AI: It's the New Security Frontier
IT has principally focused its security on transactions, but with more artificial intelligence applications coming onboard. Is IT ready for data poisonings and other new AI threats?
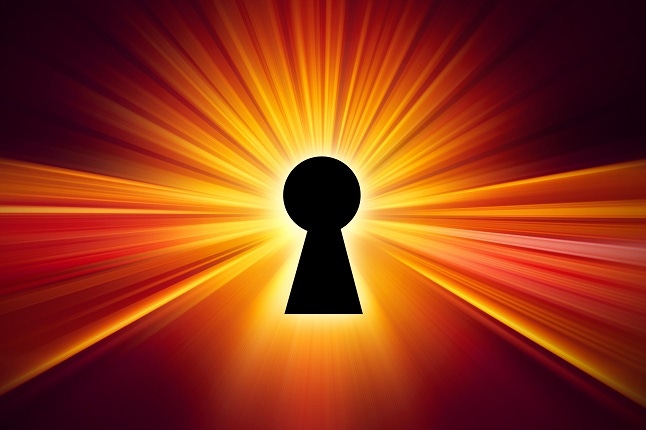
In 2021, Darktrace, a cyber artificial intelligence company, commissioned Forrester to conduct a study on cybersecurity readiness and AI. In the study, 88% of security leaders interviewed felt that offensive AI was inevitable, 77% expected that weaponized AI would lead to an increase in scale and speed of attacks, and 66% felt that AI weaponization would lead to attacks that no human could envision.
The prospect of AI security breaches concerns CIOs. In a Deloitte AI study, 49% of 1,900 respondents listed AI cybersecurity vulnerabilities among their top three concerns..
AI Security: Defending Against a Triple Threat
There are three major threats to AI systems that enterprises need to plan for. These threats range from data and software compromises to whom you partner with.
1. Data
Infecting data is a primary route for bad actors when it comes to compromising the results of AI systems. Commonly referred to as “data poisonings,” it's when attackers find ways to tamper with data and distort it. When this occurs, the algorithms that operate on the data become inaccurate and even erroneous.
Gartner recommends that companies implement an AI TRiSM (trust, risk and security management) frameworkthat ensures optimum AI governance through the maintenance of data that is trustworthy, reliable and protected.
“AI threats and compromises (malicious or benign) are continuous and constantly evolving, so AI TRiSM must be a continuous effort, not a one-off exercise,” says Gartner Distinguished VP Analyst, Avivah Litan
.
Central to this is making sure that the data that AI algorithms operate on is thoroughly sanitized, and that it remains that way. Security and observability software helps to ensure this, along with a regular practice of thoroughly cleaning and vetting data before it is admitted into any AI system.
A second tier of checkpoints is organizational. An interdisciplinary group should be establishing, drawing representatives from IT, legal, compliance and end users who are experts in the subject matter of an AI system. As soon as a system begins to display inconsistencies that suggest that outcomes or data are skewed, this team should examine the system and, if warranted, take it down. This is both a security management and a risk containment technique. No organization wants to fall victim to faulty decision making made from compromised data.
2. Machine language tampering
In a trial scenario, a Palo Alto Networks Security AI research team wanted to test an AI deep learning model that was being used to detect malware. The team used a publicly available research paper to construct a malware detection model that was intended to simulate the behavior of a model that was in production. The production model was repeatedly queried so the research team could learn more about its specific behaviors. As the team learned, it adjusted its simulated model to produce the same outcomes. Ultimately, by using the simulated model, the research team was able to circumvent the malware detection of an in-production machine learning system.
As AI system attacks grow in sophistication, more attacks on AI and machine learning code will occur.
One step that organizations can take is to monitor how much of their algorithmic or ML code is potentially available in the open-source community, or in other public sources. A second strategy is to ensure that any employees or contractors working on an ML engine and/or training it have signed nondisclosure agreements that would subject them to legal action if they tried to use the code elsewhere.
3. Supply chain governance
Most AI systems use a combination of internal and external data. The external data is purchased or obtained from third-party sources. For example, a hospital studying the genetic predisposition of its patients for certain ailments might use internal data gleaned from patients, but also outside data that can give them similar data from larger population samples. In this way, the hospital is assured that it has the most comprehensive and complete data possible.
In this example, the hospital can clean and vet its own internal data, but how does it know that the data it obtains from its vendor supply chain is equally trustworthy? The first place to check is the vendor’s security certifications and accreditations. Does the vendor have them, and from whom, and when were they issued?
Second, is the vendor willing to furnish the latest copy of its security audit?
Third, it is vital to check references. What do other users of this vendor have to say?
Fourth, does the vendor have non-disclosure and confidentiality agreements that it is willing to sign?
Fifth, is the vendor willing to accept a set of security-oriented service-level agreements (SLAs) as an addendum to the contract?
This is a general list of security items that should be checked off before entering any data purchasing agreement with an outside source.
Closing Remarks
The security of AI systems poses unique challenges as malicious parties discover new ways to attack these systems that IT have never been seen before. No one can yet predict how AI attacks will evolve, but it isn't too early to take stock of the security technologies and practices that you already have, and to adapt them to the world of big data.
What to Read Next:
How to Select the Right AI Projects
About the Author(s)
You May Also Like