Predictive Analytics 101: Getting Started and Avoiding Mishaps
Predictive analytics, offering a data-enabled view into what is likely to happen, are key to driving organizational change.
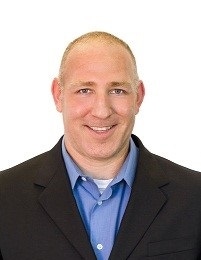
Predictive analytics is not exactly novel. It’s been around for decades. However, it’s only within the last few years that brands and organizations have started to adopt the discipline as an effective way to bolster their online marketing and spike the top and bottom lines.
Data mining, text analytics, optimization and machine learning, among other areas, are core to predictive analytics.
Adoption rates vary; however, in light of an increasingly competitive and global economy, the sooner companies get started cultivating predictive analytics the better.
The best way to begin a predictive analytics strategy is to start small and prove success before expanding it to other areas.
Getting started
The first step is to start a proof-of-concept project, which requires targeting a highly visible business area. To identify your initial target business areas, look at your strategic agenda or enterprise risk register. There will be many areas where you can develop models from historical data to predict future targets that can help you to either create value or identify potential loss. These areas will likely be:
Marketing campaigns: Predict which customer segment is most likely to purchase in response to a campaign or which messaging will cause the highest conversion.
Sales: Predict customer lifetime value, understand what a buyer’s next best offer may be, identify suggestive products.
Customer lifetime: Learn to identify markers that indicate which customers are likely to drop your product or churn altogether.
Fraud: Predict which recipients of benefits (i.e. entitlement programs, insurance claims, healthcare, etc.) or employees processing payments are likely to commit fraud.
Operations: Forecast inventory needs or predict which employees are vulnerable to quit so you can proactively manage churn.
Supply chain or vendors: Identify weak links in your supply chain or model vendors/third parties prone to fraud.
Next, figure out the data you’ll need — making sure it’s clean and plentiful — and begin to build a model that will predict your future target. Building your first model will require a little trial and error, but you’ll be able to iterate quickly and build trust with business leadership through some early return on investment.
Ensuring data quality
Data quality is often the biggest obstacle preventing the business from making progress in getting value from predictive analytics. Cleaning and harmonizing disparate data is also the most difficult step in any analytics initiative for the data scientist or analytics team, often requiring 80 percent of their initial effort.
However, overcoming this barrier is necessary to achieve any meaningful insight. Otherwise, it’s “garbage in, garbage out,” making it nearly impossible to deliver meaningful insight or ROI and making your predictive analytics program likely to fail fast.
In the long run, your strategy will need the foundation of a good data management program to help manage access, cleansing, harmonizing and analysis.
Ensuring data compliance
In addition to quality data, there will be legal and regulatory obligations, which include common global standards like PCI for payment processing or cybersecurity, or industry specific rules in areas like healthcare, financial services, conduct, and privacy. Common data management programs may include a data warehouse or a data lake for this purpose.
For instance, in the wake of European GDPR regulation, are you authorized to move data that includes personal information of EU citizens to your data lake, or do you have to filter out specific regions or specific types of data? When your program reaches a higher level of maturity, having your general counsel and compliance officer to consult as stakeholders will ensure you comply using the right data only.
Don’t treat your data like garbage
The biggest problem facing many organizations is that they are not yet doing enough with their data despite the big data craze and the low cost of computing and data storage. Andrew Sherman, a recognized expert on business growth, said at his TEDx talk at the University of Nevada, that the S&P 500 wealth has transitioned from primarily physical assets in the 1970s [87% physical 13% intangible] driven from a manufacturing economy, to primarily intangible assets in 2000s [84% intangible 16% physical]. Thus, Andrew posits that organizations generally treat their information as garbage and throw it away.
However, by using predictive analytics, information is treated as untapped value, rather than debris.
Change is accelerating in every part of the business landscape, and every industry exists in an information economy. Those who embrace data as the new oil, and analytics as the combustion engine, will not only persist but often out-compete. Embracing predictive analytics is the most effective way to facilitate the change and monetize your data.
Kris Hutton is Director of Product Management for ACL.
About the Author
You May Also Like