5 Paths To The New Data Integration
Embedded, automatic and easy new approaches meet growing demands for do-it-yourself data analysis.
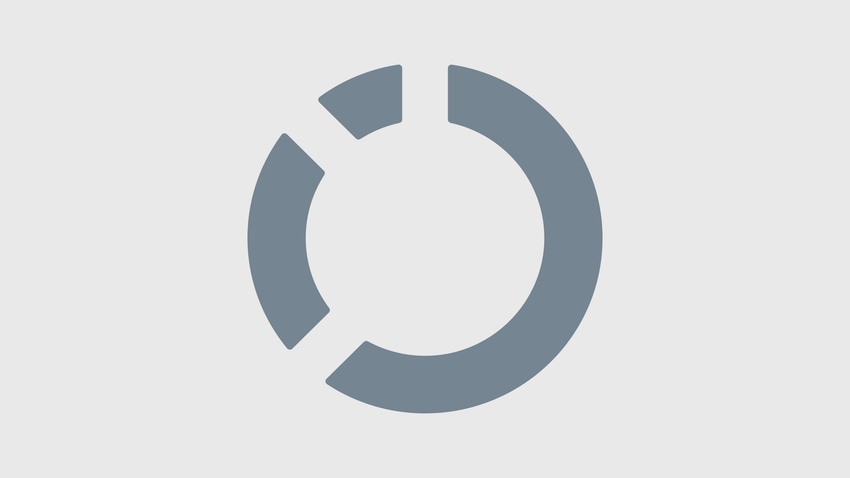
Google Sees Similarities
Google is, of course, king of open-Web mining, aiming to index the Internet-accessible universe. By adopting search facets like Endeca's, the ability to benefit from metadata, structure, and context in crawled content (for instance, page/document type, publication date, and localization), and content analytics techniques that include sentiment analysis, Google is transforming itself from a search engine into an information-access provider.
Rather than subject readers to a disquisition on Google, I'll point you to a 2009 paper that explains technical ins and outs of Data Integration for Many Data Sources using Context-Sensitive Similarity Metrics. Quoting the introduction:
"An important step in integrating heterogeneous datasets is determining a mapping between objects from one source and objects from another source, a step variously known as record linkage, matching, and deduping (among other terms) in the literature. One useful matching strategy is to use an appropriately thresholded similarity function, i.e., to consider objects as identical if they are 'similar enough'."
The Google authors talk of applications such as "merging the catalogs of many merchants" -- clearly their interest is in improving Google as an online comparison-shopping tool. Like FirstRain, they make application-appropriate assumptions, in this paper, that a catalog from a given merchant won't list a given product more than once.
The authors discuss "soft joins" based on statistical similarity measures, and they also see advantages if you can use "hard identifiers -- strings that can act as clear and unambiguous identifiers." They offer ISBN (publication number), UPC (product code), and Web URL examples.
A Uniform Resource Locator (URL) is a Uniform Resource Identifier (URI) that specifies an access mechanism such as HTTP and FTP. And URIs are the key to Linked Data integration across the the nascent Semantic Web.
Extractiv Spots Entities
Extractiv is a new company and service that joins Web-crawling technology from 80legs with semantic annotation and analysis software from Language Computer Corporation. Extractiv delivers software-as-a-service (SaaS) text analytics that identify "entities," such as names of individuals, companies, and places, as well as sentiment and relationships among entities found in source text.
A number of services, like Extractiv, support over-the-Web text (and sentiment) analysis; examples include Orchestr8's AlchemyAPI, Clarabridge, Evri, Lexalytics, OpenAmplify, Saplo, and Thomson Reuters' OpenCalais, and Zemanta. Some offer access to the Linked Data Web. You can see this capability via Extractiv. Here, I am using a Web API to annotate a White House blog page on the recent Tucson, Arizona shootings. Click on Gabrielle Giffords in the center pane and scroll until you see the Details area on the right. That area links, via a URI for Rep. Giffords, to a DBpedia page on the congresswoman, whom I chose for this example in order to honor her. This is an example of content enrichment made possible by the New Data Integration.
The DBpedia database contains structured information (e.g., data tables) extracted from Wikipedia. Check out the Gabrielle Giffords page directly and you'll see strengths that derive from the use of integration-friendly Semantic Web formats and weaknesses that include the incompleteness and the sloppiness, let's call it, of the presented information.
Click around the Extractiv-annotated page a bit more and you'll see other strengths and weaknesses: The service's ability to resolve expressions (that "almost 40 years" is a time duration), coreferences (such as the use of "she" and "her" to refer to the representative), and relationships (correctly parsing "Her husband, Mark Kelly"). Yet Extractiv did not identify "Gabby Giffords" and "Gabrielle Giffords" as a single person.
The Integration Road Ahead
There's work to be done to improve every system that deals with diverse, complex data. As my New Data Integration examples show, however, a variety of companies have made very significant progress meeting technical and business challenges. Expect more of the same -- advances toward easy-to-use, application-embedded, end-user-focused integration capabilities -- in the year to come.
Seth Grimes is an analytics strategist with Washington DC based Alta Plana Corporation and chair of the Sentiment Analysis Symposium.
About the Author(s)
You May Also Like