Can Generative AI and Data Quality Coexist?
While not exactly a marriage made in heaven, it’s still possible for AI and data quality to live happily together. The key is to focus on data quality.
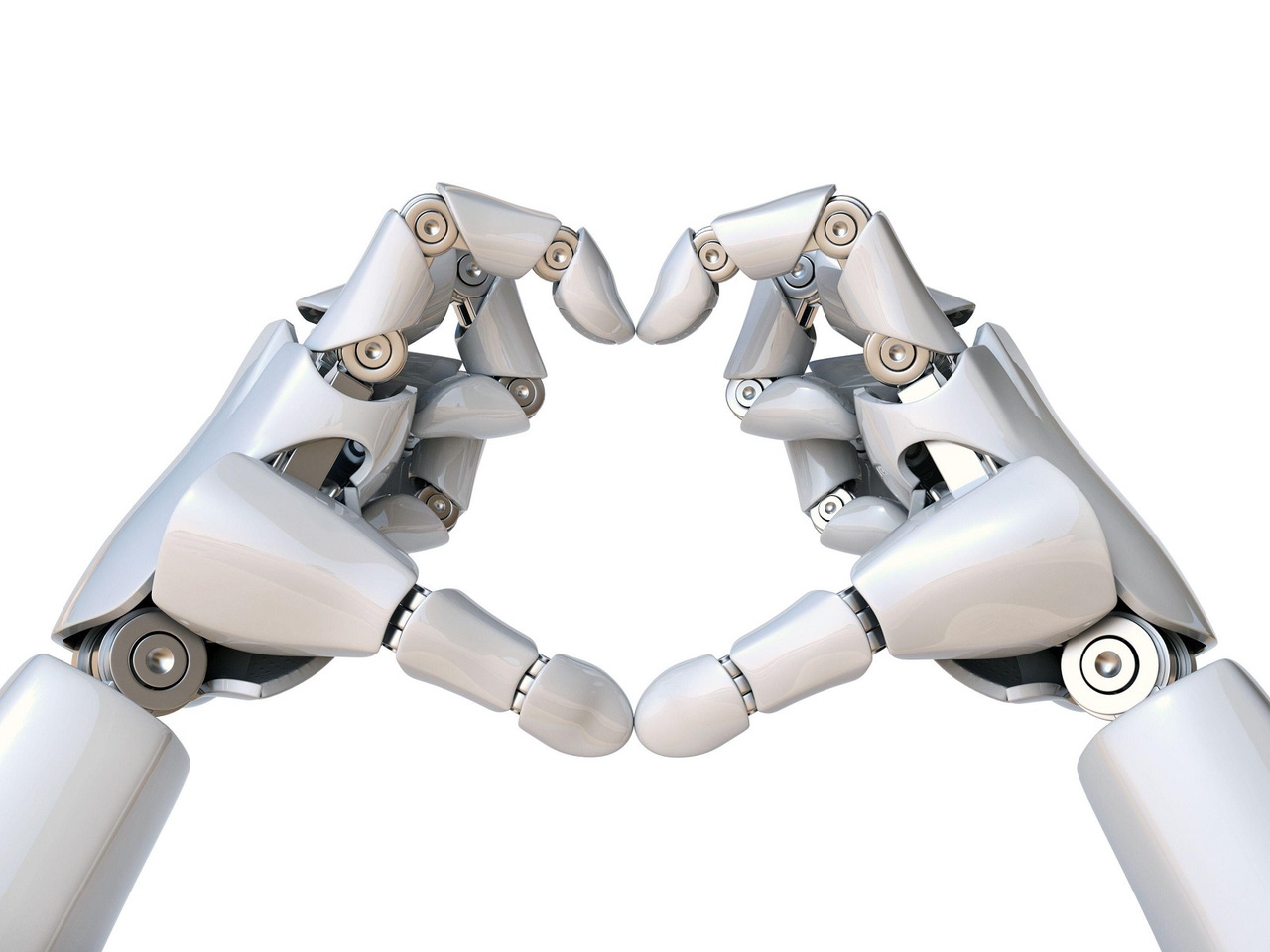
At a Glance
- Organizations will need to build both data quality and governance programs to prepare for generative AI adoption.
