Building the Foundation: What Stands Between AI Hype and Reality
Learn how businesses can navigate the complexities of AI implementation and unlock its full potential by maintaining focus on desired outcomes.
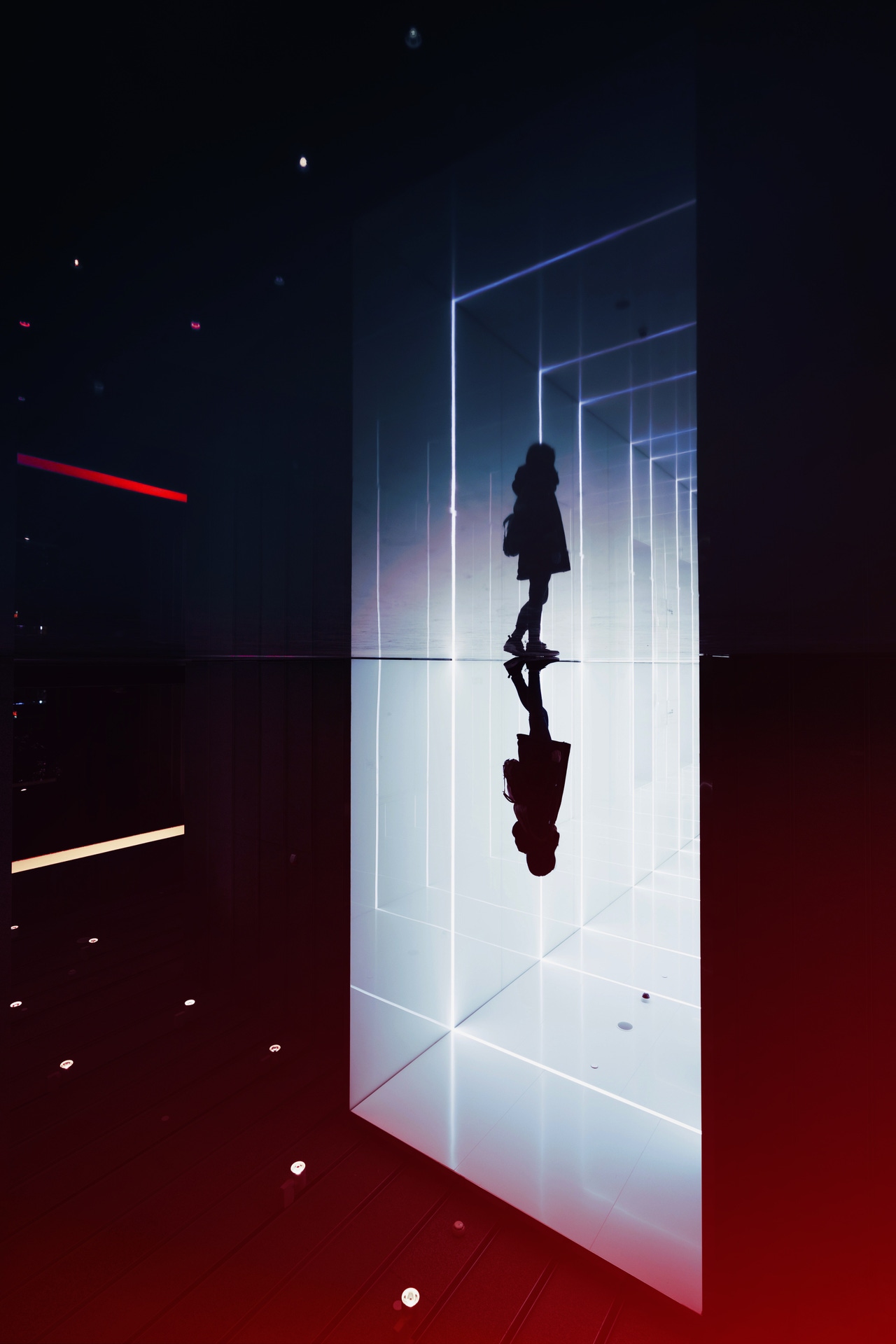
Sponsored by Lenovo
From our own CIO Study and the research of our industry peers, we see nearly every company is deploying AI and generative AI (GenAI), either across departments or within individual teams. However, successfully capitalizing on the technology and achieving business results are far from guaranteed. Adding to the uncertainty, as an industry we lack consensus or established best practices on where to start with AI. Or how to take it to fruition. Or anything in between! Instead, IT and business scrum teams tasked with executing this deployment are often stranded, looking to their peers at other organizations and hoping for a roadmap. Though these grassroots efforts vary, some best practices are emerging as more industries feel their way through the initial steps of deploying AI.
