AI-Powered Security: Lulling Us Into Misplaced Confidence?
While artificial intelligence and machine learning hold promise in cybersecurity initiatives, there are some gaps that enterprises need to consider.
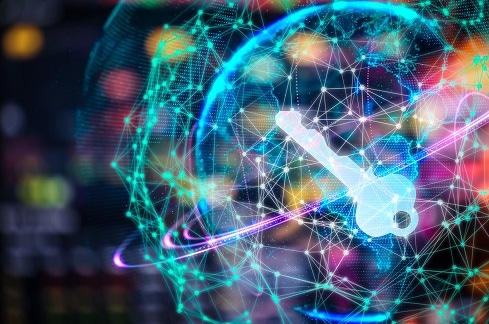
Everybody’s touting artificial intelligence as the new cybersecurity bulwark. For anti-malware and anti-hack defenses, AI’s power lies in its ability to proactively monitor, identify, and remediate almost any type of cyberthreat.
Automated systems can't have hard-and-fast rules for detecting the zillion potential cybersecurity attack vectors. But they can use AI’s embedded machine learning models for high-powered pattern recognition, detecting suspicious behavior and activating effective countermeasures in real time. For example, ML-based defenses can proactively isolate or quarantine threatening components or traffic after determining that a website is navigating to malicious domains or opening malicious files, or after sensing that installed software is engaging in microbehaviors that are characteristic of ransomware attacks.
However, if you think that AI is a security panacea, you should pay closer attention to what this technology actually requires to be effective. The core approach of modern AI is supervised learning, which involves using data that represents the phenomenon of interest in order to train ML models built on artificial neural networks. Considering all the possible threat vectors that cybersecurity solutions must address, IT professionals need to realize that no single ML model can possibly address them all.
Put simply, ML is a fragile pillar upon which to build our hopes for a truly secure digital world. As cybersecurity specialists increase their reliance on such defenses, they need to be aware of the technology’s vulnerabilities on several levels:
Failing to deter zero-day attacks: The threat of “zero-day attacks” -- for which no effective anti-malware solution exists -- hangs over ML-based cybersecurity solutions, just as it does over older signature-based cybersecurity defenses. After all, you can’t train an ML model to detect a threat for which there are no extant examples in the historical record. In addition, your security specialists probably wouldn’t be able to anticipate some of the more ingenious potential threats to the level of detail that would be needed to build “synthetic” data examples that might represent them accurately enough to train the requisite ML models.
Monitoring behavioral patterns that are too narrow or broad: Real-time anomaly detection is the heart of AI-powered cybersecurity. ML detects and blocks abnormal behavioral patterns involving endpoints, or in the network, or in how users interact with devices, applications, and systems. Having an ML model that has learned only one rigid behavioral attack vector makes a cybersecurity program vulnerable to dynamically changing attack signatures. If the ML-learned normal attack vector is too broad, it’s at risk of blocking an excessive number of legitimate behaviors as cybersecurity attacks. If that pattern is too narrow, the cybersecurity program is at risk of permitting a wide range of actual attacks to proceed unchecked.
Using unrepresentative training data sets: A cybersecurity program’s embedded ML algorithm can only learn from the reference data that is fed into it during the modeling and training process. If that data doesn’t fully represent the environment whose behavior the AI algorithm is trying to learn, the resulting program will be ineffective in detecting and blocking many actual cybersecurity incidents. Learning only from one reference data set tends to make AI-driven cybersecurity quite brittle. It’s always best to train against many training-data sets upfront and retrain against fresh data on an ongoing basis throughout the life of the program.
Incorporating predictive features that don’t address the likely threats: Machine learning is only effective at identifying cybersecurity issues if it’s looking for the specific features or independent variables associated with attacks. If hackers can identify the features that an ML model is using to flag malware, they can make every effort to avoid or obscure its use of those in their programs. But if cybersecurity algorithms can continue to explore for otherwise unprecedented features in attacks, they can build ML-driven anti-malware that can adapt to dynamically evolving threats, hopefully before the damage is done.
Built and trained within compromised data-science pipelines: ML training is neutered when the data is devoid of any records that represent anomalous cases. If cybersecurity ML is built and trained on such data, anti-malware programs will not detect attacks and will leave applications entirely exposed and vulnerable. To the extent that hackers have corrupted the data-science pipeline to prevent attack data from being ingested, aggregated, and managed in data lakes used for cybersecurity modeling, they will have crimped the efficacy of the anti-malware programs that leverage these models. And if the invaders can change the labels applied to data examples, they can throw cybersecurity programs entirely off the trail.
Relying on only one data algorithm as the guts of the cyberdefense model: ML-based cybersecurity defenses are at risk if they rely on only one, single, master algorithm, which may be susceptible to being fooled or hacked in ways that other algorithms aren’t. For stronger cybersecurity defenses, it may be best to build multiple ML models using diverse algorithms and training data sets. Each of these models can be used to monitor the same threats, serving as mutual backstops with their various vulnerabilities offsetting each other in production environments. In addition, the various models can be deployed in “divide and conquer” mode, each built and trained to watch for specific attack-vector behaviors at endpoints, in the network, and/or in user behaviors.
Ignoring the fact that hackers are using the same tools to mount ingenious assaults: Hackers are using ML to launch more sophisticated attack campaigns. One such tactic is “spear phishing,” in which ML-based natural language generation enables cybercrooks to send bogus but real-looking messages that dupe victims into giving the attacker access to sensitive information or installing malicious software. Another is polymorphic malware, which uses ML to learn, iterate, and fine-tune its assaults based on what proved most successful on prior attacks.
Where these ML vulnerabilities are concerned, your enterprise may be relying on cybersecurity vendors -- such as Crowdstrike, Fortinet, Palo Alto Networks, SparkCognition, and Symantec -- to implement effective risk-mitigation procedures within their product development and quality assurance practices. As you investigate these solutions, perform due diligence on vendors’ sensitivity to these vulnerabilities. You should demand a full accounting of how the solution providers are comprehensively addressing these issues.
Don’t let security vendors present AI/ML-driven defenses as a silver bullet when, in fact, they’re really a double-edge sword of cyberthreat.
About the Author(s)
You May Also Like