The Secret to AI Could be Little-Known Transfer Learning
As more enterprises discover AI-based business applications, the concept of transfer learning could help to level the playing field.
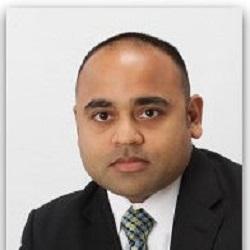
Consumers have spoken, artificial intelligence is a profitable industry. From Amazon to Google to Apple, major tech companies have made inroads, crafting intelligent software — housed in sleek, accessible hardware — that has drawn massive customer attention.
This trend is set to soon move out of home devices, like Echo and Google Home, and onto the streets, where self-driving cars leverage major breakthroughs in computer vision so passengers can ride easy, knowing their vehicles will “see” and react to objects and road signs in real time without their input. In fact, cars with these features are already popular with consumers, and by 2020 10 million cars with self-driving attributes will be on roadways.
But while there are plenty of ways for consumers to leverage AI, enterprises are asking themselves how they can get in on this wave of innovation. And a big part of the answer lies at the crossroads of computer vision and an emerging field known as transfer learning.
Transfer learning has the potential to unlock dozens of new AI use cases in the enterprise by reusing existing, state-of-the-art deep learning models.
In short, transfer learning is an approach to taking existing AI models and applying them to new data. In this case, we’ll talk about computer vision models that make deductions based on images and visual data, applying that learning on numerical sets of data. It means that businesses could use the very advanced deep learning models that perform computer vision functions, like for self-driving cars, and apply that level of sophistication to a whole new set of, non-image datasets on a spreadsheet.
The way transfer learning works is that the algorithm functions much like the human brain does when looking at a small dataset in Excel. We use our eyes to scan through information and mentally detect patterns. Computer vision applied to numerical data does the same thing, through convolutional neural networks, which look for both high- and low-detail features of an image to help classify what is pictured.
Turning numbers into images
Through transfer learning, a model built originally for computer vision use cases can take a layered approach to curate the new numerical data it's fed, improving its guesses on patterns in data from a separate domain. Researchers have proven this out, showing that transfer learning that is built using vision-based source data can be applied to a target domain of data that doesn’t contain visual information, like sensor data from Internet of things devices, for example.
For instance, a 2-D sensor’s numerical readings can be converted into pressure distribution image heat maps. Then the convolutional neural network’s last layer is peeled back, and transfer learning aids the computer vision model to reinterpret the data as a visual.
In terms of use cases, often, for many deep learning applications to work, computer vision included, it needs a much larger dataset to gain new insights from. That is something many companies have as the world ramps up to having more than 4.4 zettabytes of data. However, using transfer learning, enterprises can extrapolate patterns and real business insights from smaller data sets as well.
Companies can apply existing and sophisticated computer vision models to more traditional use cases like fraud detection and prevention, preventative maintenance, and marketing attribution. These are all areas where enterprises can apply image-based deep learning models to more general, or numerical, data sets. Much of this results in net-new insights that are not achievable with traditional machine learning methods or advanced analytical approaches.
It is important for business leaders to know that deep learning, and specifically computer vision, has much wider applications in the enterprise beyond simply vision-based use cases like autonomous driving and identifying facial micro-expressions. In fact, applying computer vision to enterprise problems can unlock dozens of new use cases and business outcomes. It’s by no means easy, but deep learning does provide a path ahead. Not every company can afford to spin up 1,000 new hires in data science, like Google or Amazon — and they don’t have to.
Not only are the state-of-the-art models mentioned available, but they can also be embedded as pre-built functions through popular libraries like TensorFlow and Keras. Therefore, methods like computer vision combined with transfer learning can help level the playing field for any businesses today that want to use AI to get more out of their data.
Mo Patel is Practice Director for AI and Deep Learning at Teradata.
About the Author
You May Also Like