Penn Medicine: Using Data To Save Patient Lives
Penn Signals is a system that uses existing data from electronic health records to perform real time predictive analysis of heart failure patients. The goal? Penn Medicine wanted to place patients in proper risk groups and assign them to cardiology resources in order to get them the best care and improve their outcomes. This work earned the company the No. 4 spot in the 2016 InformationWeek Elite 100.
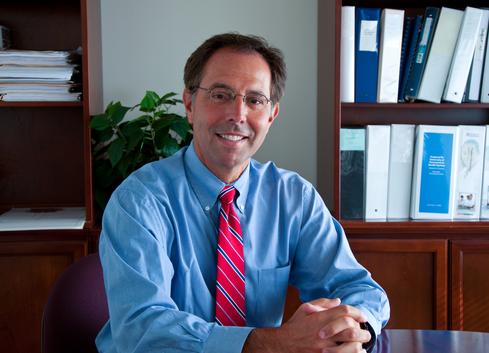
10 Big Data Books To Boost Your Career
10 Big Data Books To Boost Your Career (Click image for larger view and slideshow.)
Heart failure is serious business. According to the Centers for Disease Control and Prevention, half of all people with heart failure die within five years of diagnosis, and treatment in the US costs the nation around $12 billion every year. Philadelphia-based Penn Medicine, the country's oldest hospital organization, decided to use big data to do something about it.
Penn Signals is a system that uses existing data from electronic health records (EHRs) to perform real-time predictive analysis of heart failure patients. The goal? Penn Medicine wants to place patients in risk groups and assign them to cardiology resources in order to get them the best care and improve their outcomes.
The change from paper records to electronic ones provided an opportunity to do more than just change the medium for patient files, said Dr. Bill Hanson, chief medical information officer at Penn Medicine, in a telephone interview with InformationWeek. Penn Medicine wanted to use newly digitized records to improve patient care, Hanson said, and "one of the ways was to enable the backend systems to constantly scan to provide information that the frontline provider might not see or might not have access to. It's in the same way that Netflix or Amazon might look for patterns in what you watch or buy to offer suggestions."
Penn Medicine, which is made up of the University of Pennsylvania's Perelman School of Medicine and the University of Pennsylvania Health System, has more than 2,500 hospital beds and more than 31,000 employees. Penn Signal's foundation was laid in a decision that precision medicine was a goal of the organization, said Mike Restuccia, Penn Medicine VP and CIO.
"It's a hard decision, and it requires some strength in leadership. Our dean took the decision that precision medicine was the goal and led us in that direction," Restuccia said. "It ruffled some feathers. Sometimes it works and sometimes it doesn't."
That decision has ended up guiding the actions of Penn Medicine's roughly 600 IT employees, Restuccia said. "Our strategy … was we wanted to have common systems across the enterprise, centrally managed and collaboratively installed, with the understanding that once we had the foundation in place, we could then build off the foundation to do useful things with the data."
Finding Patterns That Save Lives
The "useful things" that Penn Signals was created to do revolve around improving the results for heart failure patients. Clinicians (physicians who see patients, rather than research physicians who work in labs) wondered whether there were patterns in patient data that could identify heart failure patients earlier, identify various levels of risk in existing heart failure patients, and help clinicians do a better job of assigning those patients to care teams and treatment protocols.
Getting to information that clinicians could act on meant diving into big data, and that required data scientists. Penn Medicine began by hiring Mike Draugelis as its chief data scientist. The opportunity to have access to both rich data and clinical professionals drew Draugelis away from a career in aerospace and national defense and into healthcare, said the former chief data scientist at Lockheed Martin.
"When we started this project, the chief medical officer of the hospital asked the newly formed data science team to work with the service lines, and the heart and vascular team was the first," Draugelis said. Cardiac care was chosen first because the clinicians there knew they had a problem, he explained.
