How AI Bias Is Impacting Healthcare
AI bias seeps into algorithms and models that affect clinical and health insurance decisions as well as clinical trials. InformationWeek speaks to experts that discuss how to avoid these discriminatory errors.
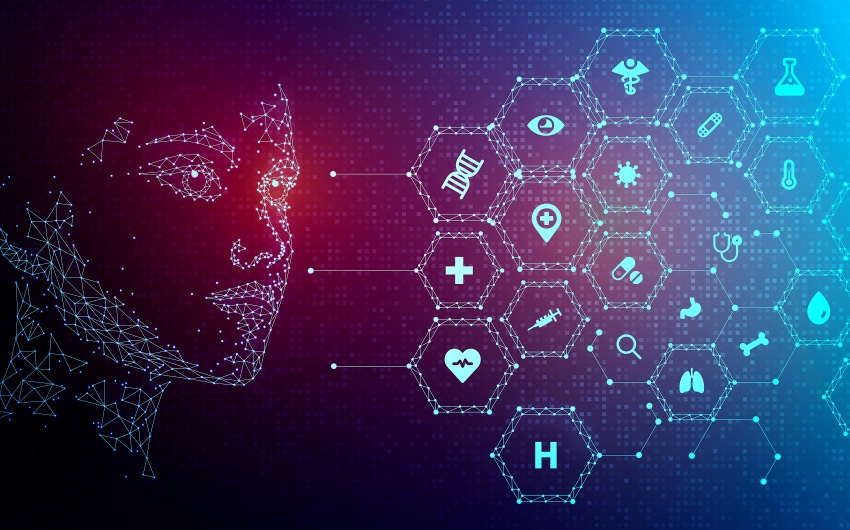
Artificial intelligence has been used to spot bias in healthcare, such as a lack of darker skin tones in dermatologic educational materials, but AI has been the cause of bias itself in some cases.
When AI bias occurs in healthcare, the causes are a mix of technical errors as well as real human decisions, according to Dr. Marshall Chin, professor of healthcare ethics in the Department of Medicine at the University of Chicago. Chin co-chaired a recent government panel on AI bias.
“This is something that we have control over,” Chin tells InformationWeek. “It's not just a technical thing that is inevitable.”
In 2023, a class action lawsuit accused UnitedHealth of illegally using an AI algorithm to turn away seriously ill elderly patients from care under Medicare Advantage. The lawsuit blamed naviHealth’s nH Predict AI model for inaccuracy. UnitedHealth told StatNews last year that the naviHealth care-support tool is not used to make determinations. “The lawsuit has no merit, and we will defend ourselves vigorously,” the company stated.
Other cases of potential AI bias involved algorithms studying cases of heart failure, cardiac surgery, and vaginal birth after cesarean delivery (VBAC), in which an AI algorithm led Black patients to get more cesarean procedures than were necessary, according to Chin. The algorithm erroneously predicted that minorities were less likely to have success with a vaginal birth after a C-section compared with non-Hispanic white women, according to the US Department of Health and Human Services Office of Minority Health.
“It inappropriately had more of the racial minority patients having severe cesarean sections as opposed to having the vaginal birth,” Chin explains. “It basically led to an erroneous clinical decision that wasn't supported by the actual evidence base.”
After years of research, the VBAC algorithm was changed to no longer consider race or ethnicity when predicting which patients could suffer complications from a VBAC procedure, HHS reported.
“When a dataset used to train an AI system lacks diversity, that can result in misdiagnoses, disparities in healthcare, and unequal insurance decisions on premiums or coverage," explains Tom Hittinger, healthcare applied AI leader at Deloitte Consulting.
“If a dataset used to train an AI system lacks diversity, the AI may develop biased algorithms that perform well for certain demographic groups while failing others,” Hittinger says in an email interview. “This can exacerbate existing health inequities, leading to poor health outcomes for underrepresented groups.”
AI Bias in Drug Development
Although AI tools can cause bias, they also bring more diversity to drug development. Companies such as BioPhy study patterns in patient populations to see how people respond to different types of drugs.
The challenge is to choose a patient population that is broad enough to offer a level of diversity but also bring drug efficacy. However, designing an AI algorithm to predict patient populations may result in only a subset of the population, explains Dave Latshaw II, PhD, cofounder of BioPhy.
“If you feed an algorithm that's designed to predict optimal patient populations with only a subset of the population, then it's going to give you an output that only recommends a subset of the population,” Latshaw tells InformationWeek. “You end up with bias in those predictions if you act on them when it comes to structuring your clinical trials and finding the right patients to participate.”
Therefore, health IT leaders must diversify their training sets when teaching an AI platform to avoid blindness in the results, he adds.
“The dream scenario for somebody who's developing a drug is that they're able to test their drug in nearly any person of any background from any location with any genetic makeup that has a particular disease, and it will work just the same in everyone,” Latshaw says. “That's the ideal state of the world.”
How to Avoid AI Bias in Healthcare
IT leaders should involve a diverse group of stakeholders when implementing algorithms. That involves tech leaders, clinicians, patients, and the public, Chin says.
When validating AI models, IT leaders should include ethicists and data scientists along with clinicians, patients, and associates, which are nonclinical employees, staff members, and contractual workers at a healthcare organization, Hittinger says.
When multiple teams roll out new models, that can increase the time required for experimentation and lead to a gradual rollout along with continuous monitoring, according to Hittinger.
“That process can take many months,” he says.
Many organizations are using proprietary algorithms, which lack an incentive to be transparent, according to Chin. He suggests that AI algorithms should have labels like a cereal box explaining how algorithms were developed, how patient demographic characteristics were distributed, and the analytical techniques used.
“That would give people some sense of what this algorithm is, so this is not a total black box,” Chin says.
In addition, organizations should audit and monitor AI systems for bias and performance disparities, Hittinger advises.
“Organizations must proactively search for biases within their algorithms and datasets, undertake the necessary corrections, and set up mechanisms to prevent new biases from arising unexpectedly,” Hittinger says. “Upon detecting bias, it must be analyzed and then rectified through well-defined procedures aimed at addressing the issue and restoring public confidence.”
Organizations such as Deloitte offer frameworks to provide guidance on how to maintain ethical use of AI.
“One core tenet is creating fair, unbiased models and this means that AI needs to be developed and trained to adhere to equitable, uniform procedures and render impartial decisions,” Hittinger says.
In addition, healthcare organizations can adopt automated monitoring tools to spot and fix model drift, according to Hittinger. He also suggests that healthcare organizations form partnerships with academic institutions and AI ethics firms.
Dr. Yair Lewis, chief medical officer at AI-powered primary-care platform Navina, recommends that organizations establish a fairness score metric for algorithms to ensure that patients are treated equally.
“The concept is to analyze the algorithm’s performance across different demographics to identify any disparities,” Lewis says in an email interview. “By quantifying bias in this manner, organizations can set benchmarks for fairness and monitor improvements over time.”
About the Author(s)
You May Also Like