Setting up Your OT and Data Teams for Success
Organizations implementing the Industrial Internet of Things can't burden data scientists with cleaning up huge volumes of dirty data.
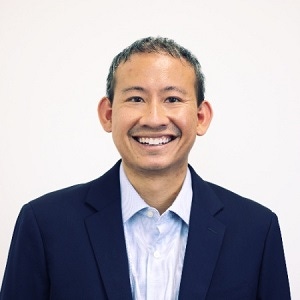
There is no one more important to the Industrial Internet of Things (IIoT) than data scientists. Data scientists are charged with taking vast amounts of raw industrial data, creating structure within that data, and ultimately finding valuable meaning. In other words, let’s dump a bunch of dirty data on these data scientists, tell them whatever we know about it, and trust they will magically build the model that provides meaningful insights and improves our processes. Powerful idea, but highly debatable on actual effectiveness.
The ideal IIoT deployment, whether it’s in the cloud or at the edge, is a seamless marriage between the know-how of the factory floor domain expert who makes sense of the physical industrial world and the power of a data scientist to derive complex analytics and machine learning algorithms. This combination of skills creates the industrial models that accurately prevent a bad outcome before it happens, such as a gigantic compressor failing in the middle of nowhere or an upstream process affecting the yield downstream. Notice that before it happens is emphasized.
There are significant hurdles to this grand vision of data science that prevent expensive mishaps and inefficiency from wreaking havoc on operations. Key factors, like a lack of input from domain experts, the “dirtiness” of unstructured, raw data and the ultimate challenge of applying a model quickly enough to prevent a bad outcome, all combine to undermine this vision. Data context from the people who understand the industrial world, proper cleansing and alignment of this data, and the ability to apply insights in real-time transform the goals of data science from an unachievable ideal into reality.
Operations technology (OT) is the domain that manages industrial environments like factories, refineries, mines, etc., and is where all this valuable industrial data originates. OT staff are experts in these machines and processes, as well as the systems that control them. An OT person has deep domain knowledge, which can range from knowing what a failing machine sounds like to how different machine functions correlate to one another.
This expertise is the missing link in providing context and clarity to the vast amount of data points produced by machines and sensors. Without knowing how to interpret the data and some starting points of the relationship, data scientists are often left in the dark. To bridge this gap, OT experts must participate in creating the algorithms alongside data scientists without the need to program it themselves. This collaboration allows OT experts to easily convert raw data into meaningful results, eliminate bad data points and even begin to define correlations and patterns. Analytic tools that enable OT teams and data scientists to speak the same language are an absolute requirement.
Cleaning up raw OT data to reveal trends and insights is the next process, which is time consuming and tedious. Challenges as simple as sensors spitting out data points at different frequencies, or trying to pick out the 1% of data points worth analyzing from the terabytes produced, can be a gigantic time sink. In fact, much of the time data scientists spend at their craft is spent preparing and aligning raw data so algorithms can be applied. This is an unfortunate use of their valuable time and can often be a menial task. And if it must be done manually, then, by definition, it is happening after the fact. Applying a domain-specific streaming analytics language allows algorithms to natively execute on time-series data. This inherently solves the issue of putting disparate data sets in order. In addition, it automatically structures and allows for real-time data alignment, or converting raw data into relevant variables. This results in raw data that can be automatically aligned in under a second, allowing algorithms to trigger immediately and data scientists to spend their time on more important tasks.
To capitalize on the vision of data science, timeliness becomes a key factor. In other words, an algorithm that predicts when a company’s most valuable process is going to fail is not helpful unless the results can be generated fast enough to avoid an unplanned downtime. Unfortunately, the data science process usually entails sending raw data to the cloud and having data scientists manually cleanse and align the data before the valuable algorithm can be fed – losing precious time. Many industrial customers are predicting failures that had already taken place because of the delays in applying algorithms to the data. Automating data clean up and algorithm processes right at the edge and on-premise, with or without cloud connectivity, allows for no-latency results in less than a second.
The grand vision of data science can be overwhelming, but less unplanned downtime, brand new business insights and safer working conditions is possible. Hiring a fleet of smart, talented data scientists is not enough; they require a tool kit of proper processing and analysis tools – from data creation to insight application – to work more efficiently and implement their findings effectively and in real time.
Matthew C. King develops new innovative solutions in emerging technology spaces. Matt is responsible for evangelizing, designing and assuring success in new technologies, working closely with partners and flagship customers to define these categories. Matt brings over five years of technology experience to FogHorn Systems. He began his career at Talari Networks, formulating the initial major wins in software-defined WAN. In 2016, he helped boot-strap FogHorn and begin the process of defining the emerging IIoT edge computing space.
Matt graduated with honors from the University of California, San Diego in 2012. He holds a BA in economics and a minor in political science.
About the Author
You May Also Like