GPUs Continue to Dominate the AI Accelerator Market for Now
As enterprises embark on AI and machine learning strategies, chip makers like NVIDIA, Intel and AMD are battling to become the standard hardware providers.
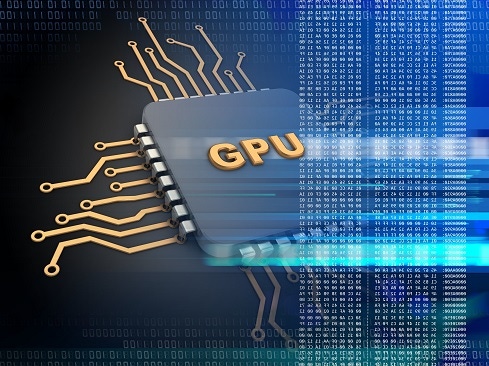
Artificial intelligence is becoming an integral feature of most distributed computing architectures. As such, AI hardware accelerators have become a principal competitive battlefront in high tech, with semiconductor manufacturers such as NVIDIA, AMD, and Intel at the forefront.
In recent months, vendors of AI hardware acceleration chips have stepped up their competitive battles. One of the most recent milestones was Intel’s release of its new AI-optimized Ponte Vecchio generation of graphical processing units (GPUs), which is the first of several products from a larger Xe family of GPUs that will also accelerate gaming and high-performance computing workloads.
GPU's AI accelerator dominance in the cloud
In AI hardware acceleration, NVIDIA has been the chip vendor to beat, owing to its substantial market lead in GPUs and its continued enhancements in the chips’ performance, cost, efficiency, and other features. Though NVIDIA has faced growing competition both in its core GPU stronghold and in other AI accelerator segments -- most notably, mobile, Internet of Things, and other edge deployments -- it has held its own in the AI chip wars and now appears poised for further growth and adoption.
Over the next several years, NVIDIA will still be the supplier to beat in chipsets optimized for a wide range of AI workloads, from training to inferencing and supporting deployments in cloud data center, enterprise server, and edge deployments. Several principal trends will buoy NVIDIA to continued dominance in AI hardware accelerators.
First, clouds will remain AI’s center of gravity. Hardware accelerator solutions supporting data center and server-based AI workloads will constitute the bulk of the opportunity through 2025, according to recent McKinsey projections. Model training will remain the predominant AI workload in the cloud for several years, though inferencing apps will steadily grow and more of these workloads will shift toward mobile, embedded, and other edge devices.
NVIDIA’s GPUs have established themselves as the principal platform for cloud-based training, and no other hardware acceleration technology seems likely to supplant GPUs in that regard over the coming 10 years. Nevertheless, edge-based inferencing will be the dominant growth segment of the AI opportunity going forward. McKinsey has predicted that the opportunity for AI inferencing hardware alone in the data center will be 2 times that for AI training hardware by 2025 ($9B-10B, vs. $4B-5B), and, in edge device deployments, will be 3x larger for inferencing vs. training by that same year.
The second trend in NVIDIA’s favor is that GPUs’ cloud dominance will give the tech a persistent role in edge applications. GPUs remain by far the most adopted hardware tech for cloud-based AI workloads. Liftr Cloud Insights has estimated that the top four clouds in May 2019 deployed NVIDIA GPUs in 97.4% of their infrastructure-as-a-service compute instance types with dedicated accelerators. AMD and Intel's rival GPU solutions are not likely to make a huge dent in NVIDIA’s market share through the middle of the decade.
NVIDIA is leveraging this cloud advantage into new edge opportunities, as evidenced by its recent announcements of high-profile partnerships for running GPU servers for AI workloads in cloud-to-edge computing environments for industry-specific, hybrid, and virtualized deployments. Even as rival hardware AI chipset technologies -- such as CPUs, FPGAs, and neural network processing units -- grab share in edge devices, GPUs will stay in the game thanks to their pivotal role in cloud-to-edge application environments, such as autonomous vehicles and industrial supply chains.
Last but not least, NVIDIA’s impressive industry-standard AI hardware acceleration benchmarks will give it a competitive advantage across the board. Most notably, the recent release of MLPerf AI industry benchmarks that show NVIDIA’s technology setting new records in both training and inferencing performance. MLPerf has become the de facto standard benchmark for AI training and, with the new MLPerf Inference 0.5 benchmark, for inferencing from cloud to edge.