- GenAI models will be increasingly expected to produce outputs tailored to individual preferences or specific contexts.
- With stricter data privacy regulations there's a heightened need for compliant data management practices.
Despite rumors to the contrary, it really is possible for AI and data quality to cheerfully coexist.
Not only is it possible for generative AI and data quality to co-exist, it’s imperative that they do, says Marinela Profi, AI strategy advisor for analytics software developer SAS in an email interview. Data for AI is like food for humans, she notes. “Based on the quality of the food you feed your body and your brain, you will receive a certain quality of outputs, such as higher performance or more focus.”
Simply put, if you've neglected the quality of your enterprise data, or haven't defined a proper data strategy, you won't get value out of generative AI, Profi says. “On the flip side those who have implemented a strong data management discipline are uniquely positioned to gain a competitive advantage with generative AI.”
As the effectiveness of any AI system, including generative models, is largely dependent on the quality of its data, better data leads to more reliable and accurate AI outputs, observes Mayank Jindal, a software development engineer with Amazon via email.
Potential Barriers
People like to push the “easy button,” says Peter Marx, CTO at AgileView, which develops synthetic geospatial data for machine learning. “They look for simplified answers to complex, data driven problems,” he says via email. The barrier is rushing into decisions without completely understanding the basis of the statistical data being presented, whether planning a trip or military operations. “The desire to give people speed at the risk of not understanding the underlying data could be disastrous.”
Generative AI is not only reshaping the way humans interact with artificial intelligence for higher productivity, but it’s also influencing the requirements and challenges associated with data quality, Profi says. As large-scale model architectures, such as Generative Pre-trained Transformer (GPT) and DALL-E become larger and more complex, the demand for diverse, high-quality datasets increases. “These models require vast amounts of data to learn effectively, raising challenges in data curation and representation.”
New training techniques that require less data or that can learn more effectively from existing datasets might reduce the pressure on data quantity but increase the need for highly representative and unbiased data samples, Profi says. “Self-supervised and unsupervised learning techniques, in which models generate their own labels or learn from unlabeled data, reduce reliance on manually labeled datasets” she notes. “However, this increases the importance of having high-quality, diverse, and unbiased raw data, since the model's learning is directly based on the input data’s inherent characteristics.”
Generative AI is rapidly moving toward cross-domain applications, such as text-to-image generation and multimodal interactions combining text, image, and audio. “This evolution necessitates data that’s not only high-quality within each domain, but also accurately aligned and integrated across different modalities,” Profi says.
Seeking Quality
A proactive commitment to ensuring data quality is necessary to tackle quality challenges, Jindal says. “Since achieving perfect data quality initially is unlikely, ongoing monitoring for inaccuracies is crucial,” he advises. Such an approach allows for the continual updating and versioning of AI models based on new findings. “Creating domain-specific model versions can also help organizations manage resource allocation based on the criticality of the domain.”
In the years ahead, organizations will need to build both data quality and governance programs to prepare for generative AI adoption, says Brian Platz, CEO and co-founder of Web3 data company Fluree via email. “Organizations will begin to invest in integrating the IT, risk, and data functions of their enterprises to ensure that the way they're collecting, managing, and deploying data is done in a safe, compliant, and secure manner.” The integration of new privacy programs, tightly coupled with data governance transformation initiatives, will result in a comprehensive framework that safeguards data integrity while upholding stringent privacy standards. “We will see the data governance function work more closely with risk departments, IT and operations in order to build data-centric governance into AI programs and training sets.”
Ethical Challenges
In light of the continuing trend toward personalization, generative AI models will be increasingly expected to produce outputs tailored to individual preferences or specific contexts, Profi says. “This will require high-quality data that’s not only relevant, but that also respect privacy and ethical considerations.”
A growing emphasis on ethical AI development, including efforts to reduce biases in AI models, makes it imperative to have data quality checks targeted specifically toward bias detection and mitigation, Profi says. With stricter data privacy regulations, like GDPR and the AI EU Act, there's a heightened need for compliant data management practices, she notes. “Generative AI developers must ensure data quality while adhering to legal and ethical standards.”
About the Author(s)
You May Also Like