5 Machine Learning Resolutions for 2019
More organizations are using machine learning for competitive reasons, but their results are mixed. It turns out there are better -- and worse -- ways of approaching it. If you want to improve the outcome of your efforts in 2019, consider these points.
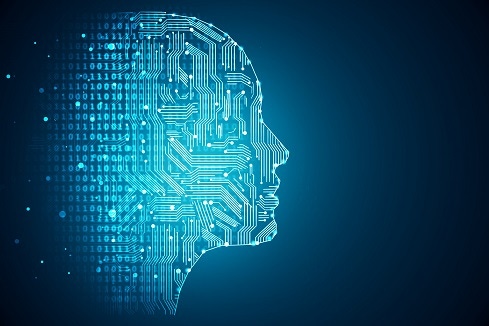
Businesses are using machine learning to improve all sorts of outcomes, from optimizing operational workflows and increasing customer satisfaction to discovering to a new competitive differentiator. Despite the explosion of options in the last few years, the results vary from organization to organization based on several factors. If you want to avoid some of the common pitfalls and drive more value from your efforts, resolve to do the following in 2019.
Start with an appropriate scope
Many organizations are so focused on the potential benefits of machine learning that they forget to consider the potential risks. In glossing over the details, it’s tempting to transform the entire business when it’s wiser to start with a use case or two, learn from the experience and build on the successes.
“Hype leads people to believe machine learning can do anything. If you believe the media, we have artificial general intelligence, but the reality is we have artificial narrow intelligence, machine learning that does narrow tasks,” said Steven Mills, associate director of Artificial Intelligence and Machine Learning of Boston Consulting Group’s Federal Division. “If you want to go down this path, you should have a vision and objective for doing it, because it will lead you through a process of reimaging the business problems you face, how you can solve them in a different way, how your business model could potentially be disrupted, and how value could flow differently through your industry.”
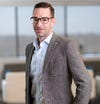
Steve Mills
During that process, Mills encourages clients to start identifying the first set of use cases.
Still, all the hype about machine learning and fears of being disadvantaged cause some organizations to attempt running before walking.
“Organizations say they have so much data and they think there’s a 'there' there without understanding how machine learning, deep learning, or automation is going to support the mission or organizational workflow,” said Josh Elliot, principal and director of artificial intelligence at Booz Allen Hamilton. “They’re starting too big, they have the wrong data or they have a lot of data but it’s unusable. We’ve also seen organizations that didn’t have infrastructure in place to accommodate what they wanted to do. Lastly, like most technology adoption, machine learning is constrained by culture.”
Two other things to consider are risk tolerance and whether the organization has the talent it needs to drive value from machine learning.
“You need to ask whether you have the talent internally not just to design and develop the algorithms but actually make it real for the end users and the customers that are going to be engaging or interacting with the machine in the future,” said Elliot. “We approach this technology in a very human-centered way, bringing user experience and UI-type experiential people into the conversations to make sure we have a full understanding of how the end user is going to engage with the machine or the output of the algorithm or model.”
Approach machine learning holistically
Machine learning is often approached as a technical problem when it’s really a multi-disciplinary effort. While the details of machine learning can get quite technical, the goals tend to focus on business impact. In addition, machine learning may impact business processes, the company’s culture, and the organization’s operating model.
“To get to value, you need to tease out how different it will be and some of the organizational challenges you might face so you can plan for them,” said BCG’s Mills.
A good first use case is one that can have a significant positive impact in a short period of time since a relatively quick win can help attract greater organizational support and continued investment. Don’t ignore longer-term projects, though, because some of them could be strategic to the business.
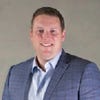
Josh Elliot
“You should map the more strategic use cases to your longer-term goals, which will get you to think about how to tackle those over time,” said Mills. “The most transformational use cases can take a lot of time.”
Generally, those participating in the discussion include whomever is responsible for machine learning, members of senior leadership, and the leaders of the business areas that would be impacted. When choosing which areas to focus on, organizations tend to consider problems that have been too difficult to solve otherwise, datasets that are valuable but underutilized or not utilized, or an area of the business that’s a major source of revenue for the organization. Such discussions require people who have deep knowledge of the business problems the organization faces, the data and what the affected areas of the business are trying to achieve.
