4 Skills Will Set Apart Tomorrow’s Data Scientists
Skilled data scientists are in high demand as businesses adopt data-driven strategies. Certain skills will make them indispensable.
June 28, 2021
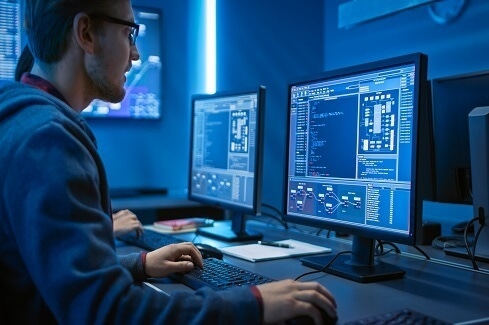
Data scientists are -- unsurprisingly -- in high demand across industries. This year, data science is the seventh most in-demand skill among the workforce.
The pandemic accelerated the adoption of data-driven strategies across industries. In retail, companies like Target turned to IoT and location data to inform their drive-up shopping strategies, simplifying the shopping experience and driving revenue in a tough year for brick-and-mortar retailers. Healthcare pivoted entirely, turning to data-driven insights to remain agile and responsive amid shifting medical supplies, filled ER beds, health screenings and other challenges. The financial services industry has utilized data for years to inform business strategies and solve emerging challenges.
While, data is an advantage now, in the future, it’ll be the foundation of all business. Data science will be a commonplace skill (and potentially a required one) beyond engineering and IT roles. To stay competitive, data scientists should invest in learning how to best translate data into actionable insights and compelling stories that will resonate across their business. These four skills will be critical to their success:
1. Communicating Probability, Statistics, and Other Branches of Mathematics
While data science is complex, the widening data literacy gap threatens innovation and collaboration across teams. Accenture found that 75% of employees read data, yet only 21% “are confident with their data literacy skills.” While organizations should invest in data literacy across the entire organization to boost productivity, today’s data scientists should learn how to best communicate the fundamentals behind data.
The ability to explain different concepts like variance, standard deviation, and distributions will help data scientists explain how data was collected, what the set of data reveals about that data, and whether it appears valid. These insights are helpful when communicating data to other stakeholders, especially the C-suite. With these fundamentals, you can easily explain the how behind data: How did you come up with these numbers? How do we know they’re valid and representative?
During these types of discussions, avoid going too far into technicalities. The best data scientists value simplicity and only add complexity as needed. To brush up on these concepts, check out offerings at online learning institutions and universities. Harvard University offers free online courses on topics like probability, as do popular e-learning platforms like Coursera.
2. Making Data Understandable
The ability to tell a story about data ties to the ability to simplify and explain the technicalities of data. Stories are innately more human than large swaths of numbers: One study found that 63% of an audience remembered stories when listening to speeches, but only 5% could recall just one statistic.
The best data scientists are also adept storytellers, providing the necessary context about data sets and explaining why the data is important in the larger picture. When sharing a new set of data or the results of a data project, focus on crafting a narrative on the top three things the audience should walk away with. Reiterate these points throughout whatever medium you choose --- presentation, email, interactive report, etc. -- to move your audience to action. This makes new information easily digestible and actionable.
3. Visualizing Data to Make an Impact
Visual mediums are a compelling but often undervalued way to communicate data. Consider the different types of graphs and charts examining various data sets for the pandemic. From maps tracking vaccine rollouts to Johns Hopkins’ collection of pandemic-related graphs, data visualization has proven to be a critical way to interact with and understand data.
The best visuals explain and contextualize large volumes of data, allowing viewers (especially non-technical stakeholders) to quickly digest information and spot key takeaways that may have been buried in the raw data. For data visualization, there are tools like Redash to create charts and graphs. For developing interactive maps, sign up for beginner seminars that teach data visualization with Python and other software. For creative inspiration -- books like Nadieh Bremer and Shirley Wu’s “Data Sketches,” following the work of Edward Tufte, or Tableau’s Public Gallery can spark new ideas.
4. Balancing Learning and Teaching
Tomorrow’s best data scientists will be both teachers and students. Find ways to build learning and experimentation into your everyday routine, reading the latest news on data techniques, or try a new software program. These approaches can showcase your value within an organization, but also offer a helpful perspective: Knowing how to approach information can improve how you share insights with others.
As more companies turn to data-driven insights to make smart business decisions, data scientists will take on more significant responsibilities within organizations. Conducting cutting-edge data science is only half the battle. Knowing how to explain your process and present findings in an engaging way will make tomorrow’s leading data scientists indispensable.
Ryan Boyd is a Boulder-based software engineer, data geek, authNZ geek, and developer relations executive. He is the Head of Developer Relations for Databricks, responsible for advocacy and experience around the Databricks Unified Analytics Platform as well as open source initiatives like Delta Lake, Spark and MLflow. Previously he led Developer Relations teams for Neo4j, Google Cloud Platform, and Google Apps. He is the author of Getting Started with OAuth 2.0, published by O'Reilly.
You May Also Like