How Enterprises Can Scale their Machine Learning
Enterprise organizations are already seeing benefits from their machine learning practices, but Forrester Research says they've only scratched the surface.
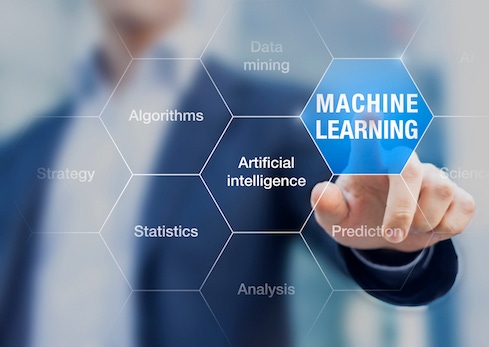
Enterprise organizations recognize the key functions that artificial intelligence and machine learning will play in their businesses in the future, and, depending on what survey you have read in the last year, they are investing to make that future a reality.
But what does it take to build a successful AI and machine learning practice? What does it take to go beyond building that practice and actually integrate AI and machine learning tools into the business itself where professionals across the organization touch it every day? How can we make these technologies which promise to provide a competitive edge into the lifeblood of the enterprise.
A couple of new Forrester Wave reports, which evaluate predictive analytics and machine learning tools, provide a blueprint for the successful AI-driven business of the future.
Forrester notes that many businesses already have such technologies in production. But we've just scratched the surface, and there's a long way to go before we begin to capture a fraction of the potential.
"Today, enterprises use machine learning models to identify customer churn, suggest upsell/cross-sell, reroute logistics bottlenecks, predict manufacturing machine failure, and make other predictions," Forrester analysts Mike Gualtieri and Kjell Carlsson wrote in the report, Forrester Wave: Multimodal Predictive Analytics and Machine Learning Solutions, Q3 2018. "
"A few models here and there are valuable and significant, but they are a mere drop in the bucket compared to what is possible," they wrote. "Enterprises have dozens, hundreds, and even thousands of applications and business processes that could, but do not currently, benefit from predictive models."
Forrester said that in 5 years it expects data science teams to be bigger than software development teams in enterprise organizations.
How does an enterprise begin to scale that effort and those benefits? Multimodal tools evaluated for the report are those that offer the breadth to reach across the enterprise, according to Forrester.
These are the tools that offer many different user interfaces, including graphical user interfaces, and a broad set of work bench tools. They can be used by data scientists, and they can also be used by "non-data scientists" to build data pipelines, create machine learning models, and collaborate with data science teams, Forrester said in the report.
Within enterprises, data science teams are small and spread out.
"To support the explosion of enterprise use cases, teams need to get bigger and, simultaneously, predictive analytics and machine learning tools need to support these teams as well as the larger community of business people, data engineers, software developers, and AI engineers."
Forrester predicts that "massive machine learning automation is the future of data science because it will make data science teams exponentially more productive." The report says that the CRISP-DM process is too sequential and too manually iterative to perform the job.
Tools to realize this future productivity will incorporate a few other elements as well. They need to integrate with software development and continuous integration tools. That's important for the AppDev teams to be able to use this work in their design, development and application deployment efforts. And second, these tools must keep up with open source innovations, such as deep learning, Forrester said. Vendors need to make new open source libraries and updated versions of existing libraries available and abstracted within their workbenches as soon as they are released. Currently, many teams are forced to use code-based solutions such as data science notebooks to get quick access to these open source libraries when they are released.
A second report, Forrester Wave: Notebook-Based Predictive Analytics and Machine Learning Solutions, Q3 2018, evaluates those data science notebook tools -- tools centered on coding in R, Python, or other languages, and using an open source Jupyter or other interface to make coding and managing projects easier.
Forrester is planning to release a third report next year that evaluates tools for automation-focused predictive analytics and machine learning.
For more about AI, machine learning, and analytics check out these recent articles.
AI & Machine Learning: An Enterprise Guide
10 Roadblocks to Becoming a Data-Driven Enterprise
Hire the Right Machine Learning Talent
About the Author(s)
You May Also Like