Top 5 Considerations When Hiring a Data Scientist
AI is a hot term in every industry, but don’t be a company that just blindly hires a group of data scientists who end up frustrated and unproductive.
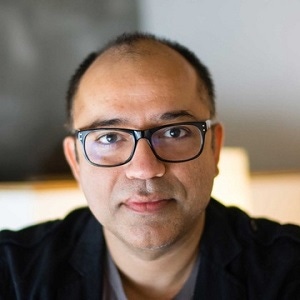
Only 10 years ago, the number of people who truly understood artificial intelligence across the entire world could fit into a large floor of a building. Fast forward to today and we believe AI is precipitating the next industrial revolution. Is that possible? Could that small group really have caused this? Do we need more people driving this revolution and working to uncover novel applications? The obvious answer is yes, but it's not so straightforward. We cannot buy or hire our way into AI by hiring a few data scientists, although that seems to be the trend these days.
Data science programs at various universities are thriving, and now even business schools like Stanford Graduate School of Business are teaching data science courses. The rate at which these programs is growing probably is still falling short of the need or the potential.
In fact, if you look at the few companies that are lucky enough to have the big bucks to get these “mythical beasts” -- or AI experts -- they are struggling to figure out what to do next. How do you really get value out of AI? How do you create the magic? Furthermore, there is growing discontent among data scientists about their inability to impact their organizations and deliver on the promise of AI.
Here are my top five considerations when building a data science organization. These considerations are 80% of the work in making a data science team or an AI strategy fruitful to your organization; while the quality of the people and the team you hire coupled with their skills and experience makes up the remaining 20%.
1. Ensure data scientists are organized to make an impact. There seems to a common misconception in how companies are building data science teams. Many enterprises and startups have created this “special” group where data scientists live, often referred to as simply the “data science team” or even the “AI Center of Excellence”. While it sounds like a fascinating place to create and join initially -- an ivory tower of sorts -- the tower often can be disconnected from the rest of the business. More often than not data scientists exist outside of the product development, technology, or IT organizations from where they have little impact on the business or product line of the organization. There is no way to take advantage of data and machine learning from the outside.
Second, living outside the data scientist cannot really understand your data or business, both essential to creating appropriate actions using data and ML. The way I’ve seen huge success come out AI is by incorporating data and data science into the core of the business and the heart of product development.
2. Treat data scientists like any other resource on your team. All of the criteria for hiring an effective engineer or product manager should be applied to the data scientist as well. They’re just another kind of engineer, not a foreign entity to be treated differently. For some reason, data scientists are being tested more for their data science skills and there is a notion that another engineer will come in and productize their ideas. That rarely works because data science products, like most products, require constant iterations and pivots. There is no way to do that through a handoff to an engineering or product team. It’s also essential to test your data scientist candidates for communication, problem-solving, engineering, and coding since those are 80% of the skills required in a great data scientist. The data science part is only 20% of the skillset. Perhaps in some far-flung future, we could have a world where data wrangling and production engineering are all done through a platform, but that's not possible today.
3. Be ready to integrate your strategy, product, engineering, and IT organizations with AI and data. In addition to the ivory tower data science team approach, a second approach is a lone wolf data scientist. Some people imagine that data scientists get PhDs with secret recipes that act as magic potions. The idea is that somehow the lone wolf can produce magic, which can be consumed by non-data scientists and plugged into current product and strategy.
However, transforming a business using AI requires a complete retooling of your staff, not just in engineering and IT but strategy and product. The most important first step to data science is finding out what problems can or should be solved and what data you have to solve it. This isn’t something your data science team can figure out independent of your business organization. Do you have people in these areas who can understand and take advantage of big data and machine learning? If so, the way to build AI is to take everyone in your engineering organization and have them build AI. The lone wolf can at best act only as a consultant. The good news is that most engineers are eager to learn.
4. Figure out if you have unique data or a unique problem or whether to just buy an AI product. Just like traditional applications such as CRM, not every company needs to hire data scientists or build out generic AI applications. This is especially true if the problem is horizontal and there is nothing unique to your business that prevents a vendor from solving it. For example, I run into companies daily who have one to three in-house data scientists trying to solve the same problem that our technology solves across industries. Vendors can have more resources, more data, and more time to solve these problems. So in some cases, there is no reason for a company to try to solve a problem by themselves.
5. Your data scientist must be nimbler than your typical engineer. Data science projects fail at a higher rate than conventional software like a run-of-the-mill SaaS application. Today, some product and engineering organizations are built to deliver very effectively on what is now a conventional software development model. In contrast, AI applications are closer to research in pharma, where things may fail even with the best team and the right resources. They can fail because we lack data, or the performance of the model is just not good enough. To mitigate this, an intense experimental approach needs to be taken with AI development. The data scientists and machine learning engineers need to be able to iterate faster and fail faster than regular software engineers. Initial results should be expected (positive or negative) in days, not weeks. This encourages a culture of experimentation that will fail hundreds of times before finding the true solution.
Given that it's important for your data science team to run hundreds of parallel experiments, the skills of the data scientist should include enough data engineering and product development skills to iterate themselves end-to-end rather than depending on others. There is certainly a gap in the market where most data scientists lack basic software engineering and data engineering skills.
Ultimately, look to hire someone who is passionate about solving your organization’s challenges, is a good communicator and problem solver, and has engineering as well as data science skills. AI is a hot term in every industry, but don’t be a company that just blindly hires a group of data scientists who end up frustrated and unproductive. The value AI brings is very real, but companies have a responsibility to be strategically aligned to take advantage of it. Any real transformation like digital, cloud, or AI cannot happen in isolation by a small disconnected group.
Aman Naimat is senior vice president of technology at Demandbase.
About the Author
You May Also Like