Kimball University: Using Regular Expressions for Data Cleansing and Standardization
Are you struggling to cleanse or standardize company names, phone numbers or other data in your warehouse? Here's how to use regular expressions to improve data matching, parse complex string patterns and increase analytic accuracy.
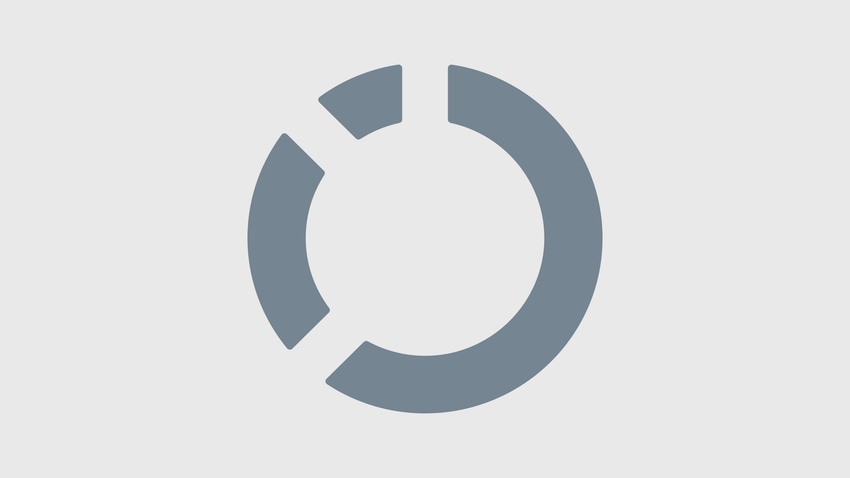
|
Data quality is one of the biggest challenges for many data warehouse and business intelligence (DW/BI) system managers. One common problem, especially with free-form entry data, is that the structure of the data is not in a standard form. For example, telephone numbers in the USA are often standardized to the pattern (999) 999-9999. You typically standardize the contents of a column in order to increase the likelihood of a match when users query or when you are doing data integration comparisons. This article describes an approach you can program directly into your ETL processing for simple- to moderately-complex data cleansing and standardization tasks.
Kimball Group recently worked on a Web-based, free-form entry registration system for an educational organization. The system works fine for processing the organization's registration transactions. As long as the person's name is on the registration list, they have a badge and course materials. However, free-form entry data is not so good for analytics. In this case, one useful analysis involves seeing how many students have been to more than one class. When new data is loaded into the data warehouse from the class registration system, the ETL system compares columns in the incoming records with existing records to see if any of the new registrants may have registered for a previous class. If the contents of these columns have not been standardized, they are less likely to match. The following examples of the Company_Name column from the source registration data illustrate this problem.
RegID | First_Name | Last_Name | Company_Name | Reg_Date |
507 | Craig | Nelson | Dataplace, Inc | 6/7/2008 |
515 | Danny | Davinci | Organics Inc. | 6/14/2008 |
516 | Jenny | Smith | Organics Inc | 6/14/2008 |
580 | Tim | Little | BVSG, Inc. | 7/31/2008 |
591 | Matthew | Adams | IncDot Incorporated | 9/8/2008 |
596 | Candy | Graham | Vencinc,Inc | 8/15/2008 |
617 | Jenny | Smith | Organics | 9/14/2008 |
Regular Expressions to the Rescue
A regular expression (RegExp) is a string matching pattern based on the regular expression syntax (Wikipedia offers a thorough description of the "regular expression" syntax). A fairly short RegExp pattern can be used to match many variations of a given pattern. In the example above, we'd like to clean up Company_Name so we are more likely to find matches as new registrations are loaded. Of course, the first approach to take when dealing with this problem is to try and get the source system to capture correct data in the first place. When this doesn't work, you will need to deal with the problem in your ETL system.
Matching is all about probabilities. You can never be 100 percent certain that two entries are the same people. In the table above, the last record is for a student who had registered before. In this case, we can match on First_Name and Last_Name, but there may be more than one person named Jenny Smith in the database. In order to improve our confidence in this comparison, we'll also match Company_Name. In this case, our match won't be exact because Jenny left off the "Inc." when she registered the second time.
