Your Roadmap to AI and ML Deployments
Don’t adopt artificial intelligence and machine learning without having a real business problem to solve. Here are some ways to help build the right use cases.
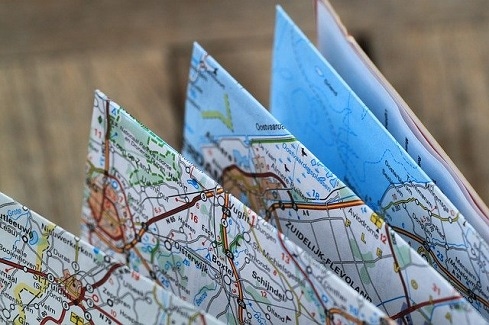
As the hype around artificial intelligence hits an all-time high, it is more important now than ever before for enterprises to find practical use cases for AI and machine learning deployments. But unfolding the ever-expanding map of possibilities posed by these technological advances can get tiresome, and many organizations lack the expertise to truly understand when AI and ML can add value -- and when they can’t. Despite how excited your team may be to leverage the power these tools offer, in both product development and for business decision-making, a lack of comprehension will serve as the greatest roadblock to benefiting from that power.
This is your roadmap for building the right use case for AI/ML within your enterprise.
1. Check for variability and uncertainty
The first seemingly easy step is identifying what you hope to accomplish by integrating and deploying AI or ML. The litmus test for whether AI/ML solutions are the right tools for the job is the degree of variability and uncertainty in the question or problem you are trying to solve.
Variability allows your models and algorithms to leverage different information from different attributes to make better, more specific predictions. The presence of uncertainty lends meaning and value to the predictions ML makes.
For example, predicting next year’s sales numbers is a very different problem than migrating data to the cloud. There’s definite uncertainty in predicting sales numbers because no one really knows the future -- just look at how quickly the COVID-19 outbreak upended businesses of all stripes. What is more, sales tend to be affected by many factors, such as the availability of your products, price, and a plethora of other external circumstances that are beyond the company’s control. That is variability.
The true value of AI and ML is their ability to apply logic and order to the chaos of variability and uncertainty. Unless your use case has both, you are likely dealing with a business intelligence or data engineering problem, making AI and ML overkill.
2. Gather your data
Once you have your use case locked and loaded, it is time to check on the data that you will be feeding into your model. To produce good results, your models might require a long data history and a variety of related attributes. When data for an important attribute is missing, it may be possible to use other variables as a proxy. Proxy variables essentially capture the same information as the missing variable. Think about what the attribute represents and how the essence of the numerical relationship might be captured or what could be correlated. For example, if you are building an economic model and don’t have reliable information about the employment rate, you may be able to use stock market or other sales variables as proxies.
If you are coming short on data, no need to panic. Depending on your use case, lack of data does not need to be a deal-breaker. Many AI/ML implementations allow for continuous learning over time as new data is collected, which can help make better predictions going forward. Spam filters, recommendation systems, and fraud models are just a few everyday examples that allow for continuous learning. There are also entire classes of models devoted to estimating what cannot be directly measured.
Some use cases may benefit from engaging an expert who can identify, select or modify available open-source models that can be used from the get-go, for example, AWS Sagemaker has a built-in image classification algorithm and Google’s BERT can be used to solve a wide variety of natural language processing problems.
3. Think about your business problems
But say AI/ML is a little out of your comfort zone, or you are an expert in another line of business, making it difficult to identify how AI or ML could help your business.
Even without all the pieces in place, you can start thinking about your business problems or customer pain-points. How do you solve them currently? How would you solve them if you had a better solution? Often this line of thinking reveals areas of uncertainty or variability -- ingredients you need for a potentially viable AI/ML application.
Next, determine whether there are any accessible internal data sources within your company. Often, identifying internal data and an area of uncertainty is enough to explore AI/ML as a viable business tool. All you need to start is to think about what a solution or design feature would look like.
Just remember at the end of the day, no one should strive to adopt AI/ML for its own sake -- it needs to solve a real business problem.
Sara Beck is a Machine Learning Solution Principal at Slalom Build with over a decade of data science and machine learning experience and additional leadership experience leading data science teams. Currently an Advanced Machine Learning Instructor at University of Washington, Beck earned a graduate degree in statistics with emphasis in bioinformatics/biostatistics, with machine learning teaching experience. Due to the length and cross-industry nature of her experience, Beck is keen at adapting to new machine learning and data tools. She loves working in professional services due to the variety of problem areas she has the opportunity to consider. Prior data science and machine learning experience included positions with T-Mobile and Starbucks.
About the Author(s)
You May Also Like