to gain a competitive advantage, embedded databases and the corresponding data lake intake need a high level of performance to provide real-time processing at scale.
Those using IoT can use embedded databases at the edge to process data immediately, even with artificial intelligence, and to copy the aggregated IoT sensor data to a data lake, while aggregating data from all the IoT devices in the data lake to develop analytics.
All these web, mobile, and IoT applications have generated a new set of technology requirements. Embedded database architecture needs to be far more agile than ever before, and requires an approach to real-time data management that can accommodate unprecedented levels of scale, speed, and data flexibility.
Leveraging cloud storage for data lakes
Data lakes have almost become synonymous with cloud storage in the industry vernacular. Early data lakes utilized Hadoop (HDFS storage), but many jumped in when cloud storage presented a better option. Cloud storage presents a more achievable separate compute and storage architecture where compute resources (Map/Reduce, Hive, Spark, etc.) can be taken down, scaled up or out, or interchanged without data movement. Storage can be centralized, with compute distributed.
Some even have mechanisms to ensure consistency to achieve ACID-like compliance for remote data changes and remote data replication to ensure redundancy and recovery.
Data integration automation
This is a more general trend than just data lakes. Most enterprise data integration is not to the data lake, but much of it will be.
Data integration constitutes upwards of 75% of the work effort in any data lake initiative. However, the absolute time is going to go down as AI gets ahead of the need upon identification of the source and target. “Common” data integration rules will be suggested or automatically applied. As enterprises grow more comfortable with the automated process, the automation of data integration will grow and efforts around the data lake will shift to management and access.
Retaining structure in structured data
Though you can do schema-less data loading in a data lake, it is important to know when and when not to build a schema for data. As a general rule of thumb, retain structure for already structured data and take the time to build schema for data that has high business or analytic value or is often queried by users. For less important or less-accessed data, or where schema will not be valued, create schema on an ad-hoc or as-needed basis. You can also add data to the lake and create the schema when the data needs to be utilized.
Data quality additions
Another trend in managing a data lake is to build it so that you can handle data quality issues, such as de-duplication. This requires additional planning to make it such that the data lake information remains up to organizational standards for accuracy, consistency and completeness. Data lakes will be brought into your data management and governance processes, just as you would for any information asset. This requires the governance to be light and agile, not heavy-handed and dictatorial. Taking the time to ensure that data quality improvements propagate throughout the lake will keep it providing consistent value and be a trusted resource for your data consumers.
Building a data lake is certainly the right response to alleviate the exponentially growing data needs of the modern enterprise. However, getting value out of a data lake over the long haul requires good information management discipline and tools and the uptake of trends like these that save time and money and add value.
About the Author(s)
You May Also Like