NVIDIA’s recent achievement of the fastest results on a wide range of MLPerf inferencing benchmarks is no mean feat and, coming on its equally dominant results on MLPerf training benchmarks, no big surprise. As attested by avid customer adoption and testimonials, the vendor’s entire AI hardware/software stack has been engineered for the highest performance in all AI workloads in all deployment modes. These stellar benchmark results are just further proof points for NVIDIA’s focus on low cost and high-performance AI platforms.
NVIDIA's winning performance on the MLPerf benchmarks in all categories (data center and edge, training and inferencing) show that it is very likely to grow or at least maintain its market share predominance at the data center/server level while making considerable headway in edge-based solutions, in spite of dozens of competitors in that segment, including large and small, established and startup.
Edge inferencing market is wide open
However, NVIDIA will find it next to impossible to achieve anything approaching the near-monopoly status it has enjoyed with GPUs in the cloud as it pushes into edge inferencing applications.
In edge-based inferencing, no one hardware/software vendor will dominate. GPUs appear likely to remain limited to cloud and server-based AI applications. At the same time, various non-GPU technologies -- including CPUs, ASICs, FPGAs, and various neural network processing units -- will increase their performance, cost, and power efficiency advantages over GPUs for various edge applications.
Indeed, CPUs currently dominate edge-based inferencing, while NVIDIA’s GPUs are not well-suited for commodity inferencing in mobile, Internet of Things, and other mass-market use cases. McKinsey projects that CPUs will account for 50% of AI inferencing demand in 2025 with ASICs at 40% and GPUs and other architectures picking up the rest.
In edge-based AI inferencing hardware alone, NVIDIA faces competition from dozens of vendors that either now provide or are developing AI inferencing hardware accelerators. NVIDIA’s direct rivals -- who are backing diverse AI inferencing chipset technologies -- include hyperscale cloud providers such as Amazon Web Services, Microsoft, Google, Alibaba, and IBM; consumer cloud providers such as Apple, Facebook, and Baidu; semiconductor manufacturers such as Intel, AMD, Arm, Samsung, Xilinx, and LG; and a staggering number of China-based startups.
Though NVIDIA has shown impressive GPU performance benchmarks in various AI workloads, the technology’s potential adoption at the edge is constrained by its higher cost and lower energy efficiency than many alternatives.
Nevertheless, NVIDIA comes well-armed to the battle. Another significant milestone for NVIDIA in the inferencing market was its announcement of its forthcoming Jetson Xavier NX module. Due for general availability in March, Jetson Xavier NX module will offer server-class AI-inferencing performance at the edge along with a small footprint, low cost, low power, high performance, and flexible deployment. These features will suit the new hardware platform both for AI inferencing applications at the edge and in the data center.
As it pushes into edge deployments, NVIDIA will leverage its CUDA library, APIs, and ancillary software offerings as key competitive assets. These are used everywhere on the planet for the widest range of AI development and operations challenges, and NVIDIA will almost certainly develop the “model once, deploy anywhere” features of this data science workbench across its chipset portfolio. It’s no surprise that Intel is pursuing an equivalent strategy with its new OneAPI to simplify programming across its wider range of AI accelerator technologies, including GPUs, CPUs, and FPGAs.
As it steps up the battle in edge inferencing, NVIDIA will experience diminishing returns on any attempts to retreat to its core market of cloud-based AI model training. Though it rules the roost in that segment now, it will see its competitive advantage wane as AMD and Intel continue to enhance their rival GPU offerings for AI, high-performance computing, gaming, and other markets.
As the AI market evolves toward multi-technology accelerator architectures, competition is likely to shift toward data science workbenches that support development in a “model once, deploy anywhere” paradigm. Widely adopted abstraction layers such as Open Neural Network Exchange and Open Neural Network Specification enable a machine learning model that was created in one front-end tool to be automatically compiled for efficient inferencing on heterogeneous AI accelerator chips.
What this points to is a world in which AI developers will place less importance on a proprietary cross-processor programming API provided by a single hardware provider such as NVIDIA or Intel. GPUs and other back-end hardware platforms will be hidden from developers as multi-technology AI accelerator ecosystems take root from cloud to edge.
About the Author
You May Also Like