Data for Good: Tracking Legislative Influence
Analytics tools can be used to help citizens identify who are the real sponsors of pending legislative initiatives, saving people many, many hours of reading and research.
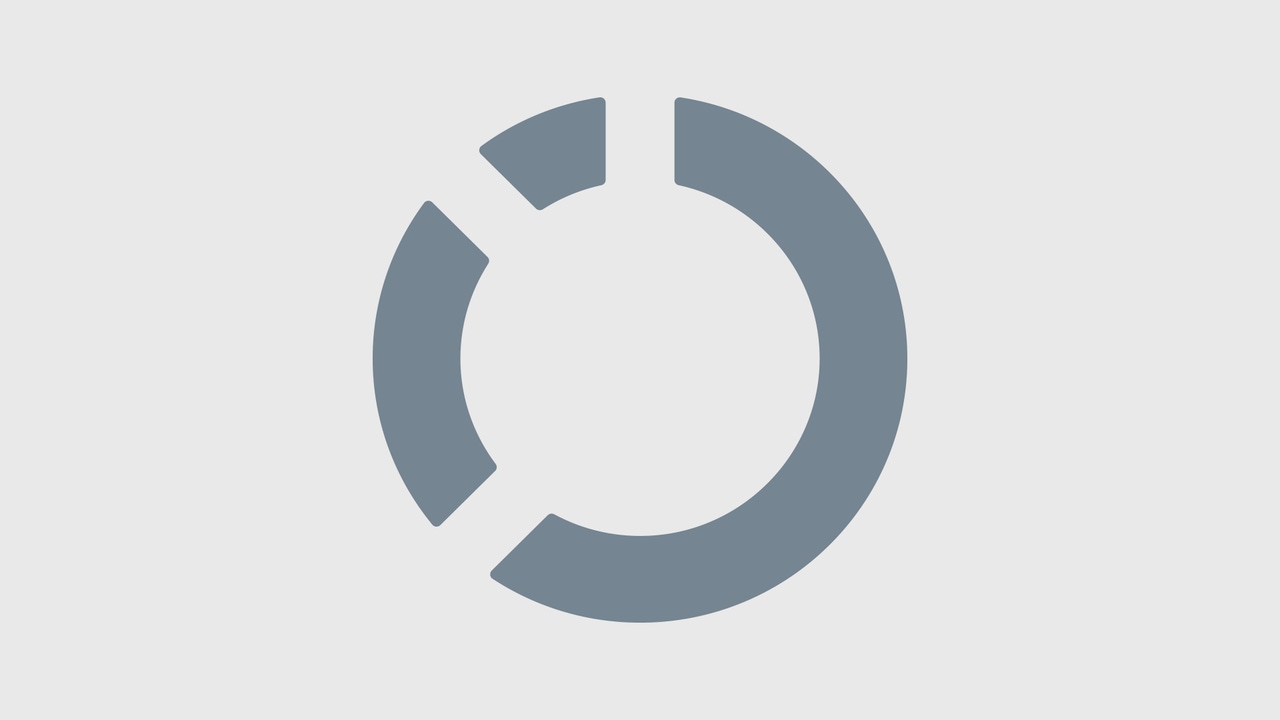
If you want to learn about the process of getting a proposed bill passed, you can read the official explanation on a state senate site. It’s remarkably similar to the steps involved for federal legislation, according to the explanation offered to the protagonist of Mr. Smith Goes to Washington. What the explanations don’t reveal, however, are the entities behind the proposed legislation.
The actual authors of proposed legislation don’t sign their names, but they do leave signatures of a sort, the signals of individual style that can be found throughout their written work. All it takes is reading through thousands of proposed bills to find the textual clues that link bills to the same source. The only drawback is coming up with the time it takes for humans to read through it all. But this is one problem that technology can solve.
One of the presentations featured at Bloomberg's Data for Good Exchange was on developing an approach to data mining the text to identify the sources behind the bills. Applying technology to sift through masses of documents that would take humans thousands of hours to read through is the project that a group of five has been working on together on at the University of Chicago's Data Science for Social Good Program.
Sifting through each piece of legislation to find matches is far too time-consuming, and relying on Google doesn’t cut it because its results are not confined to legislation and do not bring up complete documents. A more specialized tool is needed for the focus on state legislation, one they call the Legislative Influence Detector (LID). In just seconds, it can search through complete documents and will only report on matches within the legislation category.
Explaining their approach, the researchers pointed out that the Smith-Waterman local-alignment algorithm was too slow to sift through so many texts. So they start with Elasticsearch to calculate Lucene scores. That narrows the texts to work with down to 100. Those are the ones that get compared to the document in question through a local-alignment algorithm. As it maintains the sequence of words, it is much more precise and accurate than a bag-of-words model.
On the basis of the matches uncovered by LID, reporters or interested parties can track special interest influence through the trail set by the matches. To illustrate the point, they show a screenshot of LID finding similarities between the Wisconsin Senate Bill 179 (2015), restricting abortions after 19 weeks of gestation and the Louisiana Senate Bill 593 (2012). The wording the two used is almost the same. Both bills would reflect a conservative agenda, though the group doesn’t point out which particular special interest is behind them.
The LID group admits certain shortcomings of the solution, such as the fact that it’s limited to the bills collected by the Sunlight Foundation, although those alone top half a million bills. But what they don’t point to is the possibility of political bias in the selection of bills they focus on. They say they use “2,400 pieces of model legislation written by lobbyists” that is largely based on the collection of ALEC Exposed, the organization devoted to depicting the American Legislative Exchange Council (ALEC) as a bastion of corrupt corporate influence on legislation supported by the GOP.
ALEC Exposed is part of The Center for Media and Democracy (CMD), which calls itself “a national media group that conducts in-depth investigations into corruption and the undue influence of corporations on media and democracy.” While that makes it sound completely objective, it generally is characterized as “liberal,” even “uber-liberal,” “left-wing,” and “anti-capitalist". So it’s not at all surprising that it would target the conservative ALEC. That is not to say that the data is incorrect, but that transparency should really be free of political party influence. Tools like LID only are truly “data for good” if they apply the same standards to all parties.
About the Author(s)
You May Also Like