Deloitte: 5 Trends That Will Drive Machine Learning Adoption
Machine learning isn't as widely adopted as some may think, mainly because there are serious barriers to adoption. Researchers are making progress in reducing those barriers.
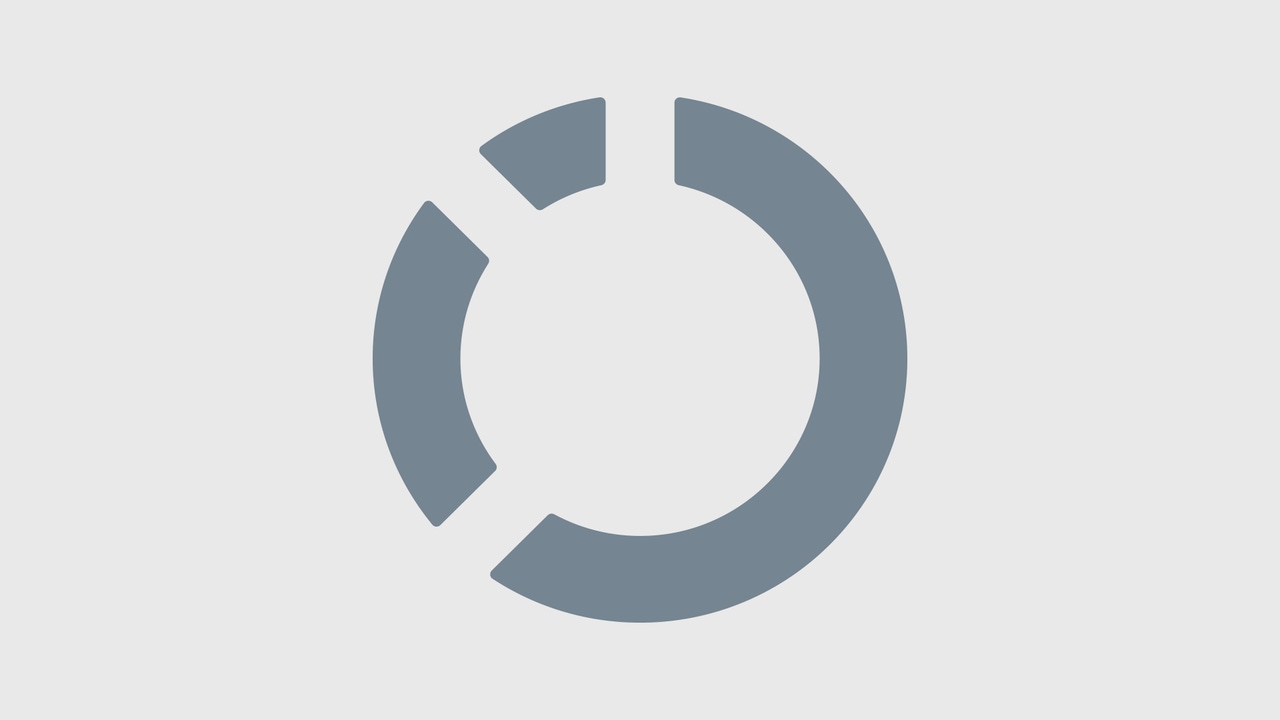
Companies across industries are experimenting with and using machine learning, but the actual adoption rates are lower than it might be seem. According to a 2017 SAP Digital Transformation Study, fewer than 10% of 3,100 executives from small, medium and large companies said their organizations were investing in machine learning. That will change dramatically in the coming years, according to a new Deloitte report, because researchers and vendors are making progress in five key areas that may make machine learning more practical for businesses of all sizes.
1. Automating data science
There is a lot of debate about whether data scientists will or won't be automated out of a job. It turns out that machines are far better at doing rote tasks faster and more reliably than humans, such as data wrangling.
{image 1}
"The automation of data science will likely be widely adopted and speak to this issue of the shortage of data scientists, so I think in the near term this could have a lot of impact," said David Schatsky, managing director at Deloitte and one of the authors of Deloitte's new report.
Industry analysts are bullish about the prospect of automating data science tasks, since data scientists can spend an inordinate amount of time collecting data and preparing it ready for analysis. For example, Gartner estimates that 40% of a data scientist's job will be automated by 2020.
Data scientists aren't so sure about that, and to be fair, few people, regardless of their position, have considered which parts of their job are ripe for automation.
2. Reducing the need for training data
Machine learning tends to require a lot of data. According to the Deloitte report, training a machine learning model might require millions of data elements. While machine learning requirements vary based on the use case, "acquiring and labeling data can be time-consuming and costly."
One way to address that challenge is to use synthetic data. Using synthetic data, Deloitte was able to reduce the actual amount of data required for training by 80%. In other words, 20% of the data was actual data and the remaining 80% was synthetic data.
"How far we can go in reducing the need for training data has two kinds of question marks: How far can you reduce the need for training data and what characteristics of data are most likely minimized and which require massive datasets?" said Schatsky.
3. Accelerating training
Massive amounts of data and heavy computation can take considerable time. Chip manufacturers are addressing this issue with various types of chips, including GPUs and application-specific integrated circuits (ASICs). The end result is faster training of machine learning models.
"I have no doubt that with the new processor architectures, execution is going to get faster," said Schatsky. "[The chips] are important and necessary, but not sufficient to drive significant adoption on their own."
4. Explaining results
Many machine learning models spit out a result, but they don't provide the reasoning behind the result. As Deloitte points out, business leaders often hesitate to place blind faith in a result that can't be explained, and some regulations require an explanation.
In the future, we'll likely see machine learning models that are more accurate and transparent, which should open the door for greater use in regulated industries.
[Deloitte also recently discussed 9 AI Benefits Enterprises Are Experiencing Today.]
"No one knows how far you can go yet in terms of making an arbitrary neural network-based model interpretable," said Schatsky. "We could end up hitting some limits identifying a fairly narrow set of cases where you can turn a black box model into an open book for certain kinds of models and situations, but there will be other scenarios where they work well but you can't use them in certain situations."
5. Deploying locally
Right now, machine learning typically requires a lot of data and training can be time-consuming. All of that requires a lot of memory and a lot of processing power, more than mobile and smart sensors can handle, at least for now.
In its report, Deloitte points out there is research in this area too, some of which has reduced the size of models by an order of magnitude or more using compression.
The bottom line
Machine learning is having profound effects in different industries ranging from TV pilots to medical diagnoses. It seems somewhat magical and somewhat scary to the uninitiated, though the barriers to adoption are falling. As machine learning becomes more practical for mainstream use, more businesses will use it whether they realize it or not.
"[The five] things [we identified in the report] are converging to put machine learning on a path toward mainstream adoption," said Schatsky. "If companies have been sitting it out waiting for this to get easier and more relevant, they should sit up instead and start getting involved."
About the Author
You May Also Like