Applying ML/AI to Sustainability Strategies
Artificial intelligence and machine learning have exploded in popularity, but one area these advancements have been transforming operations for some time is in climate technology.
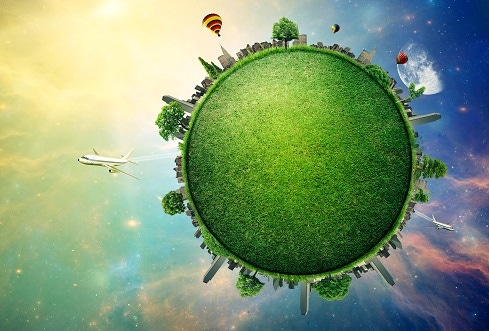
Artificial intelligence, machine learning, and examples of their many uses cases have exploded in recent months. These technologies will undoubtedly create new efficiencies and opportunities, with great potential to revolutionize the way we work.One area where these advancements have been transforming operations under the surface for some time is in climate technology, particularly by helping corporations set annual climate targets and forecast, report, and identity strategic measures they can take to reduce their emissions. To be clear though, this nascent technology is not a silver bullet for sustainability reporting and requires trained ESG professionals to work with it and critically think through its pitfalls and applications.
When governed by talented professionals, AI/ML technologies can be essential tools for automating and improving the accuracy of emissions reporting and setting an emissions reduction strategy. As companies embed these technologies, they should focus on minimizing the emissions associated with the ML/AI models while maximizing their potential to contribute to emission reductions across their operations.
Identifying Trends and CorrelationsBecause machine learning and artificial intelligence algorithms can process vast amounts of historical data on energy consumption, CO2 emissions, and various other factors such as production levels, climate patterns, and economic indicators, they can be helpful tools for sustainability experts to identify trends and correlations. With this information in hand, ESG experts can assess it and apply the relevant data to predict future emissions, develop complex scenario models, adhere to specific regulatory requirements, etc. Judgment calls like which data to use, which sources to collect the data from, the emissions factors to use, and the type of controls that need to be in place should be left to experienced specialists in the field, not the technology.The efficiency of these models and the people who understand the complexities of them can help corporations understand the potential impact of their emissions reduction actions before investing their time and capital into them. Arming organizations and their leaders with detailed information can then help them make informed decisions that account for regulatory, or stakeholder pressures they may be feeling and for the time and money they can realistically allocate to their emissions reduction strategies.
Scope 1 and 2 GHG Emissions
At KPMG, for example, we often advise organizations that are just starting their climate journeys to initially focus on their Scope 1 and 2 greenhouse gas emissions -- the sources that are owned or purchased by them -- when it comes to reducing environmental impact. Machine learning and artificial intelligence can help streamline this process, particularly for companies with nascent data collection processes.
For instance, according to the World Economic Forum, buildings and facilities alone account for 40% of the world’s greenhouse gas emissions. Leveraging ML/AI, an organization can identify ways to reduce their facilities’ emissions by working with these technologies to see which upgrades or retrofits might have the greatest potential for energy savings.
Taking it a step further, let’s say that same organization decides to transition some of their energy mix to renewable sources, such as solar or wind power. Machine learning and artificial intelligence can help the implementation teams optimize the integration of these renewable sources by forecasting energy production and demand more accurately. It can even trigger energy purchases based on certain thresholds.
Scope 3 GHG Emissions
Additionally, proper application of ML/AI should make tackling Scope 3 emissions, or indirect emissions that occur from sources that are not owned or controlled by the entity, less daunting as companies report more and as the technology evolves. Many organizations further along on their sustainability journeys already have trained staff who are working to identify high-emission suppliers, optimize logistics, and develop strategies to reduce emissions throughout their supply chains. Applying this technology could significantly accelerate these insights, allowing companies to take action -- and make an impact -- sooner.It's important that artificial intelligence and machine learning technologies be viewed less as a cure-all, and more as an exciting new tool for companies to leverage as they solidify their ESG technology strategy. By working with ML/AI technologies, companies can gain valuable insights, optimize processes, and make data-driven decisions that can significantly reduce their greenhouse gas emissions and contribute to a more sustainable future. Further, the organizations that are able to set realistic strategies will have the upper hand on competition as we move toward a low-carbon economy. And importantly, companies won’t necessarily need to build all of these technologies from scratch. An increasing number of climate technology software already has ML/AI embedded into their systems.
About the Author(s)
You May Also Like