Diversity’s Crucial Role in AI
Your board and your CEO have been clamoring for artificial intelligence, and now you have AI technology. But what if what your AI is telling you is wrong?
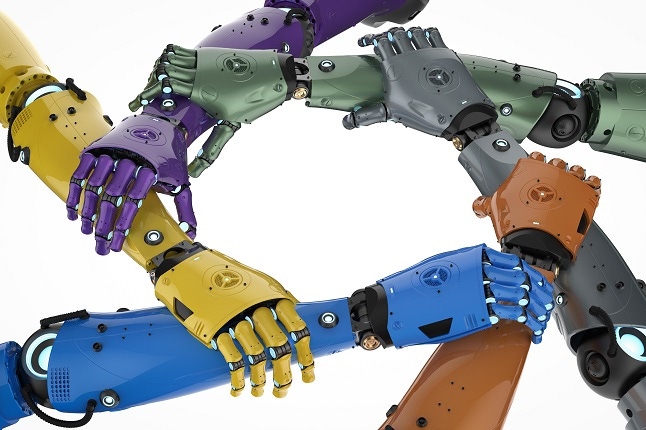
Last year, McKinsey reported that more than half of the organizations itsurveyed (52%)acknowledged that more than 5% of their entire technology budgets were being committed to artificial intelligence (AI). This was before the November 2022ChatGPT product launchthat propelled both investors and companies into a near-frenzy for capitalizing on AI.
What organizations hope to get from AI is fast and accurate decision making on critical issues, the ability to automate internal and external operations, and faster times to market on decisions that concern marketing, selling, and producing new products and technologies. However, if you purchase or subscribe to a turnkey and pre-configured AI engine, there is also that lurking question as to whether the outcomes the AI produces will be true for your specific company. And, if they’re not accurate, how will you know and what will you do?
AI’s Potential Achilles Heel: A Lack of Diversity
In 2023,Women in AI, an international organization advocating for greater inclusiveness of women in the AI industry, partnered with a leading research team at Omdia, and AI Business, a global Informa media portal. Collectively, they developed a survey of AI leaders and employees. The survey revealed that only four percent of men and women responding believed that their AI companies had achieved a 50:50 employment equity between men and women.
Clearly, diversity in hiring and leadership is a challenge for AI companies. That also is a potential risk for non-AI companies that are buying and using AI solutions.
For the companies investing in AI, this risk comes when they employ AI learning models providing outcomes that may be inaccurate, which could lead to erroneous company decisions. The potential for inaccuracy arises because the data collected and the employees who developed the AI were not sufficiently broad and diverse in their perspectives.
Here is a recent example:
In 2022, the Wonkwang University Hospital in Korea captured data from 5,628 Covid patients. The goal of the study was to train an AI model so it could accurately predict what the severity of Covid symptoms would be for a variety of patients so that each patient could be medically treated for the best results.
What theuniversity hospital foundwas that when men and women worked together on AI model training and algorithm development, the accuracy of symptom severity prediction was the greatest. However, accuracy declined when only men worked on the AI models and algorithms for female patients; or when only women developed AI models and algorithms for male patients. The Wonkwang study concluded that a diverse set of data and a diverse AI team were needed to produce the greatest level of accuracy in AI results. That most likely was because greater diversity of data and staff brought with it many more perspectives and ideas to assess data and develop algorithms in myriad ways, leading to the best results.
The AI Impact on IT and Companies
What studies like Wonkwang’s tell companies interested in implementing AI is that they will need a diversity of data and AI workforce to avoid AI bias and inaccurate results.
How can you go about reducing this risk of bias?
Understand the sources of your data.If you’re purchasing or subscribing to a pre-configured, turnkey AI solution from a vendor, your request for proposal (RFP) should include an evaluation of the data sources that the vendor is using. That is so you ensure that the data sources are sufficiently large and variegated to preclude the possibility of bias from having limited data sources.
It’s also helpful to ask your prospective vendor what it is doing internally to use a diverse AI workforce on the analytics and algorithms that it develops.
Use large amounts of quality data to feed your own AI system.It’s important to get business users, their company's database team and data science group together to review the potential data sources for your AI, and to assess the credibility and reliability of each data source. These sources and their data should be vetted for the comprehensiveness and inclusiveness of their data (e.g., Is the data coming from the US only, or from worldwide sources?). In some cases, including worldwide data might not matter, such as if you’re only doing a study on the US, but in broader use cases, it will.
Continuously monitor AI systems for accuracy. The gold standard for AI system accuracy is that it conforms with the opinions of subject matter experts in the field at least 95% of the time. In other cases, such as when AI is just being used to get a sense of a general trend, an accuracy goal of only 70% might be fine. In both cases, set and maintain appropriate metrics.
Regularly monitor AI systems to maintain accuracy metrics. If accuracy levels begin to fall, it might be time to refresh the data, look for new data sources, or fine-tune algorithms or AI models.
Employ a diverse workforce for your AI project.Almost every company today has some level ofdiversity, inclusion and equity(DEI) initiative, but when I talk with IT leaders, the common perception is that “this is a human resources thing.”
DEI is not just an HR issue.
When it comes to accuracy in AI systems and IT’s ability to deliver quality AI, diversity of AI data and the AI workforce are critical ingredients if you want to achieve accurate results.
What to Read Next:
Digital Humans: Fad or Future?
Read more about:
Diversity, Equity, and Inclusion (DEI)About the Author(s)
You May Also Like