Data Historians: The Plight of the Analyst
Data overload has made the plight of the analyst even harder. To make effective use of data, attitudes and approaches must evolve.
July 12, 2023
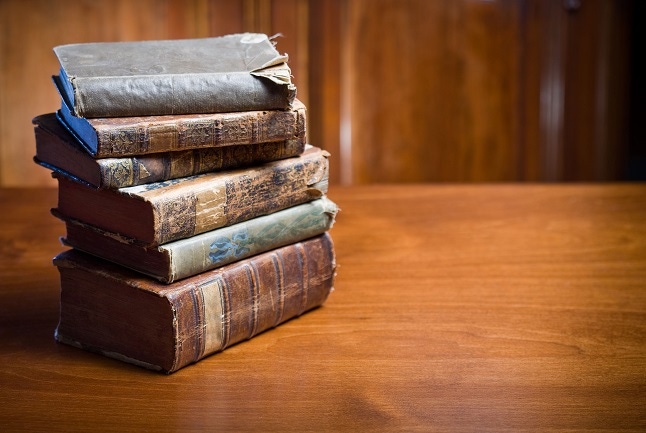
Data analyst challenges both have and haven’t evolved. Still problem solvers, they face the same old questions around whether investments drive sufficient bang for the company buck, especially from marketing chiefs and CFOs. But as the ‘more is better’ approach to data collection holds, finding actionable answers is getting harder.
With ever-growing volumes of information to manage, analysts have little time for anything other than re-telling recent history via descriptive analytics. And in an environment where CEOs arelooking for insights to help steer cost-cutting efforts and inform business strategy evolution, this means their capacity to offer useful value is worryingly limited.
What’s Keeping Analysts Stuck in the Past?
Being responsible for getting data into good shape now involves tasks once handled by data, or software, engineers. As well as gathering, onboarding, and processing multi-source data, analysts must keep a careful eye on privacy regulation. This includes navigating gray areas of global rules and overcoming legal hurdles to access data that might be considered sensitive.
From there, they face the difficulty of figuring out how to query and connect databases, in addition to developing, containerizing, and converting modeling structures into APIs so they can plug into evaluation or business intelligence tools, and begin making use of data.
All of which is time-consuming and takes even longer when the brief is “analyze everything”. Grappling with infinitely expanded workloads, analysts end up bearing an uncomfortable resemblance to the Wizard of Oz: Behind a slick exterior façade, they are really drowning in data and frantically pulling at different manual levers to make it look like data management happens by automated magic.
As a result, the final output is outdated when it finally hits the dashboards of business users. Take for example, data application in marketing teams. Across most sectors from auto to apparel, advertising campaigns have a short lifespan -- between two weeks and two months – where windows for change are limited. For campaign managers already struggling with the time it takes to shift budgets and adjust creative strategy, data that arrives late is the final issue that prevents them from harnessing ‘blink-and-you’ll miss it’ optimization opportunities.
Modern Stacks Aren’t Yet Smart Enough
Last year, McKinseyattributed issues with producing actionable data to two main causes: technological limitations (such as legacy systems) and the challenges of adopting modern architecture, as well as predicting that cloud-based tools would fix these problems.
In my view, this verdict isn’t entirely right. Uptake of cloud capabilities will likely bring some improvements to analysis efficiency, with data warehousing helping streamline merging of structured and semi-structured data. Considering therecognized prevalence of unstructured data, however, it’s also probable many challenges will remain.
Modern stacks are still prone to the same flaws that plagued early cloud systems;
usually containing multiple modular components that become complicated to coordinate and create a haphazard LEGO tower of mismatched bricks. We have seen too many cases where core challenges are driven by the tendency to view and manage data from these components separately.
A lack of integration between collation and activation tools such as Google Analytics fuels disorder and poor data quality, while forcing analysts to spend more time on data wrangling. On this point,other elements of McKinsey’s assessment are correct: using data expertise on manually exploring and connecting data is an unnecessary waste.
Minimize To Maximize — Productizing Data
At a general level, data coordination approaches need revamping. Organizations must create clear data strategies that not only determine what business needs are, but also what role each data producer and user will play in meeting these requirements.
Rather than collecting from every source, the goal should be taking the queries analysts receive from teams and working backwards to identify what is needed to answer them. Only at this stage will firms have the information they need to start iteratively developing a data model that delivers a truly valuable product.
It’s probable that implementing faster and more flexible orchestration methods will be part of this. Establishing an infrastructure where insight is instantly harmonized and transformed in line with specific needs will drastically lighten the load for analysts.
Data overload has made the plight of the analyst even harder. As well as grappling with an ever-expanding range of disconnected tools, they are fighting to wade through endless data streams and pull meaningful insights -- often generating a recap of what marketing, product, and finance teams have already figured out. To make effective use of data, attitudes and approaches must evolve. Streamlining core handling practices will make a major impact on processing times, and significantly ease the analyst burden. But to switch from history-telling to future-gazing, they’ll also need to build data pipelines with practical use in mind.
About the Author
You May Also Like