Natural Language Processing IDs Medical Complications
Embedding NLP into electronic health records allows clinicians to spot more postop complications, finds JAMA study.
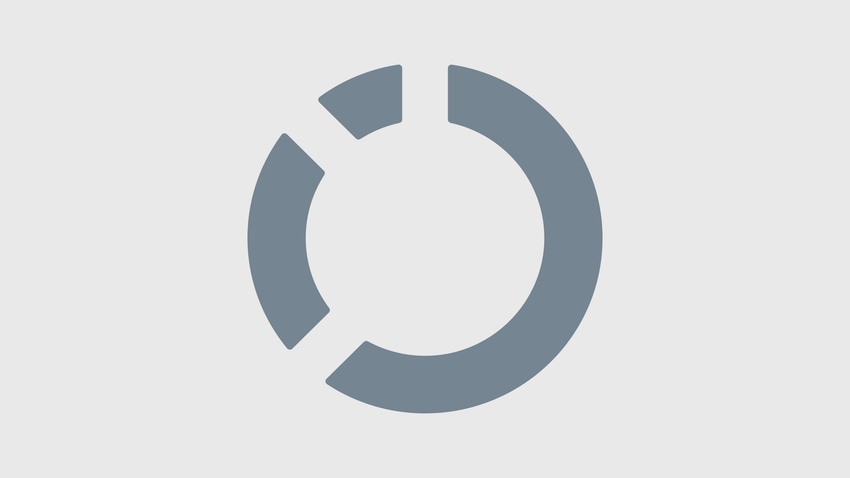
Healthcare Innovators
Slideshow: Healthcare Innovators(click image for larger view andfor full slideshow)
While we have yet to reach the holy grail of converting free text to structured data in an electronic health record (EHR), natural language processing (NLP) is beginning to show real promise in healthcare. The latest indication of this is a study showing that the application of NLP to free text in an EHR identifies postsurgical complications more accurately than the analysis of discharge billing codes.
The study in the Journal of the American Medical Association compared the NLP method to the use of administrative data in the Veterans Affairs Surgical Quality Improvement Program (VASQIP). The study population is a randomly selected sample of nearly 3,000 patients treated at six VA hospitals between 1999 and 2006.
The researchers focused on six post-operative complications that the VASQIP nurse reviewers had searched for using patient safety indicators based on billing codes. These included acute renal failure requiring dialysis, sepsis, deep vein thrombosis, pulmonary embolism, myocardial infarction, and pneumonia. The results showed that NLP was more sensitive in detecting all but one of the complications. On the other hand, NLP had somewhat lower specificity when compared to using administrative codes. (The more specific an assessment tool is, the better able it is to rule out the existence of a complication that doesn't really exist.)
NLP accurately identified 82% of the renal failures in the study population, compared to 38% for administrative data. Similar results were obtained for venous thromboembolism (59% vs. 46%) and pneumonia (64% vs. 5%). The sensitivity of NLP to myocardial infarction was 91%, nearly the same as the 89% for the billing code analysis.
Specificity, defined as the percentage of hospitalizations without targeted complications that were not flagged, was 4% to 7% higher for the quality-indicator method than for the NLP approach.
Aside from the NLP method's superior accuracy, the researchers observed, it offers several advantages for hospital quality improvement programs:
-- It can be easily adapted to any strategy an institution uses to improve quality.
-- An NLP search can be done on each day of an inpatient stay to identify complications while a patient is still hospitalized.
-- The scalability of an NLP strategy allows surveillance of the entire patient population.
-- In systems with enterprise-wide EHRs, the use of NLP for prospective surveillance could be extended to the outpatient setting.
Using NLP to identify hospital complications is a big leap forward beyond billing codes, said Carol Haraden, VP of the Boston-based Institute for Healthcare Improvement (IHI). However, she cautions, being able to identify complications more accurately won't necessarily improve patient safety.
"We already have a tremendous amount of data we could use to improve safety if we wanted to. More data doesn't necessarily mean more improvement."
Many studies have shown, she added, that clinical data is superior to administrative data for identifying medical errors. The NLP approach, she noted, may not catch on with hospitals right away, because "it's not a small investment."
One alternative is the IHI "trigger tool," a method of measuring adverse events that requires hospitals to abstract manually about 20 to 50 charts a month. After several months, Haraden said, the hospitals build a "solid baseline" they can use to measure their progress on adverse events per 1,000 patient days, adverse events per 100 admissions, and percent of admissions with an adverse event.
As for using NLP for prospective review during inpatient stays, she agreed that that is an advantage. But some hospitals use other forms of computer surveillance to do prospective review, she said.
NLP has been used for some time to extract medical terms related to billing from electronic medical records. Computer-assisted coding software is mostly used in outpatient practices, as well as radiology and pathology departments of hospitals, but is starting to spread further on the inpatient side.
Since the IBM Watson supercomputer won "Jeopardy," hopes have been raised that NLP will eventually be able to aid doctors in clinical documentation. For example, Nuance, the leading vendor of speech recognition software, recently partnered with the University of Pittsburgh Medical Center to develop its "clinical language understanding" system to the point where it can convert dictation into discrete data in an EHR. Nuance is also working with IBM to apply its Watson technology to healthcare.
Many other researchers are tackling bits and pieces of the NLP puzzle. The Journal of the Medical Informatics Association devotes the majority of its September issue to papers about new developments in the field.
Find out how health IT leaders are dealing with the industry's pain points, from allowing unfettered patient data access to sharing electronic records. Also in the new, all-digital issue of InformationWeek Healthcare: There needs to be better e-communication between technologists and clinicians. Download the issue now. (Free registration required.)
About the Author(s)
You May Also Like