16 Machine Learning Terms You Should Know
Artificial intelligence and machine learning are gaining enterprise attention. Here's an overview of some essential terms you can share with your team and executives.
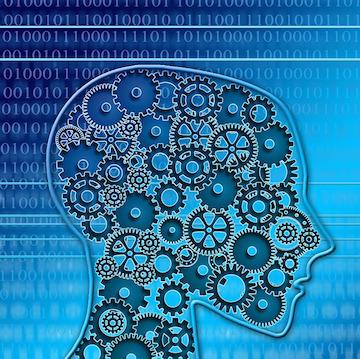
Advanced analytics is heating up. AI, machine learning, deep learning, and neural networks are just some of the terms we hear and should know more about. While most of us will never become statisticians or unicorn data scientists, it's wise for us to understand some of the basic terms, especially since we'll be hearing a lot more about machine learning in the coming years. Here are a few terms we should all know from some sites that have much more to offer:
Algorithm - a step by step procedure for solving a problem.
Attribute - a characteristic or property of an object.
Classification - to arrange in groups.
Clusters - groups of objects that share a characteristic that is distinct from other groups.
Correlation - the extent to which two numerical variables have a linear relationship.
Deep Learning - An AI function that imitates the workings of the human brain.
Decision Tree - a decision support tool that uses a tree-like graph or model of decisions and their possible consequences, including chance event outcomes, resource costs, and utility.
Natural Language Processing (NLP) - the automatic (or semi-automatic) processing of human language.
Neural Networks - a series of algorithms that attempts to identify underlying relationships in a set of data by using a process that mimics the way the human brain operates.
Normal Distribution - symmetrical distributions that have bell-shaped density curves with a single peak.
Outlier - an observation that lies an abnormal distance from other values in a random sample from a population.
Regression - a statistical process for estimating the relationships among variables.
Statistical Model - a formalization of relationship between variables in the form of mathematical equations.
Supervised Learning - accomplished with training data that includes both the input and the desired results.
Unsupervised Learning - accomplished with training data that does not include the desired results.
What Terms Would You Add?
This list above does not represent all terms that apply to machine learning because I have limited space and it's more fun to continue the discussion.What are some of your favorites in the comments section.
About the Author(s)
You May Also Like