Can IT Run a Data Science Function?
Some companies opt to place the data science group in IT -- but data scientists approach data and results differently. Can IT adjust?
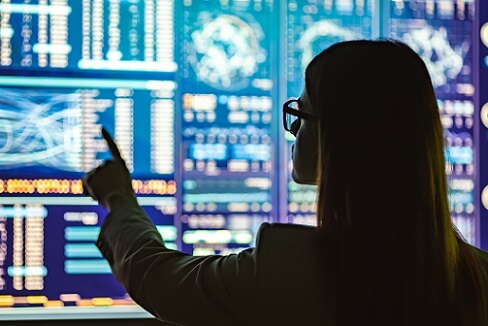
In January, career search firm Zippia reported, “24% of companies use big data analytics.” However, this was “significantly lower than the 37.8% of surveyed executives who said their companies were data-driven in 2020. Some people attribute this reduction to having difficulty solidifying data-driven decision-making as a part of their company cultures.”
There is no one reason for this lag in using data-driven decisions, but some of the reasons that we hear about include:
A belief that executives don't entirely trust data over instinct in their decisions
A lukewarm executive understanding of and commitment to a data-driven culture
Poor quality of data and its derivative results, which creates a general distrust of being data-driven
And corporate stumbles when it comes to effectively integrating data driven analytics into business processes.
We should also be clear about one thing: Most organizations (unless they are in education, life sciences, a research-directed field, or in pharmaceuticals) have not yet advanced to true data science.
Instead, they use analytics engines that are designed to complement and interact with their normal business processes. Often these analytics aren’t even of their own making. The analytics come pre-packaged, and are supported) by a third-party vendor, such as a CRM software provider that includes analytics reporting as part of its offering.
The Introduction of Data science
Nevertheless, companies are interested in expanding their analytics by deepening their approaches into the realm of data science, even if they don’t fully understand it.
The most common deployment has been to create a separate corporate data science group with its own management. In other cases, companies have chosen to split data science into subgroups that support their own “home” user departments such as finance. Then, there is a third deployment model, which deploys data science subgroups to different departments, but that also has a central “hub” group designated to coordinate all the work between the various subgroups.
But is this working?
In an industry highly reliant on data science, such as pharma, it likely is working. But for many companies, it becomes a struggle because:
Data science is an iterative discipline that doesn’t produce end results as quickly as IT can
Data science professionals are scientists; they are not necessary businesspersons or managers
Data science lacks governance, so data quality can become an issue
When these challenges assert themselves, the corporate tendency is to reorganize, and one likely reorganization move is to move data science into IT.
Running Data Science in IT: The Pro Argument
Moving data science into IT makes sense because so much of what is involved with analytics (and data science) is being integrated into business processes and systems. The IT group already understands the system and business integration issues. It also has competence in data stewardship and governance, which data science may lack.
There are other similarities between data science and IT. Both depend on storage and data processing capabilities, and the ability to manage and support these. Both require overarching architectures that define what gets hosted internally and what gets hosted in the cloud. Both need high quality data and continuous data governance and security.
Running Data Science in IT: The Con Argument
Despite the similarities, however, data science is still not the same as IT.
IT uses analytics, but the iterative development process of data scientists who continually refine complex algorithms without a guaranteed result or end point is hard to accept in IT, which is always under the gun to produce results.
Data science work doesn’t fit neatly into an IT project management routine, either. Nor does it use IT-driven development processes. Like IT system programmers, data scientists’ strengths are technical. They aren’t necessarily at ease communicating with end users or business analysts. This can make it difficult for data scientists and IT to develop a natural rapport.
The Best IT Strategies for Managing Data Science
Despite the obstacles, the fact remains that IT is the most logical area from which to manage data science if data science can’t sustain itself. Consequently, it could well be up to CIOs to manage both.
If this is the organizational outcome, here are three recommendations for CIOs who are being asked to manage data science:
1. Don’t try to change data scientists!
Like their systems programmer counterparts, data scientists are likely to be introspective and technically oriented. Their soft skills (i.e., ability to communicate, collaborate, negotiate, etc.) typically, are not their strong suit. In this situation, it is best to use a front line of IT business analysts who understand the business and the issues that users want to solve, and who can coordinate efforts between data scientists and end users.
It is possible that IT may need to add headcount in the business analyst group if it absorbs the data science function.
2. Build internal understanding on how data science works and what can be expected.
Most executives and end users have learned enough about data science to think that it has a place somewhere in the organization. They imagine that a data science discipline can take their analytics to a new level of insight, and they aren’t wrong in believing so.
At the same time, however, they only have experience with past IT work, so it is natural for them to expect the data science discipline to operate in the same way that IT operates with analytics.
They would see no reason why data scientists couldn’t produce timely analytics results with hard deadlines in the same way that IT does. Yet, that data science doesn’t work that way.
Data science is an iterative discipline that doesn’t necessarily guarantee impactful business insights in every project. The algorithms that data scientists postulate are best-case statistical attempts on how to get to information that has eluded companies for years. Unfortunately, not every postulate or algorithm works. Data scientists understand this, and they accept failure rates as part of their work.
The task for the CIO and other leaders is educating users and management on how and why data science is a different discipline than IT, and what the tradeoffs are for potentially deeper insights and solutions to elusive problems.
If the organization (especially executive management) isn’t willing to accept what could be a longer return on its investment, it might be best to stay with IT’s analytics and forget about data science until the right compelling use case comes along.
3. Know when to advocate for a separate data science function.
A biotech or a pharmaceutical company can reap immediate rewards from data science breakthroughs on new medical formulas that would take months and even years to arrive at without the help of data science. When this happens, millions of dollars in revenues and in operational savings can be realized. This is why most of these companies operate with a separate, mission-critical data science organization.
Other companies and industries are in earlier stages of data science adoption. They aren’t ready to deploy data science as its own department, or they may have tried and have been disappointed.
This doesn’t mean that there won't not be a future point when data science becomes as critical to these companies as it is in biotech or pharma today.
If and when this inflection point is reached, it’s the job of the CIO, the CEO and other leaders to recognize it and establish an independent data science function.
What to Read Next:
Q&A: How Data Science Fits into the Cloud Spend Equation
About the Author(s)
You May Also Like