Stalled Enterprise AI Gets Unstuck from Surprising Source
Enterprises have stalled in deploying AI to production, but platform investments from commercial providers may help them wrangle their open source models.
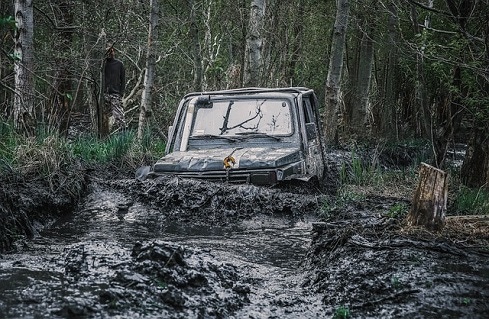
Open source technology really sparked a renaissance in data and analytics over the last 10 years: from data storage and management tech like Hadoop; to streaming data tech like Spark; and to languages for data science, advanced analytics, and machine learning, like R and Python.
Today, enterprise organizations are experimenting with and piloting machine learning and other artificial intelligence projects.
But even though open source sparked a new era of possibility and innovation, the technology offered has come as a DIY assemble-your-own toolkit. You need to put together your own enterprise-grade platform from a jumble of open source parts available. After all, that's how you did the pilot. While that may be how digital giants have always done it, that's not proven to be an easy task for traditional enterprise companies.
In 2017, just 4% of organizations had deployed AI in production, and that jumped by 10 points to 14% in 2018. But adoption slowed in 2019, rising just 5 points to 19%, according to Gartner's annual CIO survey.
These organizations need to build an enterprise data system that can manage their data and models at scale. It's one of the reasons that many enterprises have found themselves stuck at the pilot stage for AI and machine learning, unable to really pull the trigger on the value-generating production implementations.
But help is on the way from a surprising source, according to Gartner VP analyst and fellow Rita Sallam. The cavalry will come in the form of commercial artificial intelligence and machine learning platforms. This is one of the 10 major trends Gartner identified as coming to the mainstream data and analytics market for the next 3 to 5 years and presented by Sallam during Gartner's recent IT Symposium.
That may be surprising to some people, because open source tools enabled the data and analytics expansion of recent years. Yet the open source tools available today have lacked the enterprise grade features that many larger traditional organizations need, such as project and model management, transparency, and data lineage, Sallam told InformationWeek in an interview.
"At the same time you see many of the commercial platforms, particularly those emerging in the cloud, supporting the open source ecosystem," she said. They include Azure ML, Amazon SageMaker, and Google TensorFlow. "All are commercial platforms but largely leverage and integrate with the open source ecosystem. They provide the development environment, integration with open source, and a platform for processing models at scale. They provide the enterprise mission-critical features to help improve the productivity of expert data scientists and open up the potential for model creation to a broader skill base."
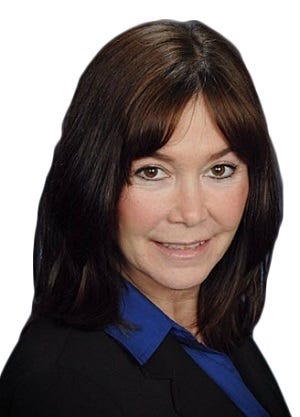
Rita Sallam, Gartner
While open source tools offer flexibility to data scientists and machine learning engineers, that flexibility comes at the expense of a disciplined system, according to Sallam. At the same time, cloud providers are trying to solve that problem. They can offer orchestration features with these platforms, they can offer planning and product roadmaps, and they can offer greater predictability to client organizations.
Cloud providers have identified AI and data science as a strategic area of focus going forward because their workloads are compute and data intensive. Attracting those workloads to the cloud would be financially lucrative to cloud providers.
There are also several providers of data and advanced analytics software platforms that have worked with enterprise companies for many years already, such as SAS and IBM. Sallam said that these companies have been investing in updating their efforts for a new era that has embraced open source tools. For instance, SAS announced in May 2019 plans to invest $1 billion in AI and its related components. The company introduced Viya in May 2016, billed as a cloud-enabled, in-memory analytics engine that is elastic, scalable, and fault tolerant. More recently, SAS COO and CTO Oliver Schabenberger told InformationWeek that the company wanted to help enterprise organizations with their stalled AI deployments with ModelOps and ModelOps Health Check Assessment designed to manage models created with any tool including open source tools such as Python or R.
IBM is also pitching its Watson technologies as the solution that can help enterprises get past the pilot stage into production with artificial intelligence, and Watson GM Rob Thomas has penned a series of blogs and done some time on the speaker circuit this fall to promote Watson's many technologies.
IBM and SAS's great advantage against the big three public cloud providers is that "they are actually running some of the world's most sophisticated models at scale," Sallam said. "They have models in production that are really difficult to replace."
That incumbency in vertical markets like finance and healthcare are strengths for companies such as IBM and SAS. Sallam noted that Oracle and SAP have also made enhancements to their own portfolios over the past several years to add AI and ML into the platform itself. Newer commercial providers include companies such as Aible, H2O.ai, and DataRobot, according to Sallam.
DataRobot product management executives Phil Gurbacki, Adam Weinstein, and Dan Darnell told InformationWeek in interviews that it is also helping enterprises with their AI production problems with model management and monitoring technologies.
"There are so many different models out in the world, so many different frameworks," said Weinstein. The DataRobot platform and technologies are designed to help organizations wrangle many different models, including open source models.
According to Gartner's Sallam, these commercial vendors do stand to gain as enterprises look to commercial solutions to help them move their AI into production.
"SAS, IBM, SAP, Oracle and other providers could benefit as the market shifts towards commercial platforms," she said. "But the primary beneficiary will be the cloud providers…they have momentum. In addition to the platforms themselves the marketplaces these vendors offer are an advantage."
Such marketplaces could enable organizations to easily buy and integrate models or data sets. That kind of set up removes complexity from the effort to deploy AI into production.
"It accelerates time and cost savings and enterprise readiness of machine learning models," Sallam said.
About the Author(s)
You May Also Like