What You Need to Know About Augmented Analytics
As enterprises travel on a digital transformation path, they need to stay current with analytics, but it’s not a linear journey.
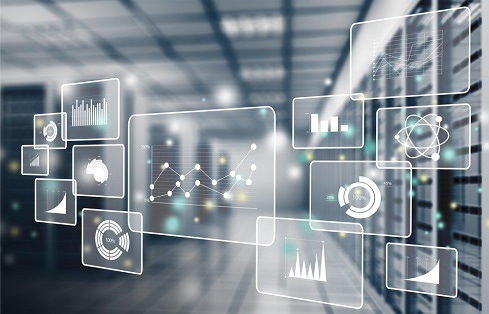
Static reporting and dashboards aren’t enough to stay competitive anymore. The problem is insight limitations. Specifically, you need to know (or more precisely, you apply what you consider to be) the variables and correlations that matter. Enter augmented analytics. This new class of tools uses machine learning and artificial intelligence to find patterns you may not have considered while speeding time to insights.
According to a recent Gartner report, “[Augmented analytics] leverages ML/AI techniques to transform how analytics content is developed, consumed and shared. [It automates] data preparation, insight discovery and sharing. It also automates data science and ML model development, management and deployment.”
Be careful, though. Just because augmented analytics automates some tasks and democratizes analytics among a broader array of users than dashboards doesn’t mean that organizations no longer need analysts and data scientists. In fact, Gartner expects by 2020, augmented analytics will become the dominant driver of new business intelligence and analytics purchases, data science and machine learning platforms and embedded analytics. (Note the three different audiences: business users, data scientists, and developers.)
Augmented analytics is the next disrupter
BI has become synonymous with reports, and analytics has become synonymous with dashboards, even though the lines have blurred over the years as traditional BI vendors updated their offerings. Augmented analytics is not synonymous with machine learning and AI, although it leverages those techniques.
“We’ve been through at least two waves in analytics. On the BI side you have the semantic layer, rigidly-modeled data warehouse era where the tools were only usable by people in IT, so that really limited what the broader set of business people could do with their data,” said Rita Sallam, VP analyst and Gartner fellow. “As a result of that, you saw a lot of exports to Excel and then companies like Qlik, Tableau and Tibco Spotfire entered the market with tools that were more departmental in nature. They targeted the hotbed of dissatisfaction among users that wanted to do more.”
Now the target market is an even broader base of users because corporate competitiveness depends on the quantification of everything. As companies continue to digitize more of their operations, they’re producing more data and more types of data that need to be analyzed. “Finding the needles in the haystack” is a more complex proposition than it once was to the point of humans needing machine intelligence to make better sense of it.
“Ten years ago, if I used a sales dashboard and saw my numbers going down, I’d ask if it was price or product quality,” said Sallam. “Now we have many more options, so it may be a combination of weather and something broke on my website. How can I explore all the possible combinations or permutations rather than 10 or 12 columns of variables?”
Enter machine learning and AI which can help prepare the data for analysis, analyze the data, and explain the insights. Augmented analytics vendors are attempting to address the shortage of data science talent by making data scientists and less-skilled people more productive.
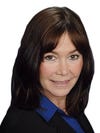
Rita Sallam - Gartner
“Data science is evolving in the same way where machine learning and some AI techniques are being used to automate the feature selection process, the model selection process, even the code generation once a model is selected,” said Sallam. “These new augmented analytics capabilities that are applied to data science and machine learning platforms automate a lot of that. They include an explanation of how the model was calculated and the statistics in terms of the accuracy of the model.”
Get ready for a new user experience
Reports and dashboards are alive and well in the enterprise and they will remain so for some time. However, both use pre-defined KPIs. That approach has value, but pre-defined KPIs also limit the scope of potential insights. For one thing, it may not be clear whether the KPIs are the right KPIs. Sallam said reports and dashboards also assume the user understands the relationships in the data.
Augmented analytics exposes insights dynamically based on context and what’s statistically important. The context includes but is not limited to the user’s role, what the user looked at in the past and what the user’s network looked at in the past, like the predictive engines used by Amazon, Facebook, Google and Netflix. Augmented analytics also uses the natural language conversations it has with users to further inform context.
Augmented analytics doesn’t automate data storytelling, though. It simply assists with and accelerates it.
Execute a parallel analytics strategy
Rip and replace is a bad idea here because BI and analytics products still provide a lot of value. More importantly, augmented analytics requires users to be data literate. Instead of planning a wholesale shift, leverage what’s in place while experimenting with augmented analytics so you can better understand the differences and how your analytics strategy should unfold over time.
“Companies will need to start looking very carefully at the vendors’ roadmaps [whose products] they already use to see where they are with respect to incorporating or adding or innovating with augmented analytics,” said Sallam. “Particularly early adopters will take a look at new, innovative vendors and bring them in alongside of their existing investments to see how they can enhance their analytic activities.”
Be sure to include analysts and data scientists in the validation process because they’re in a better position to know what questions should be raised and what tests should be used to validate the product.
Somewhere between slick sales collateral that promises anyone can do anything and the constraints of needing expert assistance for everything is reality. That reality is that tiers of expertise are necessary to democratize analytics and solve difficult problems simultaneously.
“We believe that as you push insights out to more people in the enterprise, data literacy becomes really important,” said Sallam. “[That way, users] know how to make decisions based on the information, what questions they should ask, the differences between the types of insights that are being served up, the implications that has on how they should make decisions and what they should factor in.”
Bottom line
The scale and complexity of data used in enterprises has become more than humans can handle well, so it’s no surprise that machine learning and AI are defining the latest wave in analytics, which Gartner calls “augmented analytics.” Augmented analytics increases the amount of machine intelligence that’s used in the human and machine partnership so humans can be more productive. Like other technology products, augmented analytics solutions aren’t a replacement for critical thinking. In fact, you should be thinking about data and analytics more critically than you ever have before.
About the Author
You May Also Like