Distorted Netflix Rental Data, Online at NYTimes.com
I'd like to like the New York Times's on-line visualization, "A Peek Into Netflix Queues." I'm a big fan of the paper and its infographics, but in the end, Netflix visualization strikes me as glitzy rather than informative, a misleading graphical tarting up of incomplete data. I will explain where Netflix and the Times went wrong...
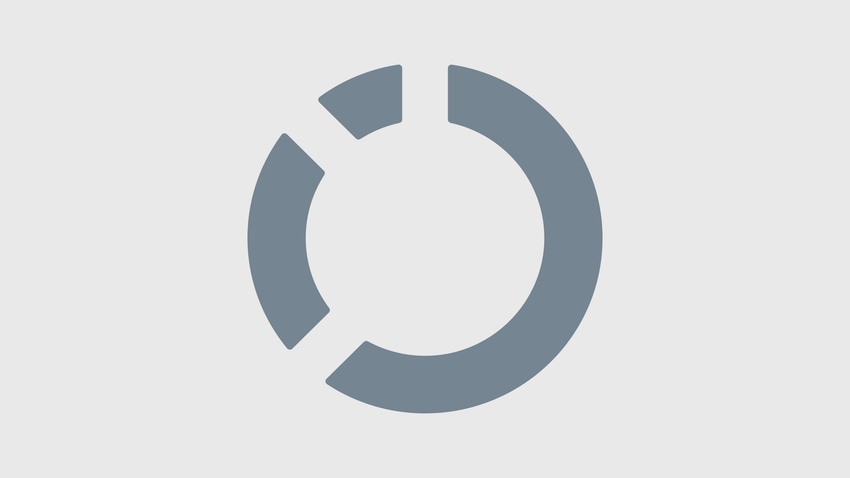
I'd like to like the New York Times's on-line visualization, A Peek Into Netflix Queues. I'm a big fan of the paper and its infographics -- witness my True BI for the Masses -- but in the end, the Netflix visualization strikes me as glitzy rather than informative, a misleading graphical tarting up of incomplete data.
Score one for Netflix' publicists: The Times imprimatur validates the data, relative 2009 popularity of movies for rent by ZIP code, yet the data is incomplete and therefore not what is claimed. The result is a pretty but false picture. It does NOT present correct relative Netflix rental popularity for 2009 as claimed. Do check out the visualization, which does offer nice interactive features, and I will explain where Netflix and the Times went wrong.There's much to like about the Times' Netflix visualization. The Times presents data we can all relate to, relative movie rental demand, in a form we are all familiar with, geographical maps. The presentation contrasts data across and among major metropolitan areas: it's not just stats without context. Further, it's interactive. A slider and buttons let you move from one movie to the next via multiple sort orders: Most rented, Alphabetical, and By metascore, a cumulative critics' rating. There are capsule reviews linked to full New York Times movie reviews. Lastly, this visualization is a great example of a Google Maps mash-up and includes a mouse-over effect that generates a pop-up with the top 10 rentals, plus the position of the rental you're explore if it's not among the top 10, for each particular ZIP Code area.
Now the Times isn't claiming to be doing business intelligence, but still, the Times's journalism standards are second-to-none, and good journalism and good BI have a lot in common, hence this critique. The visualization is fun to play with, but it's based on data that is too shallow to be newsworthy. Further, the data is not what it seems and the visualization approach distorts the information the visualization does convey. Start with the article title and ask yourself, What, exactly, are we looking at? The title refers to "Netflix queues," implying that the data represent movies that Netflix subscribers have requested to rent, yet the text says the data represent "100 titles that were frequently rented from Netflix in 2009." A snapshot of the queues would be good data; a comparison by rental frequency decidedly is not. That's because the list includes, for example:
The Bucket List, released on DVD on June 10, 2008.
The Curious Case of Benjamin Button, out on DVD on May 5, 2009.
The Proposal, out on October 13, 2009.
The Netflix data, if representing full-year 2009 rental numbers as reported by the New York Times, apparently compares data for a movie available for twelve months of 2009 against a movie available for less than three. The Times does not report this data deficiency. If Times staff and editors were aware of this variable yardstick, they should not have published this visualization. If they were not aware, they should have been. If, instead, somehow the data was properly normalized, then the text accompanying the visualization was wrong.
How might they have normalized the data? Here's one way: Whichever movie came out last in 2009, take the amount of time it was out and count the rentals of each move over that amount of time following its release. Rank those part-year numbers to get a fair, albeit not full-year, top 100 list that better reflects relative popularity.
Moving on, what of the data presentation?
The data give rental rankings, not number of rentals. Fine, for any particular ZIP Code area, and fine also for a quick comparison of one ZIP Code area to another. Not fine, however, for a deeper look at the data. First off, the geographic area covered by particular ZIP Codes varies very significantly, from a few blocks in Manhattan to tens of square miles in rural areas within metropolitan areas. There is a varying but generally weak correlation between ZIP Code area size and population. These facts mean two things:
A yellow-painted lower ranking for one area may mean more Netflix rentals of a given movie, in absolute numbers, than a red-colored higher ranking for that same movie in another area.
The color mass for a larger geographic area will deliver a stronger impression than the color mass for a smaller area regardless of the meaning of the rental rankings for the two areas.
As an example, ZIP Code 98052 in the Seattle area carries more visual weight than the geographically much smaller ZIP Code 98012 in Seattle. Without a means of weighting the data by population and/or actual number of rentals, we have distortion, the same kind of distortion we have seen in recent national elections where red Republican-voting areas are larger than blue Democratic-voting areas, visually inverting the proportion of actual voting.
The remedy here is to better describe the data being delivered, to offer an additional, alternative view that mashes up the data on a cartogram. Mark Newman of the University of Michigan, in a page on the 2008 U.S. presidential election vote, shows how: several ways, including ones I haven't mentioned, use of a nonlinear color scale.
The New York Times gets it right far, far more often than it doesn't. The Netflix rental visualization, while graphically appealing, is one of the paper's infrequent clunkers. I hope we can learn from it.I'd like to like the New York Times's on-line visualization, "A Peek Into Netflix Queues." I'm a big fan of the paper and its infographics, but in the end, Netflix visualization strikes me as glitzy rather than informative, a misleading graphical tarting up of incomplete data. I will explain where Netflix and the Times went wrong...
About the Author(s)
You May Also Like