Moving forward, truly capitalizing on this groundbreaking technology will require mobilizing the right resources. Organizations that lack experience in this field can do so by finding the right partners: those that pair proven technical expertise with the broader solutions and capabilities needed to create tailored, domain-specific AI implementations that drive business outcomes.
The additional, more nuanced requirement is for leaders to enter AI projects with a growth mindset -- willing to accept AI and GenAI as experiments and learn through the course of project deployments. As organizations participate in the cross-industry buzz of GenAI, it’s important to stay focused rather than get too caught up in the hype. According to McKinsey, AI use at work is still in its early days with only 22% of global survey respondents saying they’re using GenAI regularly in their jobs -- despite many more of them having access to it!
Though companies will deploy AI in various ways, an effective strategy requires five crucial commitments:
Develop clear and measurable outcomes
Provide or find the required resources
Set boundaries to maintain focus on desired outcomes
Monitor data quality to produce the best results
Adhere to a strong security and compliance posture
Clarity on the Desired Outcome
Digital transformation accelerated the evolution of business strategy considerably over the last decade, and GenAI represents a significant inflection point that will propel us even faster. Due to labor market dynamics and the pace of digitization, IT has concurrently become a strategic resource rather than simply an enabling function. As such, IT departments have shifted from emphasizing delivery milestones to focusing on business metrics. Despite the fervent hype that AI has generated, organizations must maintain focus on these outcomes and desired use cases -- without becoming tempted to divert resources for fear of missing out. Now is the time to be pragmatic and take the finite steps needed to effectively utilize AI and attribute tangible outcomes that prove the value of the technology.
Required Resources and Talent
Proven AI expertise is a must-have in order to determine what specific resources are required to build and deploy successful AI. Organizations that lack the expertise need to work with partners that can provide it. Especially with GenAI, there is a tendency to treat AI initiatives as distinct from other IT projects and manage them in a silo. While some GenAI needs are distinct, they still tie back to existing resources, budgets, and talent. Determining those connection points is critical to staying as efficient as possible by maintaining that balance between leveraging existing resources and developing GenAI with the specialized attention it requires.
Setting Boundaries
AI can produce exponentially more data than previous digital investments, so well-defined boundaries are critical to keeping the focus on desired outcomes and managing hallucinations. The massive amount of data and metadata produced by AI provides a rich foundation on which organizations can build custom capabilities and experiences for employees, partners and customers alike -- albeit the needs of each of these three audiences are unique. The challenge is to ensure that these initiatives remain thoughtful and use-case specific, so the AI efforts do not become too fragmented or haphazard. For example, organizations can use AI to collect data points on hybrid work, determining an employee’s working habits and personalizing their digital experience accordingly. Once implemented, the tools and relevant insights could be shared with partners to better serve mutual end customers.
Data Quality
The adage of “garbage in equals garbage out” rings especially true with data. Without the proper attention to verification, AI can leave massive piles of useless mess -- not to mention storage and governance costs! Organizations must create data standards and build guardrails around their data, while allowing for some flexibility in how data users operate. In addition to standards and guardrails, there must be clarity of vision on what to do with the data in the first place. An organization’s data is its most valuable asset, and its value can only be realized if the data is actionable. So many organizations looking to leverage AI are producing massive amounts of data without the right guidance on what to do with it, rendering it impotent. This calls back to upfront resource considerations and the importance of selecting the right partners to provide expertise and fill in the gaps.
Security and Compliance
Finally, any successful AI deployment requires transparency and a degree of explainability to facilitate security measures and ensure data compliance. The nature of AI and its relationship to data can exacerbate security vulnerability, and our industry has seen threat actors use AI tools to find and exploit systems to launch malicious attacks. Like any technology, AI and GenAI can be used for good as well as evil, and they can result in security attacks that proliferate rapidly, making them orders of magnitude more damaging and difficult to identify and mitigate. Furthermore, these attacks can put increasing amounts of sensitive data at risk. Working with the right partner that understands compliance and security issues with AI -- and has a track record of effectively implementing policies to mitigate those issues -- is essential. The AI-powered applications and the data that feed them must be continuously monitored to protect an organization’s assets and to ensure AI success.
Sharing Our Successes
While best practices for AI deployments are still evolving, organizations must prioritize a few critical decisions to get started to maximize success rates and minimize the risk of falling behind competitors. The bottom line is that focusing on desired business outcomes keeps the expectation of an AI deployment consistent across an organization and gives it the best chance of becoming reality.
Let me know your organization’s biggest AI considerations in the comments, and let's keep building AI together and learning from each other.
About the Author(s)
You May Also Like