Hallmarks of High Data Maturity
Data maturity is synonymous with business transformation. By understanding your data maturity in four areas, you can pursue it alongside enterprise goals.
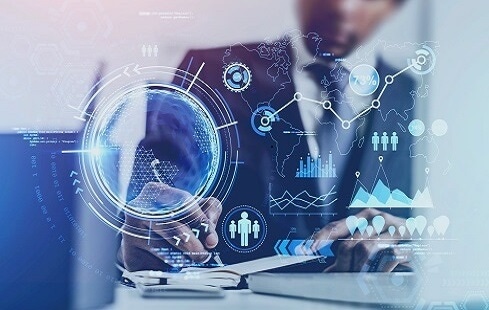
Data is universally accepted as a key asset for enterprises today, and the adoption of the right data ecosystem and processes can be a tremendous boon to company prospects. A company absolutely must be aware of what the data marketplace is making possible and, with discernment, actively engage the process of adoption.
At all times, enterprise data maturity is either going up or down. Momentum up the model is essential for success but keep in mind the model will evolve over time. No plateaus are comfortable for long.
Maturity levels tend to move in harmony across categories. I use organization, technology, architecture, and processes as categories of data maturity. I’ll share some examples of the hallmarks of high data maturity in the categories.
Organization: For the organization category, you will want to have data governance encompass the entire enterprise -- all domains, all departments, all systems. This should be pervasive in the enterprise culture and a touchstone for all decisions. Effective data governance is an enabler for improving data maturity.
Technology: So much data technology is required to be put to work in an enterprise today, and so much of it revolves around enabling an organization to be an artificial intelligence leader. To that end, databases at the edge in IoT architectures, embedded databases, pervasive master data management, and self-describing data are evident in highly mature organizations in 2021.
Architecture: Sensible allocation of workloads to platforms is a requirement. At the least, this means data warehouse, data lake and master data management platforms are present, operating, and mature. Data integration between platforms should be automated, real-time streaming.
Processes: This is a big challenge for many as it involves people more than any other category. Highly mature organizations ensure all development is done with “loose fitting” but a nonetheless present set of development guidelines. Also, in mature organizations, data is understood by the executives to be a strong asset, with there even being the occasional reference to data in financial disclosure statements.
Unless you’re a 5 out of 5 across the board, continue your journey by delivering business wins utilizing higher-level elements of maturity such as these, and thereby increasing your data maturity. You must deliver business wins while you improve data maturity.
For example, let’s say there is a customer churn project being planned. The planned methods are batch data collection of maintenance records and SQL coding to look at what customers are approaching that time when seemingly they need outreach. This can be changed to an automatic assessment of all touchpoints in real-time with automatic accumulation into analytic stores where, using machine learning, the customer’s next moves, unabated, are known. This can be translated into the best way to influence the customer. This approach yields a business win as well as improves data maturity.
Each level of data maturity corresponds to not only increased data maturity but also increased organizational maturity and bottom-line ROI. With a maturity model, enterprises can self-assess data capabilities in organization, technology, architecture, and processes categories, yielding an overall view of the current level of data maturity. This is highly impactful on next steps.
Related Content:
Why You Need a Data Fabric, Not Just IT Architecture
About the Author(s)
You May Also Like