To Operationalize AI, Invest in Humans
Enterprise organizations are showing strong interest in artificial intelligence, but many are struggling to move beyond experimentation.
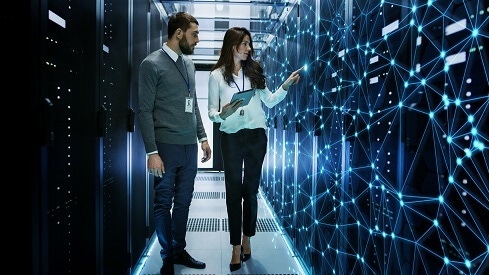
IT leaders and business executives around the world recognize the strategic importance of operationalizing AI, yet surprisingly few have moved beyond experimentation. A recent Capgemini survey finds that only 13% of companies have moved beyond proofs of concept (POC) to scaling AI across the enterprise.
The struggle to operationalize AI is painful because it represents lost time and resources and unrealized potential. Articles abound full of suggestions, frameworks and manifestos, shared with the intent of closing the gap between AI concept and enterprise delivery (including one proposal to eliminate the POC altogether). Many of these are smart and worthwhile. Something that we’ve rarely seen mentioned in any of them, however, is the counterintuitive advice to invest in humans.
We’ve seen multiple organizations create data science teams with the express intent of operationalizing AI, and we’ve delivered several successes. What we’ve learned is that operationalizing AI often requires an increase in human involvement. Here’s some advice:
1. Expand your team’s skill sets. Data scientists spend a small portion of their time building AI models. They spend most of their time understanding the business problem, collecting and cleansing data, interpreting data from a business context, exploring hypotheses suggested by stakeholders and more. Though it’s complicated, building a model -- a forecast or recommendation engine -- is one of the simplest steps.
Often the hard part is establishing the necessary system of support to operationalize AI. Organizations can’t just hire a team of data scientists and expect them to be successful. Besides data scientists, organizations need software developers -- increasingly specialists in data and machine-learning engineering -- and program managers. Specialize for effectiveness, generalize for efficiency. Develop the right community of practice and the appropriate trade-offs between efficiency and effectiveness.
2. Evolve team competencies. Keeping humans in the loop is necessary for success. The most well-designed models are far from foolproof. Models break. Undetected errors can quickly compound. You’ll need knowledgeable people to monitor your AI systems and respond to issues as they arise. Moreover, you’ll need your team to continuously evolve their competencies.
Consider doctors, who complete four years of college, four years of medical school and multiple years of residency. Nevertheless, their education never ceases. As new discoveries are made and the state-of-the-art advances, doctors evolve. The same must be true for your teams. Your teams need to have the necessary skills to leverage advances in technology and algorithms.
3. Translate for the business. The business insights function is critical to any operationalized solution. No matter how automated a process is, it’s still solving a business problem. Reporting back to the business about what worked and why, is critical to AI’s success. For example, if an AI model is a recommendation engine, how well is it serving its purpose? What worked and what didn’t? Who acted on the recommendations? Based on what we’ve learned so far, should we redesign the product or the offer? These and many other business-oriented questions should be asked, answered and communicated.
4. Work collaboratively to pick the right problems. Not all problems deserve to be operationalized. Sometimes only one department needs a solution that’s used by few people. Such solutions can be sophisticated, but don’t require widescale deployment across your company. AI models that are run annually to support brand strategy or go-to-market strategy, for example, may not need to be operationalized. Models that feed sales reps’ actions, such as recommendation engines that determine next-best actions, should be operationalized.
5. Integrate your AI teams into the business. Organizations need comprehensive visions. They need to build complete teams, but they also need a plan for integrating AI and their data science teams into the broader organization. There are a few ways to accomplish this.
A centralized model combines an organization’s data scientists (and potentially other related specialists) into one shared resource. A centralized team has a greater center of gravity, which makes it easier to drive company-wide initiatives. They also often take a broader perspective on the business, so these projects align closely with the company’s priorities. A federated model embeds data scientists into other organizations within the company, allowing them to work more closely with subject-matter experts and the business. Consequently, they likewise make more localized prioritization decisions. The projects delivered by federated data science teams are often smaller in scope, but more agile in nature. A hybrid model combines the best of the centralized and federated models by having data scientists report to a centralized organization, complemented by “dotted-line” accountabilities with stakeholder organizations.
Operationalized AI is worth striving for, but it’s a strenuous journey. You need an infrastructure in place to support the rapid deployment of new AI pilots. That infrastructure is people. Invest in their development, promote their communication and collaboration, and embed them into the business. It takes some effort to get there but persisting will be worth it as the rewards are numerous.
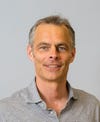
Ron Sielinski is principal director of data sciences at Microsoft.
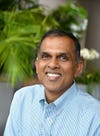
Arun Shastri PhD, leads the analytics practice at consulting firm ZS.
About the Author(s)
You May Also Like