Basic Operators
Regular Expressions have a full set of matching operators and abbreviations that work like the simple search string operators in SQL ( %, ? and *) or in Windows Search. Here are the RegExp operators we will use to clean up Company_Name:
Operator | Function |
* | Optional - match zero or more occurrences of the preceding string |
| | Or (Alternation) - match the string on one side of the operator or the other (can have multiple ORs in a group) |
\ | Escape - when used with an operator means treat the next character as a literal - e.g. \* means match the "*" character \ when used with certain regular characters represents a control character |
\t | Tab character |
\b | Word boundary |
( ) | Substring grouping |
Finding the Occurrences of "Inc"
Because there are several versions of "Inc," we need a RegExp that is flexible enough to find them all without including any false matches. RegExp engines always move in a forward direct from the beginning to the end of the string being searched. If we start our expression based on the first example, our initial RegExp looks like this (not including the quotes):
", Inc"
This won't match the second row because it doesn't start with a comma and it has a period at the end. By making the comma and period optional, RegExp now looks like this:
",* Inc\.*"
This combinations of operators looks for zero or more commas followed by a space followed by the letters Inc followed by zero or more periods. The period character itself is a RegExp operator meaning match anything, hence the backslash, which is an escape character changing the meaning to match the literal period character.
At this point, our RegExp will match the first four rows, but not the next two. We can extend it to match the fifth row by adding the full string " Incorporated" onto the front of our RegExp with an OR operator like so:
" Incorporated|,* Inc\.*"
The order of this is important. The full word "Incorporated" needs to come first, otherwise the RegExp engine will only match the "Inc" substring to the first three letters of Incorporated. The space in front of "Incorporated" means the pattern will match with the example, but it will not pick up a leading comma. We'll fix this next.
Now we are left with one final challenge in the test data: the "Inc" with the missing space after the comma in the sixth row. In our current pattern, the comma is optional, but the space is not. If we make the space optional as well, the pattern will over-match and return hits like the "inc" in the company name "Vininc" in the first row. A more targeted pattern needs to include an OR between the comma and space sub-pattern and the comma only, like this:
" (,* |,)(Incorporated|Inc\.*)"
We moved things around a bit in this version. The pattern is now in two sections, marked by parentheses. The first looks for the start of the target string: a comma and space, a space, or just a comma. The second section looks for either "Incorporated," or "Inc" followed by an optional period. The Final Results
This simple expression will match six different ways to type incorporated, plus differences in spacing. The ETL process would use it in a regular expression Replace function to add a standardized version, like ", Inc.", or replace it with an empty string, like the following results set:
Company_Name_Standardized
Dataplace
Organics
Organics
BVSG
IncDot
Vencinc
Organics
Not only does this standardization increase the likelihood of a match, it also improves the analytics. A count of registrations by the standardized Company is much closer to reality. This result is still not perfect; you might be combining companies that are not the same. Remember, matching is all about probabilities.
Where Can You Use RegExps?
Regular expressions can be used to help standardize any text or number column. You can also use them to extract substrings, like the search string component of a complex URL. There are examples of regular expressions to standardize or parse out most common data entry problems. This one works on United States phone numbers (\d stand for any digit):
"(\d{3})([-\ )]|\.)*\(*(\d{3})([-\ )]|\.)*(\d{4})"
You can find regular expression engines in most development toolkits, such as Microsoft's .Net regular expressions library (System.Text.RegularExpressions class), the Java JDK, and in many scripting languages like PHP, Python, and PowerGREP. Many UNIX utilities have regular expression engines built in as well. Your ETL tool may even offer direct access to regular expressions as part of its toolkit. The free version of EditPad Pro is a nice tool for interactive development and testing of your RegExp patterns.
If you have big data integration problems there are more sophisticated tools to do matching, including name and address lookups, fuzzy matching algorithms, and standardization libraries. However, if you have a relatively small data cleansing or standardization problem, or need to parse out complex string patterns, regular expressions can be a big help.
About the Author(s)
You May Also Like