Machine Learning Can Extend Life Of Flash Storage, Paper Finds
Industry consultant Tom Coughlin writes in a new paper that machine learning will extend the life cycle of flash memory, which experiences "cell wear" with repeated use.
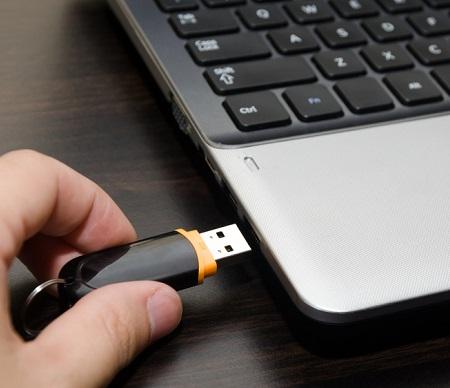
6 Machine Learning, AI, Analytics Trends To Watch
6 Machine Learning, AI, Analytics Trends To Watch (Click image for larger view and slideshow.)
Flash memory is being drawn into the mainstream of enterprise storage, but its tendency to deteriorate with use remains an Achilles' heel. A paper released at the Aug. 9 start of the Flash Memory Summit in Santa Clara, Calif., finds that machine learning can counteract that deterioration and drastically extend its life cycle.
The paper was written by Tom Coughlin, president of Coughlin Associates (PDF), a solid state consultant in Atascadero, Calif. He is also general chairman of the summit. The paper was sponsored by NVMdurance, a Limerick, Ireland, firm that is applying machine learning in the software it creates for managing solid state devices.
Using machine learning to prolong the useful life of high-capacity SSD systems is a new field.
The fact of that use of flash memory cells results in their physical deterioration as holders of electronic charges (which get translated into digital bits) can't be reversed. However, Coughlin argues that machine learning can understand the pattern of how the solid state device is being used and rejigger registers and voltages to maximize device longevity.
With the complexity and scale of today's SSDs, "the task becomes impossible to do manually," Coughlin wrote in his introduction to the machine learning concept.
Flash memory works by storing a charge on a floating gate, which can be described as a charge trap. To load the trap, a known voltage level is needed to push electrons through a layer of insulation that allows the cell to hold the charge after the current is taken away.
A key characteristic of flash is that less voltage is needed to load the trap when the memory cell is new. The voltage used may range between 7 and 12 Volts, Coughlin noted. Use of the cell tends to degrade the insulation layer, "making it harder to keep electrons on the floating gate," he continued. Higher voltages are needed as the cell ages, but they result in more degradation of the insulation.
"As electrons leak off the gate over time, this changes the voltage on the floating gate and also leads to bit errors," Coughlin reports. Knowing the rate of leakage becomes a way to predict how long the data in the cell will remain intact. The more frequently the cell is programmed and erased, the weaker the insulation layer becomes, and the life of the device as a whole is gradually shortened.
The process of electrons tunneling out of a charged cell through the insulation and into a neighboring cell is what is known as signal to noise ratio (SNR). The SNR must be kept in check for the flash device to know its data is intact and can be read accurately. Device makers invest heavily in error correction codes that can overcome the noise levels and confirm accurate data is being transferred.
The issue affects NAND devices being widely used today, Coughlin wrote.
"NAND cells are susceptible to bit errors," and NAND device makers add parity bits that tell the controller -- a built-in flash device microprocessor -- what the data being retrieved should look like. An error detection and correction engine on the controller can then verify the bits or recover small amounts of leakage-disrupted data.
The controller relies on registers that tell it which sequences of data were stored in what sectors they occupy on the memory chip. A single-level flash may have 50 registers -- a complex set to manage but still within reach of a human programmer.
But today flash is being manufactured with multi-level cells that contain four distinct voltage charges in each cell, or even three-level cells, leading to the need for a 1,000 registers of critical location information, a number likely to be beyond the grasp of a single programmer.
All the issues of flash operation are exacerbated by the growing complexity of the memory chip. Multi-level cells have a lower tolerance for signal to noise ratios; the more levels, the lower the tolerance, Coughlin wrote.
"As the levels of charge on the floating gates becomes smaller and smaller, the impact of a few electrons migrating from one floating gate to another becomes more and more significant."
By applying machine learning to the characteristics of the memory chip and the patterns in which the data is being stored, a model can be built of how the chip is functioning and how it might function in a revised pattern that could extend its life.
Putting Theory to Use
NVMdurance, the firm that sponsored the report, builds Pathfinder and Navigator software.
The first determines optimal register settings for use in a neutral lab environment. The second watches the operation of the device in the field as it employs the lab register settings, and collects feedback from use and health monitors.
The Pathfinder then constructs and tests new models based on its predictions of how to gain greater longevity for the type of use the device is getting. If the new model tests out, its registers gradually replace those initially put into use.
"This process is repeated as many times as needed to find more useful candidate solutions," Coughlin wrote.
In the NVMdurance version of machine learning, a machine learning engine called Plotter uses the test data to tune the registers of the device in use. NVMdurance is an early participant in the field of using machine learning to SSDs, but it seems likely that once it's understood there's a way to increase device longevity, fresh startup competition will emerge.
The approach allows an automated engine to look at voltages being used at the beginning of a device's life and minimize them as long as possible so as to minimize what's known as cell wear, or the gradually increasing leakage of electrons from SSD cells. The approach also allows more predictability for the time that a NAND storage device must be phased out.
[Want to see how flash has become more mainstream? Read IBM Introduces Three General Purpose Flash Arrays.]
What Coughlin didn't say was how much longevity is gained through the application of machine learning.
However, NVMdurance moved to it after capturing flash optimization techniques and expressing them in algorithms. The company was founded in 2013 at the National Digital Research Centre in Ireland when a flash research group, ADAPT, joined forces with an equipment maker, Evolvability Ltd.
Together they represent 15 years of research and foundry work in flash memory. NVMdurance optimization methods were originally applied manually, but the complexity of flash has outstripped that process.
Coughlin is an established analyst in digital storage, publishes the Digital Technology Storage Newsletter, and is the author of Digital Storage in Consumer Electronics: The Essential Guide, published by the Newnes Press in 2008. He is a holder of six patents in the field and is a Region 6 IEEE director.
The 2016 Flash Memory Summit continues through Wednesday at the Santa Clara Convention Center.
About the Author(s)
You May Also Like
How to Amplify DevOps with DevSecOps
May 22, 2024Generative AI: Use Cases and Risks in 2024
May 29, 2024Smart Service Management
June 4, 2024