Streaming Analytics: Government Must Step Up
By blending static big data with streaming analysis, agencies will get insights they've never had.
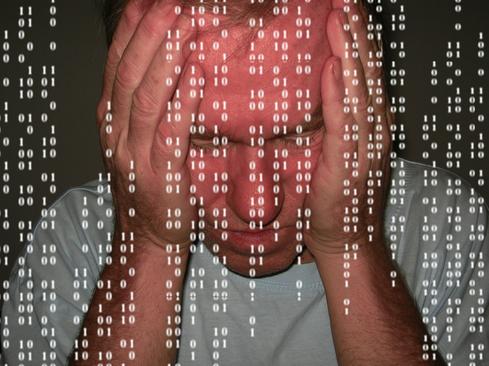
20 Great Ideas To Steal In 2015
20 Great Ideas To Steal In 2015 (Click image for larger view and slideshow.)
As government agencies continue to embrace big data, many have only taken the first step, such as implementing a Hadoop or NoSQL database. While these databases may offer a more efficient or cost-effective means to process large data sets, it's still static data. On its own, Hadoop doesn't offer a means for real-time decision-making.
With the narrowing decision windows that agencies face in their efforts to combat fraud, waste, abuse, and cyber-security threats, it's critical that government augment static big data repositories with real-time streaming analytics.
New streaming analytics tools that weren't available until recently stand to deliver the real-time, perishable insights agencies need to take preventative action, and not just react after the fact when data has lost value. They need systems designed to process high-velocity data flows from innumerable real-time data sources such as the Internet of Things, mobile apps, sensors, clickstream, and even transaction data that has largely been untapped by most agencies. They must blend real-time data with static data in order to dramatically enhance their real-time decision-making capabilities.
Once the technology is in place, two common data categories are necessary for collection: static and streaming. Static data is stored in a variety of ways, from traditional database or data warehouse to Hadoop clusters or NoSQL databases. The analysis is run on this stored data. Streaming data refers to real-time data -- information pouring in from sensors, devices, or social media that is not worth storing due to its perishable nature. For instance, if the data you gather from CAC smart cards tells you there's a suspected insider threat currently in flight, that data's only relevant for immediate apprehension for a short window of time, while the person is still on the premises.
[ See how UPS uses near real-time data analysis to make better logistics decisions. ]
In order to maximize the benefits of all of this data, agencies must be able to run analytics on both their static and streaming data. Not only can this be done, it must be done to give government agencies a more complete analysis and a more holistic view of the current state of each agency, which can be referred to as situational awareness.
If an agency has an existing Hadoop implementation where analysis is running on an hourly, daily, or weekly basis, streaming analytics can be leveraged to "color" the data with contextual information from those static feeds. In other words, additional information can be added to the feed data to provide more context. For example, if the static analysis revealed Joe might be involved in activities associated with insider threats, streaming analytics could show Joe's location, the machines on which he is logged in, and past commands issued on those machines for the session.
Another example of the value of blending historical and real-time data comes into play during a cyber-attack. Typically during an attack, agencies are flooded with data from a variety of sources. Much of this data is irrelevant, so an agency needs an effective way to sift through the data to quickly identify the abnormality. That requires correlating data from a variety of sensors, log files, and databases and merging that information with historical comparison data to stop the attack and provide insights into the attempted breach.
This approach to blending historical and real-time analysis to take proactive measures is not new, but has been difficult due to the lack of tools capable of the needed large-scale ingestion of data. Agencies must be careful of the products they choose and the architectures they put in place for their analysis to support such scale. They will need to rethink their business processes in order to fully utilize these new real-time insights and build on product foundations that will carry them forward. The safest way to tackle this effort is to identify the industry leaders in the space, such as those in Gartner's magic quadrant, and to take an iterative approach. This means building out their static big data analysis using industry standard technologies such as Hadoop, then introducing real-time analytics tools that integrate well with those technologies on small projects, and building from there.
With real-time big data analytics, agencies can pick out the needles as the data haystack is streaming by. They do not have to worry about taking all of the irrelevant data, putting it into storage, running an analysis, waiting, and then redoing that over and over again. Instead, in real-time, the agency can pull information out of the streams and make a decision while they still have a window of relevance. This allows them to take proactive action on the data. By exploring the merging of both historical and streaming data, agencies can gain the ability to analyze data to optimize operations, mitigate risks, and make decisions in real-time.
About the Author
You May Also Like