The State Of Social Media Analytics
Tools to help enterprises sift through mountains of unstructured data to find nuggets of useful information are still immature, says Deloitte expert.
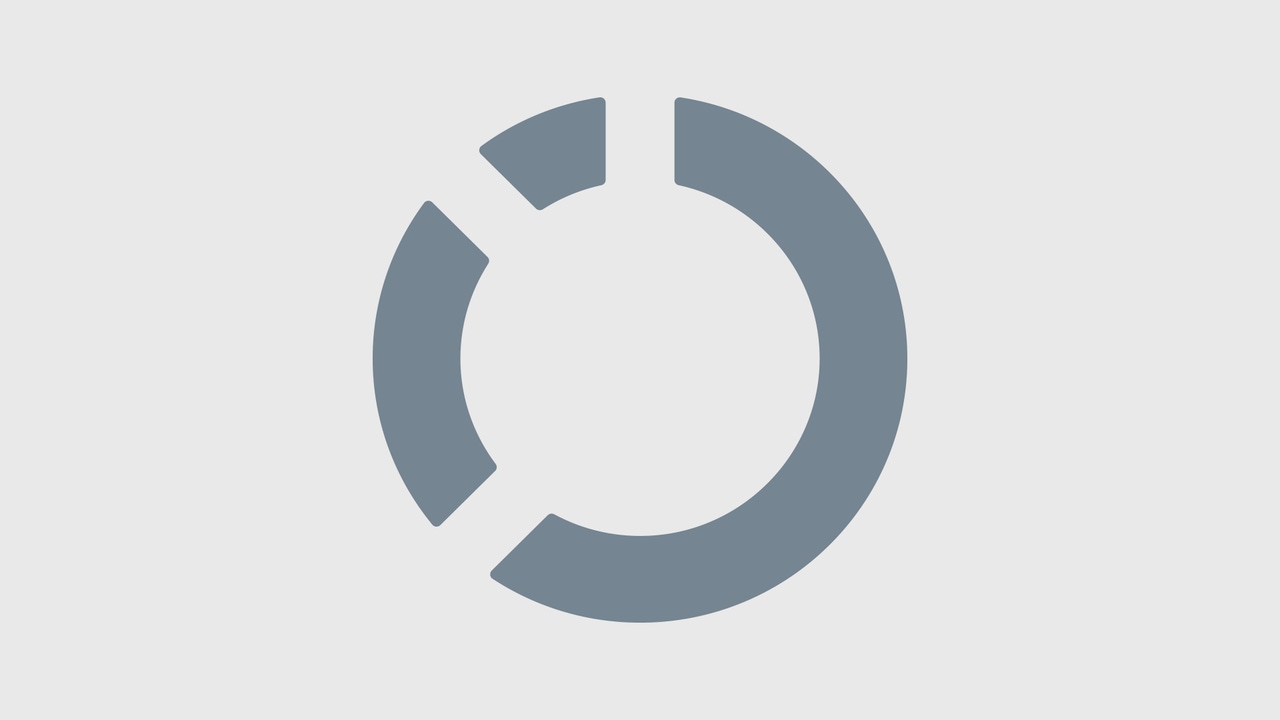
14 Leading Social CRM Applications
Slideshow: 14 Leading Social CRM Applications(click image for larger view and for slideshow)
Considered along the same spectrum as business analytics, from basic information management and business intelligence to predictive analytics, social media analytics is both advanced and just getting started, according to a Deloitte Consulting expert.
"From my perspective it's infantile--the mere fact that you have so many little start-ups, from my point of view, reflects that," John Lucker, leader of leads Deloitte's Advanced Analytics & Modeling practice said in an interview. Many of these startups have good ideas, but their efforts haven't yet coalesced, he said.
The technology for analyzing social networks is advanced in the application of "big data" analysis techniques that some organizations are applying to analyze sentiment about their brands or divine consumer marketing trends and opportunities. Still, the products to support these requirements are still young and the understanding of what to do with the data is still scarce, Lucker said.
Lucker offered his thoughts, not as a social analytics specialist per se, but as someone with deep experience in corporate analytics who is increasingly fielding questions from clients trying to understand the applications of social media data. Before joining Deloitte in 2000, he developed his data analysis and marketing prowess mostly through work with banks and insurance companies in a career stretching back to the early 1980s.
If social media analytics is immature, that only means that it lags slightly behind the use of analytics in other contexts, he said. "None of the companies I work with in the Fortune 1000, none of them are really mature in the use of analytics. There are pockets of greatness and brilliance in most companies, but they are typically just pockets."
As Deloitte classifies things, the range of maturity for corporate analytics starts with information management (simplify organizing data for later recall), progresses through business intelligence (flexible reporting on historical data), performance management (systematically applying data to improve corporate performance), and advanced or predictive analytics (making credible predictions based on the data).
Social media data is a little different in that we don't control how it is created or recorded on the Web and have to impose structure on it retroactively. Just parsing out all the social media mentions a company wants to track takes a lot of computing horsepower. But relatively few organizations have progressed beyond the basics of historical reporting on that data to making useful predictions, Lucker said.
"From the technology side, it's more of a classic, pragmatic story," Lucker said, but the more you move toward advanced analytics, the more the focus shifts from the technology itself to knowing how to use the technology and how to interpret the data.
For example, you have to decide how far to trust the data coming from an uncontrolled medium. If you were to mine the comments sections of newspaper websites dealing with the budget, the Tea Party, the debate over lifting the debt limit, or the recent Standard & Poor's downgrade of U.S. Treasury bonds, you could certainly get a sample of consumer sentiment, but how representative is it? How many of the people posting are actually lobbyists and their minions? How many are professional complainers and cranks?
"It's not a random sense of people's sentiment," Lucker said. "How do you normalize unstructured data that is inherently skewed or biased?" There are data cleansing strategies for filtering out suspect data, but you could wind up eliminating some legitimate content in the process. "You may be creating an equally biased data set based on what you think is the norm. That's a much different problem from dealing with corporate data such as customer information or pricing information where it's much easier to statistically understand bias."
Meanwhile, it's important not to overestimate the accuracy of the data or the quality of the analysis based on it, Lucker said. Web marketers like Amazon.com can do a lot to divine our tastes by watching what we buy, but even they can be confused when we use their service to buy a gift for someone else that doesn't reflect our tastes at all. The challenge is even greater for the marketer working with a social media feed, where some of a consumer's posts may be random comments or inside jokes between friends that don't reflect their real preferences at all.
"We should be looking for ways of validating the signals that come from the data against secondary or tertiary data sources," he said. "I would never suggest to you that the right way to do something is don't do it at all until you can get it perfect. I'm just saying that putting too much weight on stray comments, or stray data signals, could be dangerous."
Attend Enterprise 2.0 Santa Clara, Nov. 14-17, 2011, and learn how to drive business value with collaboration, with an emphasis on how real customers are using social software to enable more productive workforces and to be more responsive and engaged with customers and business partners. Register today and save 30% off conference passes, or get a free expo pass with priority code CPHCES02. Find out more and register.
About the Author
You May Also Like