BCG considers technology only 10% of the machine learning equation. The rest is data work (20%) and change management (70%).
Make the connection between data and machine learning
People have been hearing about the importance of data for the last couple of decades, particularly as it relates to business intelligence and analytics. However, the data may also serve as training data for machine learning purposes, which means things like data quality and bias need to be taken very seriously.
“You have to be thinking about how to get your data house in order as part of all this. That’s not to say you shouldn’t start thinking about machine learning initiatives before your data is perfect, because frankly that could be a three to four-year journey and it can be hard to get an organization to invest over that time horizon if you’re not showing value along the way,” said Mills. “You can’t do machine learning without solving the data problems.”
Data scientists spend considerable time getting data into a usable state. At the same time, some platform providers are claiming that most of that time is both unnecessary and wasted.
“There’s a huge amount of work that needs to be done because the data may not be machine-readable,” said BCG’s Mills. “Then, there are the technical nuances of thinking about classification. You have to think about class imbalance in the data and the implications of that. For example, if I’m going to sample customers, is that sample representative of my entire customer base? Pointing an algorithm at raw data can result in biased models, models that are not as accurate as you thought they were. There’s a lot more data work required than people tend to think about.”
Don’t expect too much “out of the box”
Despite the fact there are no silver bullets, enterprises keep looking for them. The shortage of data science talent has resulted in a flurry of product and service announcements designed to make machine learning easier for a broader base of prospects including data scientists, developers and even business users. However, transformative results are not necessarily just a few clicks away.
“Be wary of vendors, technologists, and data scientists who say they have a solution they created for one use case or client that can easily transfer over into your environment. Anyone in their right mind that’s working in this space recognizes that every environment is different, each organization has different data and values that data differently, and everyone weights things and the outcome differently,” said Booz Allen Hamilton’s Elliot. “There are models and those algorithms may provide a 60 – 70% solution, but they have to be retrained. Understand that is critically important.”
It's also wise to ask whether the vendor can explain what its algorithms are doing. Granted, not all machine learning scenarios involve the same level or type of risk, but the need for transparency is another important detail to consider.
Don’t forget infrastructural requirements
Machine learning involves several considerations, including speed, accuracy and ROI, all of which can be negatively impacted by infrastructural shortcomings such as the inability to store and process the requisite amount of data.
“Some of the deep learning neural networks require tremendous amounts of computing power to train the systems,” said Elliot. “Clients don’t necessarily understand the capital upfront costs whether it’s in the cloud or on-prem.”
BCG’s Mills suggests contemplating the desired end state and the machine learning architecture that will be required to achieve it and then as individual use cases are addressed, build toward those long-term goals to avoid creating problems that need to be fixed later.
“If I’ve got people building new models that are all using the same datasets, the same ETL functions, the same basic frameworks, there’s an efficiency play. It’s a bit of a risk reduction play too because you’re creating a standardized set of system interfaces and systems being exercised and used, and it just gives you that many more opportunities to catch issues for anything that’s concerning you,” said Mills. “If you’re building a whole new system every single time, there are so many more places where you can have problems. You’re far better trying to work off the same baseline and have everyone look at, examine and test that one baseline.”
Bottom line
Don’t “just do” machine learning, consider what you want to do with it first and why, and then start experimenting. While all the possibilities may have you thinking on a grand scale, it’s better to start small and grow, working toward longer-term goals while accomplishing shorter-term ones.
Taking a holistic approach to machine learning is a cross-functional effort that requires analyses and cooperation at both business and technical levels. Without that, machine learning efforts might be interesting, but less impactful and successful than intended.
[To learn more about trends in machine learning, check out these recent articles.]
How Employers and Employees Can Adapt to an Automated Workforce
Build Reliability into Autonomous and Intelligent Systems
A Digital Twin Approach to Predictive Maintenance
How Automation Empowers the CIO to Think Outside the IT Department
About the Author(s)
You May Also Like