Shark! A Toothy Ton of Data
Researchers are using machine learning to get a clearer picture of shark behavior, and work to help those sharks to survive.
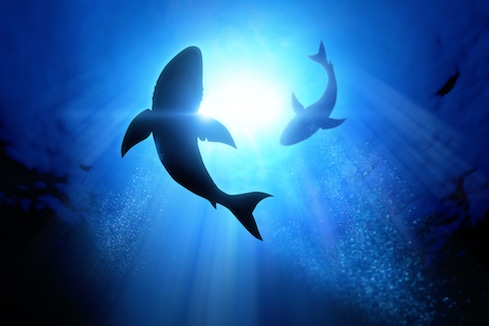
When scientists want to study birds, they have an enormous crowdsourced data set that they can use. When they want to study mammals on land, they can tag them and track them. But what about when scientists want to study marine animals like sharks?
That's a little more complicated. The world's oceans are much bigger than its land mass. Human scientists are land creatures, too, so most of their in-person observations are limited to the surface of the water, and the surface is just a fraction of the actual volume of the ocean which averages 2.3 miles deep. Plus, the salt water of the oceans is pretty much incompatible with electronic equipment for tracking animals' motion and behavior. All this together makes studying sea creatures a lot more difficult than studying other animals. Indeed, it leaves much of sea life a mystery to us.
Maybe that's why the most prevalent characterizations of sharks are those from fiction -- the man-eating monster in Jaws and all its sequels, for instance.
Yet scientists have been trying to set the record straight on shark behavior for decades by using actual data instead of the imaginations of Hollywood writers. One of those scientists is Salvador Jorgensen, a senior research scientist at the Monterey Bay Aquarium's Project White Shark. Using a number of innovative "tags" that have been packaged to be impervious to the ocean's salt water, this project collects data about shark movement and behavior and then uses analytics and machine learning to gain a more complete picture of what sharks do.
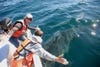
Salvador Jorgensen successfully tags a white shark
These tags are actually collections of sensors -- among them gyrometers, accelerometers, thermometers, and most recently a camera -- specially packaged for marine environments and designed to be attached to or ingested by a shark.
These devices also include a beacon, and after about a week in the field, which is about how long the battery lasts, these devices are collected and brought back to the lab for data transfer and analysis, Jorgensen told InformationWeek in an interview.
Jorgensen said these data logger devices are like Fitbits for sharks. Devices can track the shark movements over the course of several days. Some devices are equipped with cameras and attached to fins to get a shark's-eye view of the ocean. And some devices are packed in blubber and fed to sharks. Jorgensen pioneered this device ingestion technique, which allows the device to monitor how often the shark feeds by recognizing changes in temperature that only happens when ocean water enters the stomach along with the next meal.
"We feed these devices to the shark wrapped in a blubber burrito," Jorgensen said. Like owls, sharks eventually spit out the parts of their meal that are undigestible.
After about a week, Jorgensen and his team start to look for the beacons and collect the devices. Jorgensen said that the beacons are equipped with a wet/dry switch that is activated once the device floats up to the surface, breaking the circuit.
Some of the devices do get lost. Some wash up on beaches many miles away and a phone number and cash reward on the packaging prompts the people who find the device to contact the lab and send it back there. Jorgensen said that people are usually pretty excited to hear that the object they found was either attached to or ingested by a shark.
Once the device arrives back at the lab, research scientist Jerry Moxley and deep learning engineer Zac Liu work on downloading the data from it via a WiFi connection. The data comes down in a 15 to 25 column data set in a CSV format. Moxley told InformationWeek in an interview that the data is made up of multichannel sensor data that may include data such as depths, accelerometry, video fields, and more. All this is downloaded to a local server at the aquarium and catalogued with many more datasets. Currently the team has hundreds of gigabytes of data stored, but less than a terabyte. The data is organized by deployments, according to Moxley.
Once its downloaded, Moxley works on the data using R, and Zac uses Python to work with machine learning package Keras to operate on the Tensorflow deep learning library. For the compute, the team leans on CyVerse, a supercomputer initiative by the National Science Foundation.
The team is using these advanced technologies to gain more insights into the data they already have. For instance, to save battery life the sensors in the field don't capture a high resolution of data all the time. They may collect a high level of detail for 6 hours and then go back to collecting data at a lower resolution to save on battery life. That's one of the reasons why Jorgensen said that any innovations that increase battery life will probably be the technology to have the greatest impact on shark research. Currently, the team uses machine learning to fill in the blanks of high resolution data during a time when only low-resolution data was collected.
Specifically, for example, how often is the shark beating its tale? By using machine learning to extrapolate high resolution data to fill in the blanks of low resolution data, scientists get a better picture of shark energetics and activity and calorie budgets, Moxley said.
What have the scientists learned about shark behavior?
"One of the surprising things we've learned about white sharks is that they don't eat that frequently," said Jorgensen. "We knew from early studies they could go as long as a month. A strategy for white sharks is to have a large meal and then go long periods without foraging. They are not going after everything they see. They are bypassing potential prey all the time."
About the Author
You May Also Like