How to Choose the Right Analytics Skills
Just because an analyst hits the ground running does not mean that analyst has the ongoing breadth needed to solve every day analytics problems.
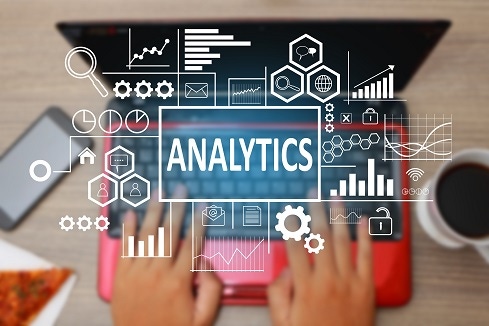
It is wonderful to imagine an analyst hitting the ground running to bring his or her skills to bear on a key problem. The desire for such a person certainly exists, as demand for data analysts and managers have risen over the years. Last year, LinkedIn reported that job shortages for data science skills intensified between July 2015 and July 2018. According to LinkedIn, any manager in Dallas or Atlanta would have had hiring options back in 2015, but in 2018 both cities ranked among the top 10 that had shortages for data science skills.
Yet the reality is that such a data miracle worker will likely work few miracles, if at all. No one person will embody all the analytic and data science skills that a manager requests. Managers must be ready to seek candidates who have the right temperament to engage a range of skills, rather than narrow specific skills. Every day data science and problems demand breadth of skills. The right person needs to show a breadth of interest, because learning and applying the desired skills require working with other professionals as much as it does a mastery of certain topics.
At this year’s Interop conference I heard a presenter say that a professional can be a "jack of all trades, master of one.” This implies that selecting the right analytics skills mean envisioning each role as a hybrid of general responsibilities with one really in-depth skill that the candidate feels confident about. The responsibilities cover the following concepts:
Data governance
Data management
Programmatic skills for creating models
An awareness of organizational agility requirements
Picture the responsibilities along the cross branch of an upside down T, with the tall part being the in-depth responsibility you want from a specific role. Once you do, you will have a good idea of how to frame the broad skills a candidate should have.
Notice that I did not suggest knowledge of a specific tool or programming language. Onboarding a familiarity with either is indeed essential. But a flood of tools exists in the market with considerable training options. In addition, most object-oriented languages have similar variation of functional features. You can have techniques for promises and closures in R programming the same way you can in Python. But the real saving grace in selecting a candidate is to look beyond a specific skillset to examine how and when that skill is applied. That examination likely will reveal a skill fluidity -- how they work with others to solve problems as well as contribute what they know.
More on Data Science Skills
Python Beats R and SAS in Analytics Tool Survey
Five Factors Shaping Data Science
How to Prep Your Career for the AI Job Apocalypse
Much of the need for skill fluidity stems from better aligning teams to advanced software delivery process such as DevOps, agile development, and CRISP-DM. The steps in these processes can be cyclical to get to a final product. CRISP-DM is applied to data and data wrangling to create the optimal model can feel ongoing. The cyclical nature changes the value viewing a base skill as the key to success. Instead, a candidate’s ability to unlearn what he or she has learned against their familiarity with associated operations is the key.
Scaling analytics means aligning professional commitment with strategy.
A solid learning environment will raise capabilities, dovetailing into the need for diverse talent. The interest in increasing STEM (science, technology, engineering and math) representation among women and minority professionals is high. Increasing team diversity would do more than provide a vital support to that interest. Developing a diverse team capability would bring forth more perspectives on data analysis that avoid social gaffes encountered by machine learning initiatives. It is imperative to support focusing on potential insights rather than focusing on technical solutions.
A solid learning environment also establishes a positive sentiment that can bolster team morale when complex challenges such as developing a machine learning model arise. Teams will better internalize new knowledge and skill, while organizations enjoy a system that helps to retain that knowledge when attrition occurs.
Management recognizes the need to maintain analytic capability to stay competitive. They are now learning that any advantages gained exist on a continuum. So when hiring a smart candidate, the best approach is to focus on the candidate’s general ability to reason and to interact with other people against which knowledge set they possess. Building on the candidates’ experiences will lead to an analytics approach that the organization can eventually internalize and lead to building the best team possible.
About the Author(s)
You May Also Like