Build Reliability into Autonomous and Intelligent Systems
When building and using autonomous and intelligent systems, it’s important to know they’re behaving reliably, because if things go wrong, they can do so at scale, fast.
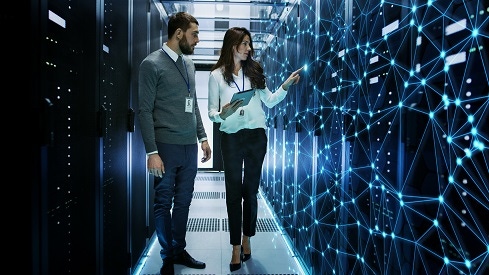
Organizations are racing to implement artificial intelligence, focusing on the potential benefits, but not necessarily the risks. When building and using self-learning systems, whether autonomous or not, enterprises should do what they can to ensure they’re functioning reliably. Otherwise, those systems can’t be trusted. Worse, the unexpected outcomes could undermine the trust your company has built with shareholders, partners, customers and employees.
If you consider self-learning systems just another technology, your IT risk management strategy probably reflects that. Following are a few important points to consider about need and importance of building reliability into autonomous and intelligent systems.
1. It’s a new form of liability. System reliability has traditionally been measured by the degree to which actual outcomes align with intended outcomes. In a deterministically-programmed world, a given input should yield a given output. However, in probabilistic systems, the outcomes aren’t so black-and-white. Software developers, IT, and business leaders should consider the many ways autonomous and intelligent systems can be used and abused, as well as what can go “right” and “wrong” with the system.
If something does go wrong, who will be held responsible for unintended consequences? The person who designed the system? The person who wrote the algorithm? The person who used the system? This very question of accountability is being explored now by industry groups, lawyers, technologists, and lawmakers.
“With anything that's new and evolving, all the checks and balances aren't in place. For example, with self-driving cars, how do you program it in terms of a crash whether it hits the tree and injures the passenger or hits a person on the street?” said Sanjay Srivastava, chief digital officer at global professional services firm Genpact. “As humans we have variances. When you put those variances into a system and there’s a problem with it, it will be amplified all over the place.”
According to Frank Butendijk, distinguished VP and Gartner fellow, there are three parts to the responsibility discussion: Designers, users, and machine agency – that is, a machines’ responsibility for its autonomous action.
“Right now, we recognize human agency and organizational agency, but we need to have a discussion about machine agency,” said Butendijk.
The modern workforce is humans and machines. Organizations are responsible for the actions of both, so it’s in the best interest of the business to put a premium on the reliability of the autonomous and intelligent systems they’re using.
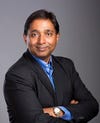
Sanjay Srivastava
2. The problem is multi-disciplinary. It’s important to know what software is doing, including why algorithms are behaving the way they are, what potential edge cases exist, whether bias exists and if so, the potential impact(s) of the bias. The point some organizations are missing is that these are not just engineering problems, they’re business problems.
Risk management (and as part of that system reliability) should begin at the earliest stages of autonomous and intelligent system design and use, so when the unexpected arises, the organization is better prepared to manage the potential fallout. Companies should have a multi-disciplinary group(s), including people from the legal team and chief ethics officer (or functional equivalent) to discuss the potential opportunities and benefits of self-learning systems, their potential use cases and associated risks. The different educational and professional backgrounds help organizations perceive the benefits and risks of autonomous and intelligent systems more holistically than is possible by relying on one type of expertise (such as engineering) alone.
3. Bias can backfire. Humans are highly biased creatures, so the data they create tends to reflect those biases. For example, social media data tends to be very negative, so if a chatbot is trained on that data, it will reflect that negativity as demonstrated by Microsoft’s Tay bot which morphed from a friendly bot to a racist bot in less than 24 hours.
Right now, people still tend to think about data in terms of a problem they want to solve, but not whether the data fully represents the environment in which they’re going to deploy the system. As a result, facial recognition systems built with Caucasian biases are unable to recognize people of color as reliably. Similarly, Amazon’s HR AI pilot systematically discriminated against women.
4. There’s a network effect few have considered. Autonomous and intelligent systems have outside dependencies, meaning they rely on other systems and components to do their magic. One or more of those dependencies might be on another autonomous or intelligent system or a set of such systems.
{image 3}
There’s a public outcry about transparency as it relates to deep learning systems that are unable to explain their results or the reasoning that lead to the result. In other words, no one truly understands how the system operates. Now, connect that system to other such systems and the level of uncertainty could grow exponentially. Few have considered the network effect yet because they’re implementing systems narrowly to solve specific problems.
5. Top-down and bottom-up support are necessary. Company leadership has a responsibility to define and enforce the values and principles that guide the company and the development of its products or services. Those values and principles should be kept in mind when automating decision-making and enabling self-learning systems in addition to any other requirements such as regulatory compliance. However, there is often a big disconnect between what the organizational leaders envision and what is implemented several layers below.
Conversely, employees should be encouraged and incentivized to identify issues that impact the reliability of (and thus trust in) autonomous and intelligent systems because they’re helping to manage potential risks and their associated liabilities.
6. Reliability is a multi-faceted concept. Traditional product considerations apply to autonomous and intelligent systems, meaning if they’re doing what they’re supposed to do, they’re safe as appropriate in the context and secure. However, autonomous and intelligent systems, particularly the self-learning varieties, also involve other considerations including:
Transparency – can the system explain its result and the reasoning that lead to the result?
Failsafe – if something goes wrong, can a supervisory AI identify the pattern early enough to minimize the results, can the system shut down, or can the system notify an agent when its operations are starting to drift out-of-bounds?
Real-world benefit – is the system delivering the benefits for which it was designed in the real-world?
User alignment – are the system’s capabilities within the end user’s level of competence or does the system require specific training to minimize the potential for negative consequences?
Responsibility – who will be held accountable if something goes wrong?
Bias – what bias exists in training data? What was done about it?
Data quality – poor quality data can lead to spurious results.
Crisis management – if something went wrong, what would it likely be? What are the edge cases? Assuming Scenario X happened, what would the company’s response be?
Bottom line
Autonomous and intelligent systems aren’t inherently reliable. To make them reliable, their creators and users need to understand their capabilities and limitations, as well as the potential benefits and risks.
The problem is that most enterprises don’t know how to think through the risk part of the equation yet because they are myopically focused on the potential benefits. However, as autonomous and intelligent systems become more commonplace, errors will occur that negatively impact individuals and groups which will lead to a new generation of lawsuits and new regulations.
[For more about artificial intelligence and machine learning, check out these recent articles.]
On Sale: AI Lessons from the Retail Industry
How Automation Empowers the CIO to Think Outside the IT Department
6 Tech Trends for the Enterprise in 2019
About the Author
You May Also Like