When the cardiac team can properly treat a patient in the early stages of heart failure, the results are much better. But many patients were coming into the health system and never being referred to the cardiac team because they came to the hospital for an issue that had nothing to do with their heart.
"The first thing we did was look at how many people were coming through the system not identified with having a chronic heart problem," Draugelis said. The team then looked at all the information in the electronic health records to see which of those patients had the markers for heart failure. If the markers were there, then the patient could be flagged for review by the cardiac team.
Building the system meant combining the strength of the data and the data science team, Hanson said. "We had to invest in [the data science team], building the engine that spots or creates the patterns that say that a patient is at high risk for readmission, but we were leveraging the existing EHR system," he said. "That includes all the demographic information, the lab information, and the diagnostic information that goes with the record."
Embedded Teams
Draugelis' team of six data scientists and developers, working alongside the clinicians on the project, looked at eight years of clinical data to create the algorithms needed to correctly identify patients' risk levels. "When you're doing this kind of development, it's important to have the team embedded with the clinicians so we can have the conversations and converge on the best plan for the patients," he said.
"It's not just the data science team that makes this successful. It really is an integrated team with the IT group and the clinical teams that provide the concept that they want to bring to the patients," Draugelis said. The fact that each of those teams can be small is another piece in keeping with Penn Medicine's general philosophy of IT management.
Brian Wells, associate VP of health technology and academic computing at Penn Medicine, leads the teams that provide the technology supporting Penn Signals. "We like the idea of small teams," Wells said. "Mike [Draugelis] has a small team of two or three people involved in this. The clinical team that validates the results is small. We feel that teams of two to four people are more efficient."
The technology those small teams are providing is largely homegrown and flexible. All the clinical data, both historical and real-time, is in Penn Data Store, a system built on Oracle and IBM Data Stage that accepts and transforms the data every night, Wells said.
Brian Wells, associate VP, health technology and academic computing at Penn Medicine.
"It's billions of rows of data and millions of rows every night, so it's about a day behind," Wells said. Data comes out of this data warehouse via a system developed in-house called Clinstream. For Penn Signals, Clinstream transfers the data into a big data system built on MongoDB.
The work of the small teams collaborating with one another has been an effective system for patients. "In terms of the accuracy and specificity of identifying the risk, it's exceeded our expectations," Hanson said.
What's Possible
This is only the beginning. "Where we've lagged is figuring out how to put [together] the end-to-end process of how to ingest data, spit out results, and deliver the results to people to get the right outcome," he said. "There's a continuum of things there, some of which are technical, some of which are workflow, and some of which are people. We're going to be continuously learning how to do that for the next decade."
In addition to continuing to learn how to make better use of the data at Penn Medicine, the organization's executives said they hope to spread the word about what is possible through data analysis. "One thing that I really want to make other health systems aware of is that they can do this too," Draugelis said. "It's hard, but not as hard as people think it would be. The keys are having the data, having the clinical team that's ready, and having them integrate the science with their patients."
Restuccia agreed that more health systems should be data driven. "At the highest level we feel institutionally that, if a health system isn't mining its data to guide clinical care, it's like leaving money on the table in a poker match. It's a shame that you did it," he said. The teams are critical, Restuccia added. "You have to have almost hand-to-hand, shoulder-to-shoulder monitoring of the initial results to make sure they're what you thought they would be," he said. "If they're not, then tweak the systems to bring them up to your expectations."
The upcoming generation of doctors is eager to embrace the clinical opportunities embedded in EHR data, Hanson said. "We're seeing a lot of interest from medical students and residents who believe they have models we should be experimenting with. Not surprisingly the younger people in training are the ones with the ideas about how we can take advantage of data to improve care," Hanson said.
IT will have to be prepared to support those doctors, Restuccia noted. "It's going to become the norm. I am not a clinician, but it seems to me that, in the past, practicing medicine was as much an art as a science," he said. "The answers are in the data. The art is how you apply the data, and now clinicians have more data than ever before."
About the Author
You May Also Like