Enterprises are White Knights in Battle to Avoid AI Winter
It is up to enterprise organizations, not just academia, to maintain artificial intelligence's momentum when it comes to innovation and adoption.
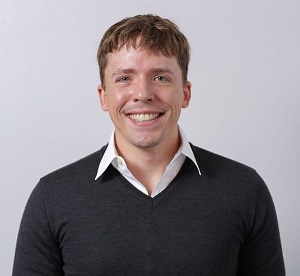
Despite how groundbreaking artificial intelligence may seem today, it isn’t a new phenomenon. The technology and the concept have existed since the 1950’s. It was 1956 when AI was first studied at the Dartmouth Summer Research Project on Artificial Intelligence. Around the 1960’s and 1970’s, AI advanced rapidly. We saw the invention of anthropomorphic and autonomous robots. By the 1980s, the world was excited, hooked, and hungry for more.
But the pace of innovation soon decelerated. Companies that had spent over a billion dollars on AI programs known as “expert systems” saw their investments quickly deemed irrelevant with the rise of powerful desktop computers. Promises of machines that could conduct conversations and perform human-like reasoning were 10 years overdue. AI’s performance could no longer satiate the public’s appetite. With a lack of interest and a corresponding reduction in funding, we entered an “AI Winter” that lasted until the 2000’s.
In this new century, there has been an explosion of AI innovation. Google solved search with machine learning, then acquired a company called DeepMind and found that their deep learning solutions could translate between languages better than, and in a fraction of, the time as, models that had been trained for over a decade. An AI beat the world champion at chess, and then Go. The Turing Test was passed. GANs (generative adversarial networks) are synthesizing new text, videos and images, and reinforcement learning promises to accelerate AI development.
The applications seem endless. With recent news of self-driving cars, barista robots, cashier-less grocery stores, and Elon Musk’s fear of an AI apocalypse, people are once again excited about AI. But some argue that we are just a few unmet expectations away from another AI Winter. Most of these arguments focus on the limitations of AI in its march toward general AI, or the unlikelihood that the recent rapid pace of innovation in narrow AI can be sustained.
But we’ve come a long way since the 1970’s. The emergence of the Internet, high performance computing, and novel modeling techniques gives practitioners access to extraordinarily powerful tools, more powerful than what we had 50 years ago. And enterprises now have strategies to drive AI adoption, teams building differentiated AI models, and troves of proprietary data to power these efforts. This foundation sets AI up for unprecedented success in the coming decades.
So how do we gauge progress? Look to the enterprise, not academia. Though they don’t make for exciting headlines like breakthroughs in core AI research, applied enterprise applications are where the innovations in AI will be converted into real impact and value. Even if improvements in AI research came to a complete halt tomorrow, there are still trillions of dollars worth of unrealized value from machine learning and AI applications that currently exist. If they realize the full potential of applied AI, enterprises will emerge as the white knights in the fight against an impending AI Winter.
Consider a few recent examples of progress in the enterprise. Just as Steve Cohen was proclaiming that models will run the world, quantitative hedge funds were capping a decades long run to the top of Wall Street. Just a year later, financial firms like Stripe upgraded their fraud detection with machine learning to reduce fraudulent claims costs to an all-time low. As Google and Tesla paved the way for self-driving cars, multiple startups were acquired by major auto manufacturers to pioneer their own technology. And just recently, hospitals have begun to prove that AI-assisted physicians outperform experts operating alone.
Every sector of our economy will be impacted by AI. The question becomes: by how much? This is the beginning of the so-called second wave of AI innovation in which businesses leverage proprietary data to build differentiated models for value-added purposes.
These examples are just the tip of the iceberg. As companies mature in their AI adoption, there is a general AI roadmap that they will follow. First, companies will automate their business operations to drive bottom-line savings. Core processes related to finances, accounting, customer service, and supply chain management will be some of the first to make the shift. Second, companies will apply AI to address entirely new problems, creating new product categories that drive top-line revenue growth. Finally, companies will implement AI that makes such accurate predictions so cheaply that it will entirely disrupt and, in some cases, invert their own business models.
A classic example of this, and one cited in Prediction Machines: The Simple Economics of Artificial Intelligence, relates to the future of Amazon’s business. Once Amazon’s predictions for a user’s product preferences improve in accuracy, the company could begin shipping products to users before they buy (whereas today users buy and then Amazon ships). These are the cases in which AI becomes transformational.
This future is by no means inevitable. If companies deliver on implementation, they will realize this AI potential. In short, the only risk is execution risk. So how do we know if they’re on track?
We need to look for at least three indicators. Focus on technology adoption; have enterprises adopted leading technologies that help them attract, retain, and maximize the potential of their data scientists and machine learning engineers? Keep an eye on financial reporting; do companies properly account for and report on AI’s impact on their business on a regular basis, like AI leaders do today? Finally, observe research-to-applications lags; are companies adopting techniques that were popularized in research a half-decade or more prior to the application, as is somewhat typical of enterprise adoption curves?
Anyone worried about the next AI Winter should keep a close eye on the enterprise; what it proves is that we’re nowhere near exhausting the potential impact of AI.
Scott Clark is the co-founder and CEO of optimization-as-a-platform startup SigOpt. Scott came up for the idea of SigOpt while he was getting his PhD at Cornell. Scott has been applying optimal learning techniques in industry and academia for years, from bioinformatics to production advertising systems. Before SigOpt, Scott worked on the Ad Targeting team at Yelp leading the charge on academic research and outreach with projects like the Yelp Dataset Challenge and open sourcing MOE. Scott holds a PhD in Applied Mathematics and an MS in Computer Science from Cornell University and BS degrees in Mathematics, Physics, and Computational Physics from Oregon State University. Scott was chosen as one of Forbes’ 30 under 30 in 2016.
About the Author(s)
You May Also Like