What to Know About the Differences in Analytic Architecture Patterns
Enterprise data architects should take into consideration the data lakehouse, data mesh, and data fabric when constructing the analytic environment today. Here are the main ideas these patterns offer.
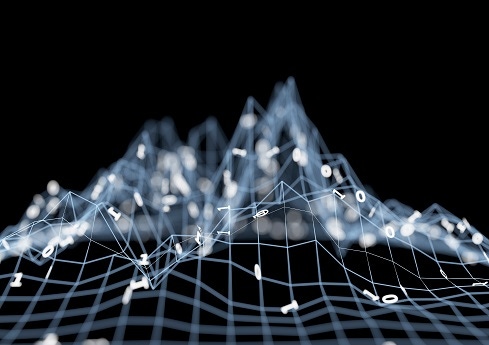
Several architectural patterns have emerged that decentralize most components of the enterprise analytic architecture of which data lakes are a large part today.
The data lakehouse, data mesh, and data fabric all offer ideas for the enterprise data architect to consider today when it comes to architecting the analytic environment. They are not mutually exclusive and the best pattern to follow may be customized pattern with input from all. In this article, I will explore the main ideas these patterns offer.
The Data Lakehouse
The messaging of all the major data platform vendors today is focused on the concept of a lakehouse architecture, which combines the best elements of traditional data warehouses on relational databases with scalable and lower cost per capita data lakes on cloud storage.
This is a good idea in principle but also creates numerous points of failure if it’s not engineered appropriately.
Data lakes were created to manage unstructured data in various formats on lower cost storage for data science and machine learning, but they lack key characteristics of data warehouses around data quality, and it can be difficult to mix a variety of data integration jobs with appends and reads.
Determining what data goes into the lake(s), what data goes into the warehouse(s) is a still a relevant skill in the data lakehouse architecture. Data lakes and data warehouses serve different purposes and should be used in conjunction with each other for optimal data management. Data lakes are ideal for storing raw, unstructured data while data warehouses are designed for structured, processed data.
Some of the most important queries that an enterprise runs today span the boundaries of the data lake and the data warehouse. You can’t store all data everywhere just in case, in order to avoid a cross-platform query.
The data lakehouse is a combination of data lake and data warehouse capabilities that has been enabled by several key technology advancements. Metadata layers now provide the ability to set up drill-through paths for data lakes, allowing for greater navigability and increased performance. New query engine designs are allowing for high-performance SQL-like execution on data lakes, and access to data science and machine learning tools are being made available.
Significant engineering went into building the coordination between a lake and a warehouse so that queries can access not only the correct data they are looking for, but also use the most expedient route in doing so. Vendor examples include Redshift Spectrum, Snowflake External Tables, BigQuery Omni and Synapse External Data Sources.
The Data Mesh
The challenges of a single enterprise data lake architecture include a departmental ownership model that would naturally have a limited focus. This can therefore limit collaboration. Since most operational systems are likewise owned by a single department, this could limit the technical focus. It is necessary to learn an area of the business to a degree before developing an architecture that works broadly, and few builders stray beyond their primary focus.
Just as some organizations are too large to expect a single data warehouse to be practical, the same goes for the data lake. The question becomes how to settle the data warehouses, data lakes, and data integration pipelines into the organization reasonably, striking the balance between production and specificity.
The data mesh architecture decentralizes and decouples components by business domain in recognition of the importance of context, such as how different businesses define “customers.” This allows for design flexibility, particularly for self-service, while managing the enterprise management needs.
The data mesh enables businesses to swiftly and effectively modify their data governance procedures to satisfy ever-changing demands. Adaptive data governance can assist businesses in reducing data breaches, protecting customer privacy, and remaining in compliance with laws and regulations. Additionally, the mesh can assist businesses in enhancing the accuracy and quality of their data. Also, by ensuring that data is utilized effectively and efficiently, its adaptive data governance can assist organizations in maximizing the value of their data.
The data mesh could manifest in the architecture through sensible, decentralized yet architected multiple data warehouses, multiple lakes, and multiple data integration pipelines that still adhere to enterprise components where possible.
The Data Fabric
Data fabric designs are based on the idea of providing extensive data virtualization capabilities and loosely linking data in platforms with applications that require it.
Knowledge graphs and metadata management are used in data fabric architectures to combine data from different data kinds and endpoints. This helps data management teams group together datasets that are linked to one another as well as incorporate brand-new data sources into an organization's data ecosystem.
Automation is a significant area of capability that the data fabric and the access to all enterprise information it provides have made possible. The mesh approach automates parts of managing data workloads, resulting in productivity advantages, but it also aids in breaking down data system silos, centralizing data governance procedures, and enhancing overall data quality.
The “fabric” of virtualization can traverse clouds. In a multi-cloud context, one cloud, like AWS, may manage data inflow while another platform, like Azure, is in charge of data transformation and consumption. This is an example of a data fabric architecture. Then a third vendor, such as Google, might offer analytical services. These settings are connected by the data fabric architecture to produce a single view of the data.
The enterprise data architect should take into account the data lakehouse, data mesh, and data fabric when constructing the analytic environment today. As always, if you’re trying to solve an important problem, you should judge the solution by measurable progress, not how it sounds on paper.
McKnight Consulting Group is revolutionizing the way businesses leverage data, analytics and artificial intelligence by crafting and creating the necessary data infrastructure for organizations to succeed. www.mcknightcg.com.
About the Author
You May Also Like